An Efficient Clustering-Based Privacy-Preserving Recommender System.
NSS(2022)
摘要
Recommender systems help online services deliver personalised content and facilitate information overload. Collaborative Filtering is a commonly used technique for building recommender systems. It analyses data collected from users in order to predict their preferences, this causes concerns over the privacy and security of the data as conventional techniques do not prioritise data privacy. Incorporating cryptography into recommender systems resolves the privacy issues, and yet it is impractical in many cases due to the computational overhead imposed by the cryptography and the sheer amount of data needed for computations. In this paper, we propose an efficient clustering-based privacy-preserving recommender system. The system employs homomorphic encryption for protecting user data while retaining the ability to generate recommendations using Collaborative Filtering. To facilitate information overload, a secure clustering technique is adopted to partition data prior to generating recommendations. The proposed system is secure under the semi-honest adversary model. Experiments show that the proposed system is efficient, scalable and produces accurate recommendations.
更多查看译文
关键词
clustering-based,privacy-preserving
AI 理解论文
溯源树
样例
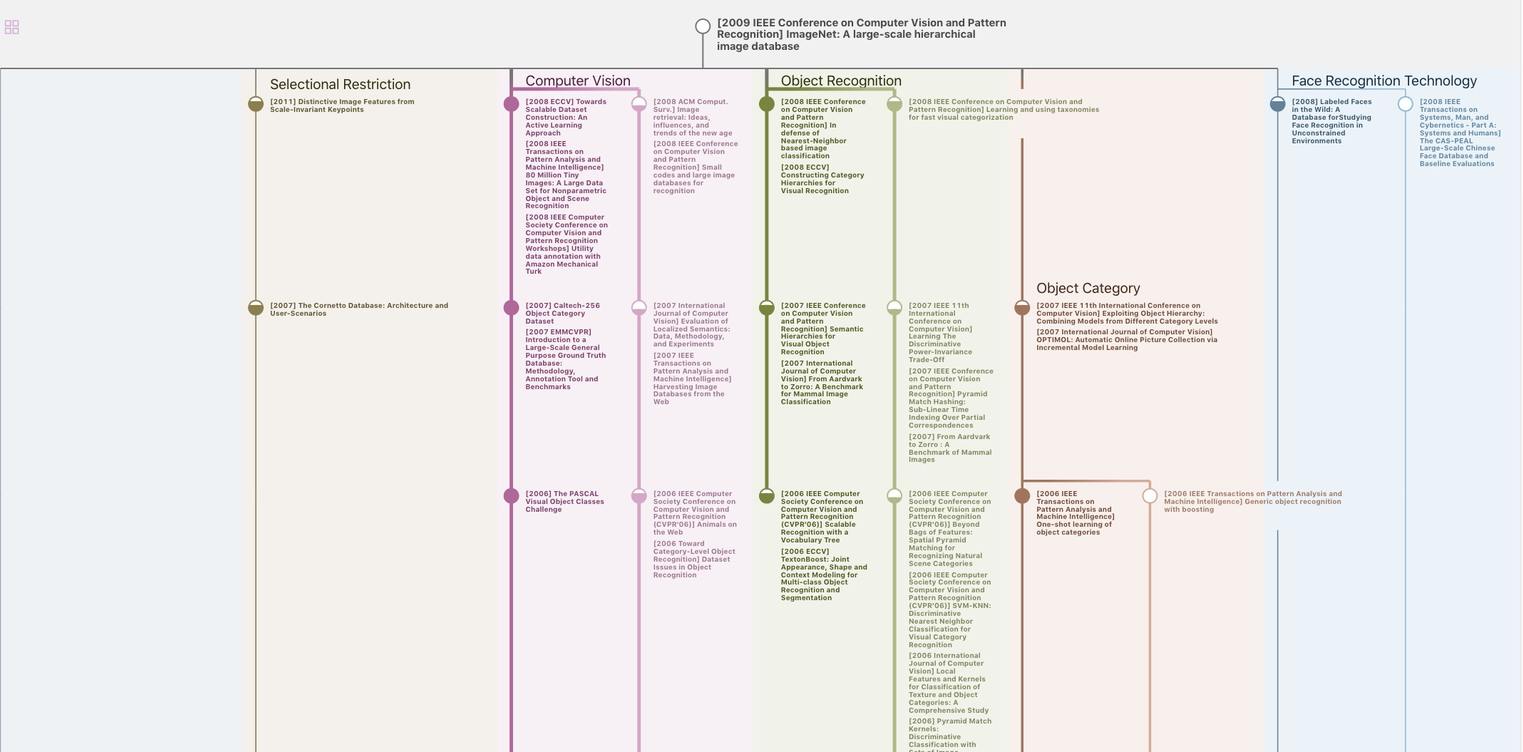
生成溯源树,研究论文发展脉络
Chat Paper
正在生成论文摘要