DRAGON: Deep Reinforcement Learning for Autonomous Grid Operation and Attack Detection.
ACSAC(2022)
摘要
As power grids have evolved, IT has become integral to maintaining reliable power. While providing operators improved situational awareness and the ability to rapidly respond to dynamic situations, IT concurrently increases the cyberattack threat surface - as recent grid attacks such as Blackenergy and Crashoverride illustrate. To defend against such attacks, modern power grids require a system that can maintain reliable power during attacks and detect when these attacks occur to allow for a timely response. To help address limitations of prior work, we propose DRAGON- deep reinforcement learning for autonomous grid operation and attack detection, which (i) autonomously learns how to maintain reliable power operations while (ii) simultaneously detecting cyberattacks. We implement DRAGON and evaluate its effectiveness by simulating different attack scenarios on the IEEE 14 bus power transmission system model. Our experimental results show that DRAGON can maintain safe grid operations 225.5% longer than a state-of-the-art autonomous grid operator. Furthermore, on average, our detection method reports a true positive rate of 92.9% and a false positive rate of 11.4%, while also reducing the false negative rate by 63.1% compared to a recent attack detection method.
更多查看译文
关键词
power grid reliability, cyberattack detection, deep reinforcement leearning
AI 理解论文
溯源树
样例
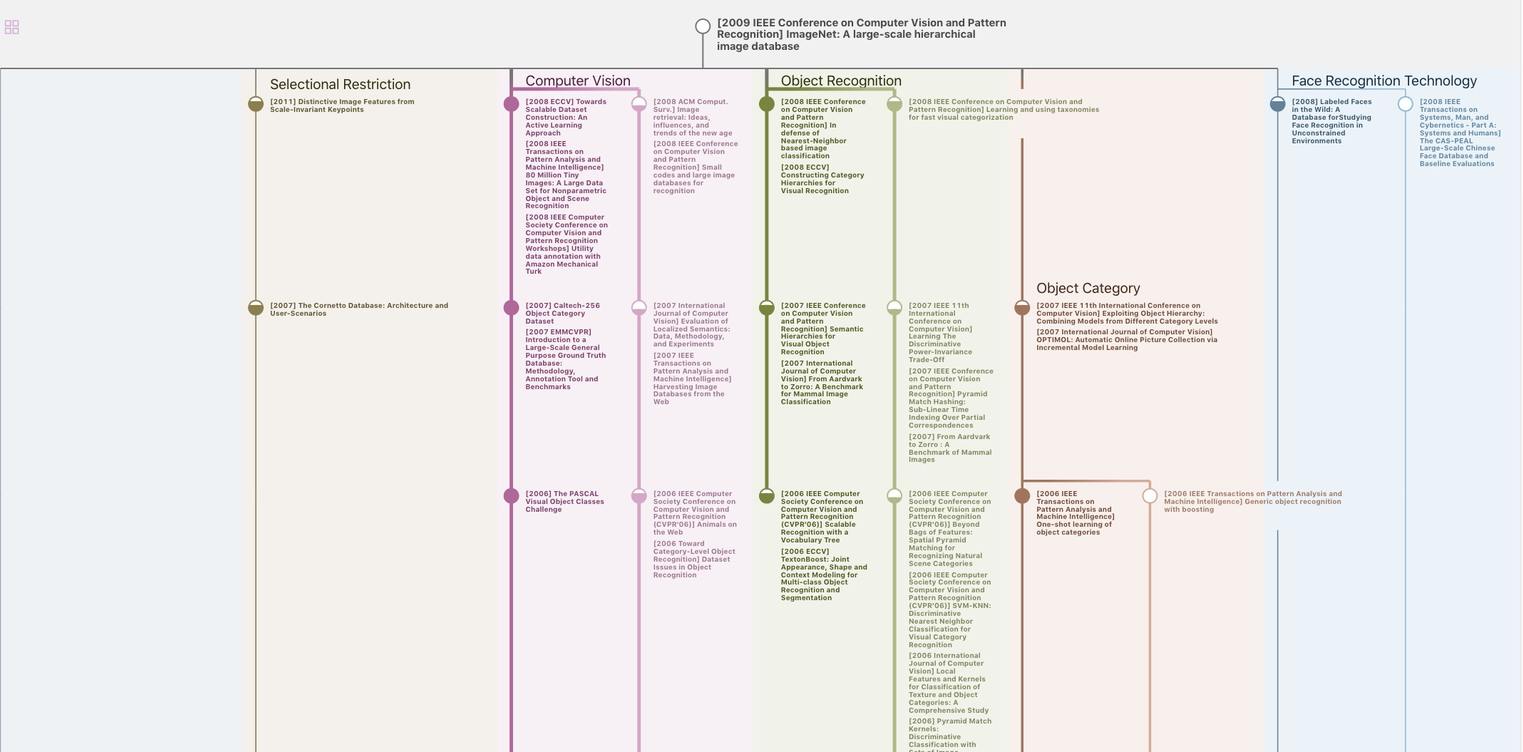
生成溯源树,研究论文发展脉络
Chat Paper
正在生成论文摘要