Energy-Efficient Intelligent Pulmonary Auscultation for Post COVID-19 Era Wearable Monitoring Enabled by Two-Stage Hybrid Neural Network.
ISCAS(2022)
摘要
This paper proposes an energy-efficient intelligent pulmonary auscultation system for post COVID-19 era wearable monitoring. This system consists of a tightly coupled two-stage hybrid neural network (TC-TSHNN) model and a corresponding multi-task training paradigm to improve prediction accuracy and generalization ability based on the fact that the number of COVID-19 patients is far less than that of normal people. At the first stage, two-category coarse classification is performed to identify normal and abnormal lung sounds. If the lung sound is abnormal, the second stage would be triggered to perform a four-category fine-grained classification. Besides, discrete wavelet transform is utilized for feature extraction, denoising and data reduction. In addition, advanced lightweight convolutional neural networks are used to reduce the model's computation and improve the model's performance. The hybrid network model can achieve 92% computation reduction and energy saving compared with a direct four-category classification when the input lung sound is normal, which is the majority of cases. Experiment results with inter-patient classification on the COVID-19 lung sound dataset from Tongji Hospital in Wuhan City and the ICBHI'17 dataset show that the proposed TC-TSHNN model can significantly reduce power consumption while maintaining competitive performance against the state-of-the-art work.
更多查看译文
关键词
wearable monitoring,energy-efficient,two-stage
AI 理解论文
溯源树
样例
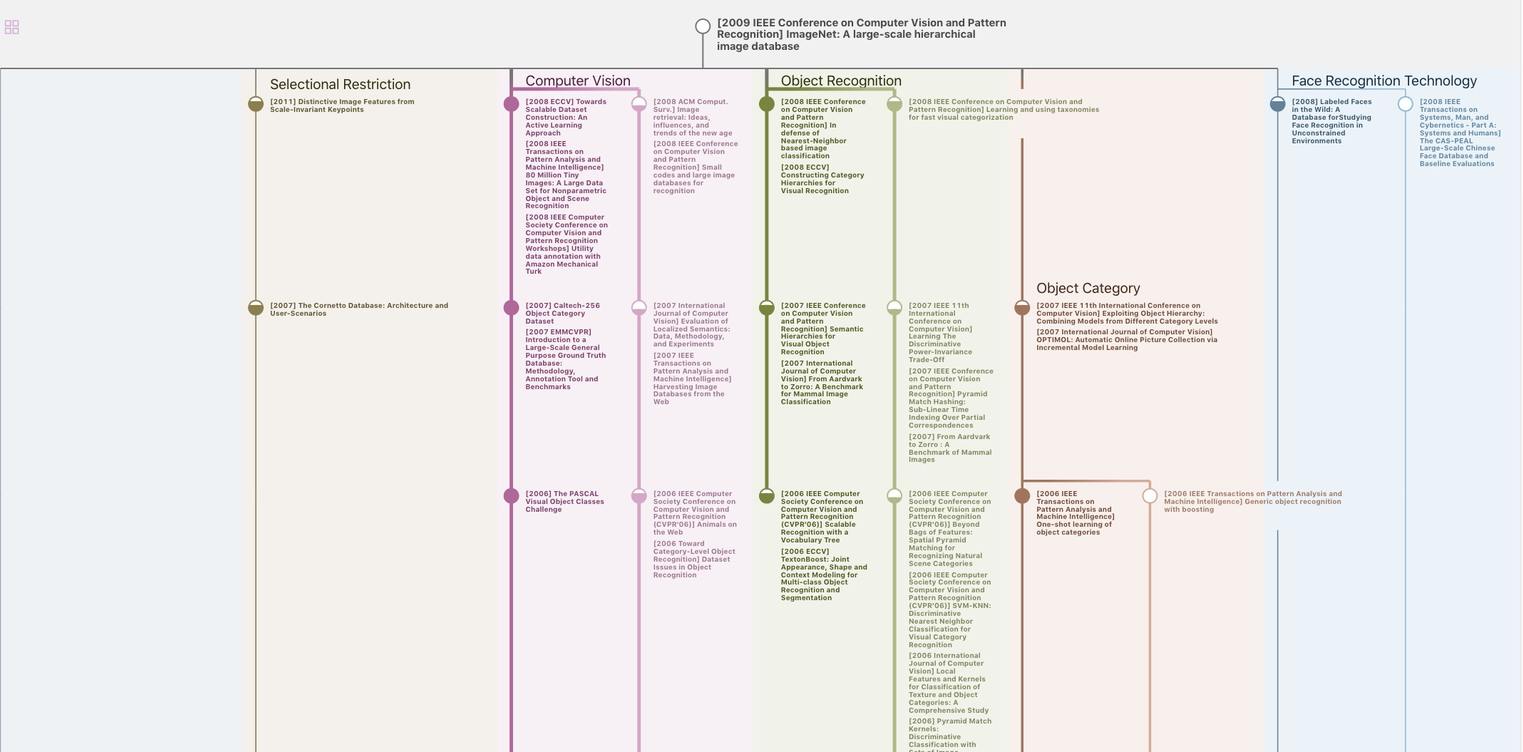
生成溯源树,研究论文发展脉络
Chat Paper
正在生成论文摘要