Generative Data Augmentation via Wasserstein Autoencoder for Text Classification.
ICTC(2022)
摘要
Generative latent variable models are commonly used in text generation and augmentation. However generative latent variable models such as the variational autoencoder(VAE) experience a posterior collapse problem ignoring learning for a subset of latent variables during training. In particular, this phenomenon frequently occurs when the VAE is applied to natural language processing, which may degrade the reconstruction performance. In this paper, we propose a data augmentation method based on the pre-trained language model (PLM) using the Wasserstein autoencoder (WAE) structure. The WAE was used to prevent a posterior collapse in the generative model, and the PLM was placed in the encoder and decoder to improve the augmentation performance. We evaluated the proposed method on seven benchmark datasets and proved the augmentation effect.
更多查看译文
关键词
Text augmentation,Generative model,Text classification
AI 理解论文
溯源树
样例
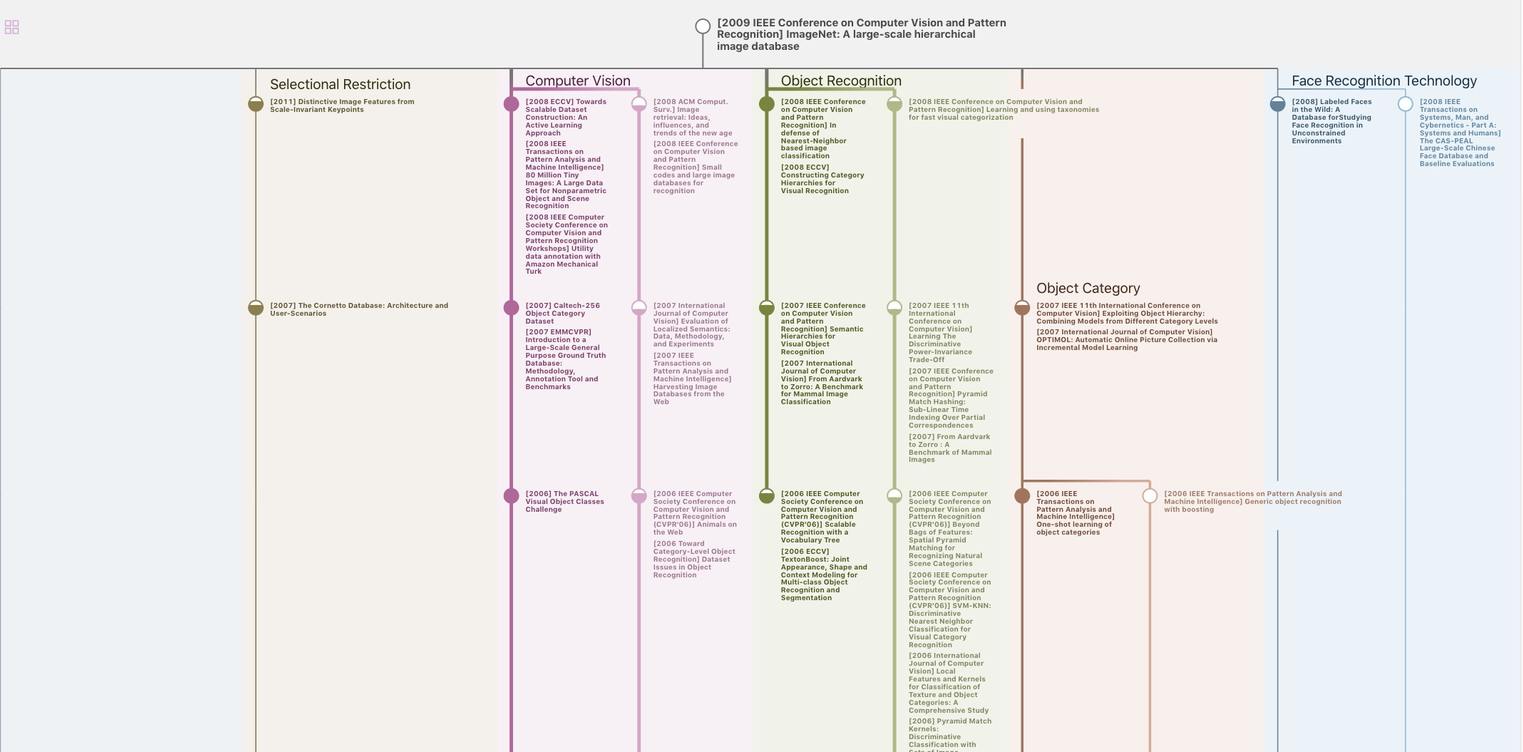
生成溯源树,研究论文发展脉络
Chat Paper
正在生成论文摘要