Graph Neural Networks for Idling Error Mitigation.
ICCAD(2022)
摘要
Dynamical Decoupling (DD)-based protocols have been shown to reduce the idling errors encountered in quantum circuits. However, the current research in suppressing idling qubit errors suffers from scalability issues due to the large number of tuning quantum circuits that should be executed first to find the locations of the DD sequences in the target quantum circuit, which boost the output state fidelity. This process becomes tedious as the size of the quantum circuit increases. To address this challenge, we propose a Graph Neural Network (GNN) framework, which mitigates idling errors through an efficient insertion of DD sequences into quantum circuits by modeling their impact at different idle qubit windows. Our paper targets maximizing the benefit of DD sequences using a limited number of tuning circuits. We propose to classify the idle qubit windows into critical and non-critical (benign) windows using a data-driven reliability model. Our results obtained from IBM Lagos quantum computer show that our proposed GNN models, which determine the locations of DD sequences in the quantum circuits, significantly improve the output state fidelity by a factor of 1.4x on average and up to 2.6x compared to the adaptive DD approach, which searches for the best locations of DD sequences at run-time.
更多查看译文
关键词
Quantum circuit,Decoherence errors,Noisy Intermediate-Scale Quantum (NISQ),Dynamical decoupling (DD),Machine learning,Graph Neural Network (GNN)
AI 理解论文
溯源树
样例
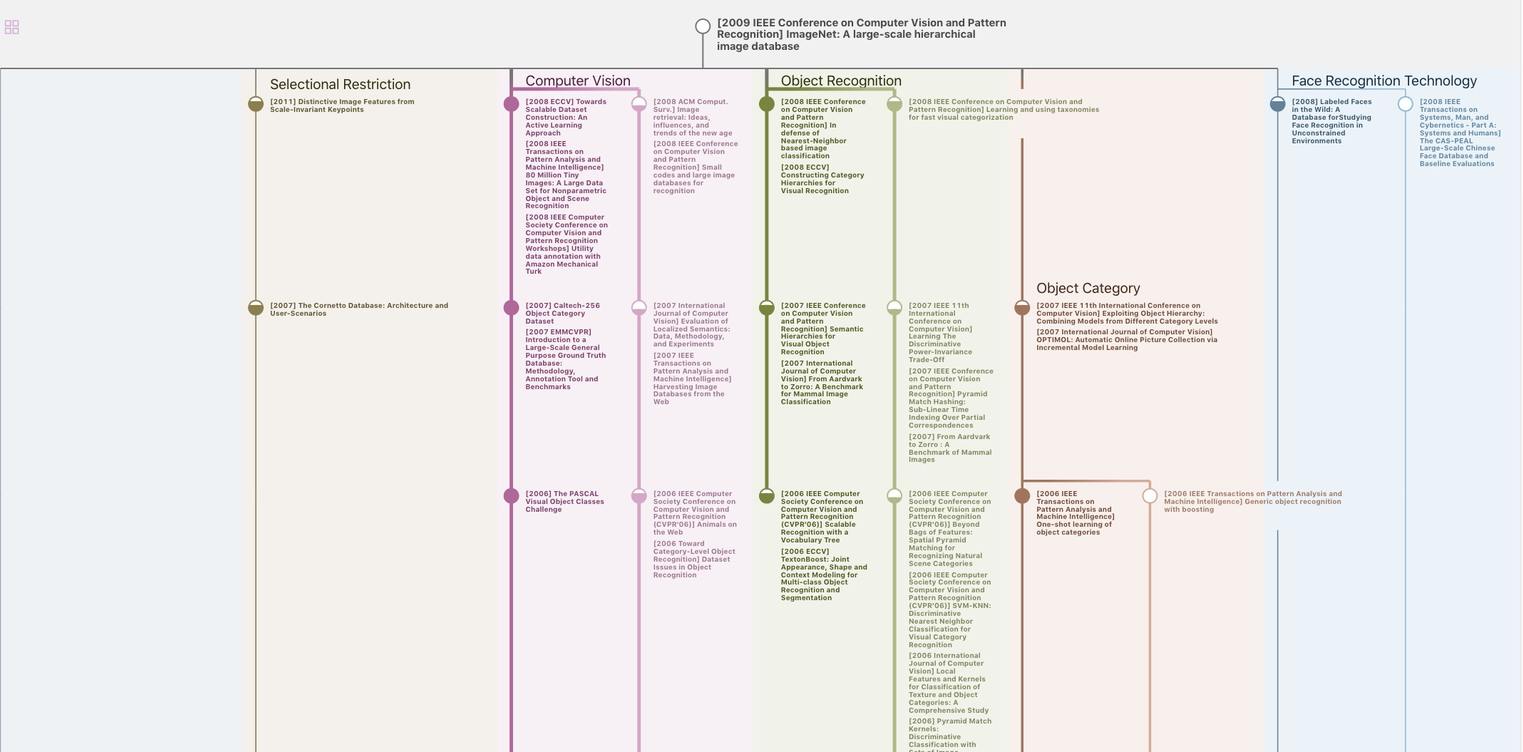
生成溯源树,研究论文发展脉络
Chat Paper
正在生成论文摘要