A Text-Independent Forced Alignment Method for Automatic Phoneme Segmentation.
AI(2022)
摘要
Phoneme segmentation is important for many healthcare applications, such as the diagnosis and monitoring of children with speech sound disorders (SSDs). This is usually addressed by performing forced alignment (FA), which essentially annotates an audio file to provide information on what has been uttered and where. While many FA tools exist, very few can work automatically without the assistance of a transcription. This work aims at providing a novel text-independent FA tool by using two models, namely wav2vec 2.0 and an unsupervised segmentor known as UnsupSeg. To provide labels to the segments, the class regions that are obtained by nearest-neighbour classification with wav2vec 2.0 labels pre-CTC collapse as the reference points. Maximal overlap between the class regions and the segments determines class label. Additional post-processing steps, such as over-fitting cleaning and application of voice activity detection, are also performed to further improve the segmentation performance. All the models used to create the tool are selfsupervised, and thus can leverage great amounts of unlabelled data to reduce the need for labelled data. When evaluated on the TIMIT dataset, our implementation achieved a harmonic mean score of 76.88%, competitive against other alternatives.
更多查看译文
关键词
segmentation,text-independent
AI 理解论文
溯源树
样例
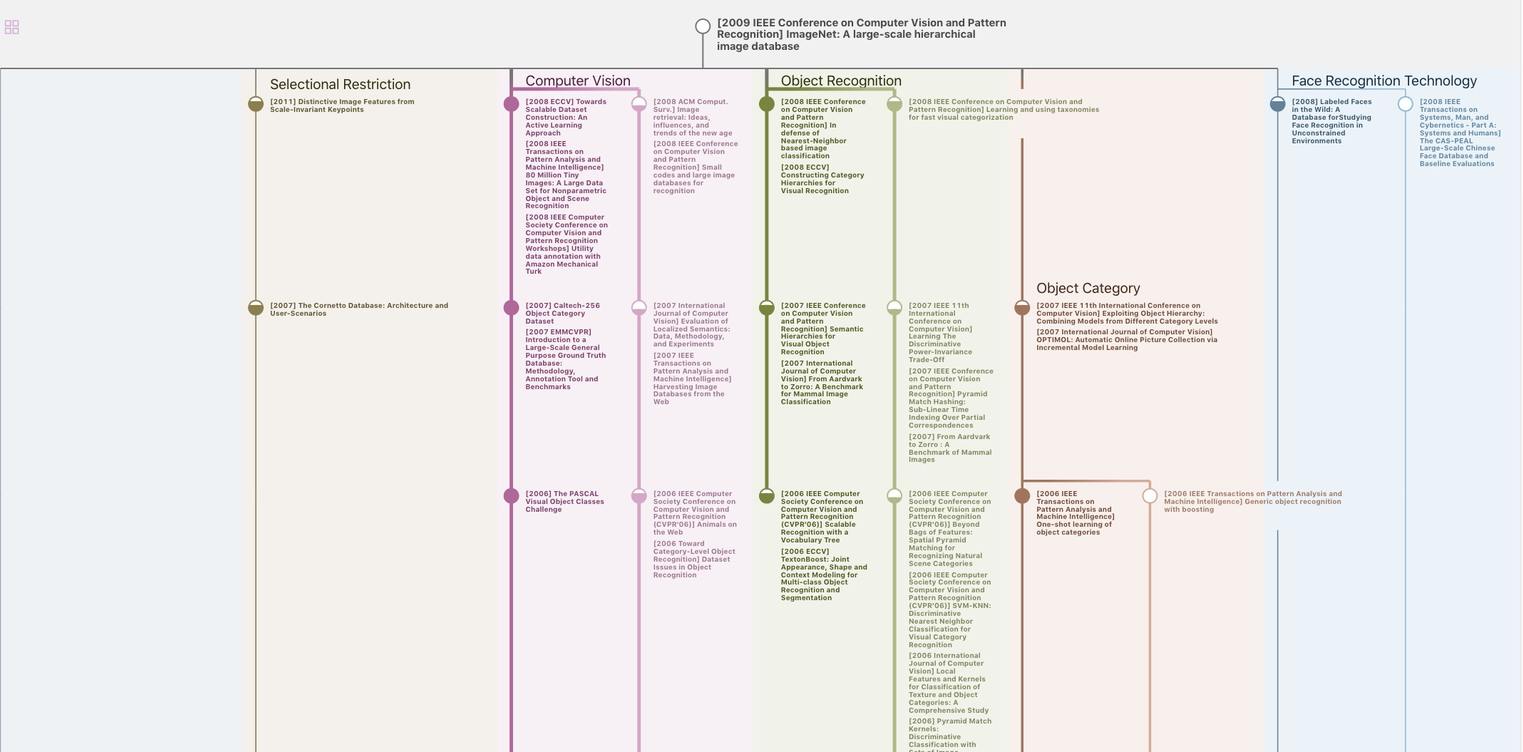
生成溯源树,研究论文发展脉络
Chat Paper
正在生成论文摘要