A Vision Transformer Based Scene Text Recognizer with Multi-grained Encoding and Decoding.
ICFHR(2022)
摘要
Recently, vision Transformer (ViT) has attracted more and more attention, many works introduce the ViT into concrete vision tasks and achieve impressive performance. However, there are only a few works focused on the applications of the ViT for scene text recognition. This paper takes a further step and proposes a strong scene text recognizer with a fully ViT-based architecture. Specifically, we introduce multi-grained features into both the encoder and decoder. For the encoder, we adopt a two-stage ViT with different grained patches, where the first stage extracts extent visual features with 2D fine-grained patches and the second stage aims at the sequence of contextual features with 1D coarse-grained patches. The decoder integrates Connectionist Temporal Classification (CTC)-based and attention-based decoding, where the two decoding schemes introduce different grained features into the decoder and benefit from each other with a deep interaction. To improve the extraction of fine-grained features, we additionally explore self-supervised learning for text recognition with masked autoencoders. Furthermore, a focusing mechanism is proposed to let the model target the pixel reconstruction of the text area. Our proposed method achieves state-of-the-art or comparable accuracies on benchmarks of scene text recognition with a faster inference speed and nearly 50% reduction of parameters compared with other recent works.
更多查看译文
关键词
decoding,text,encoding,transformer,multi-grained
AI 理解论文
溯源树
样例
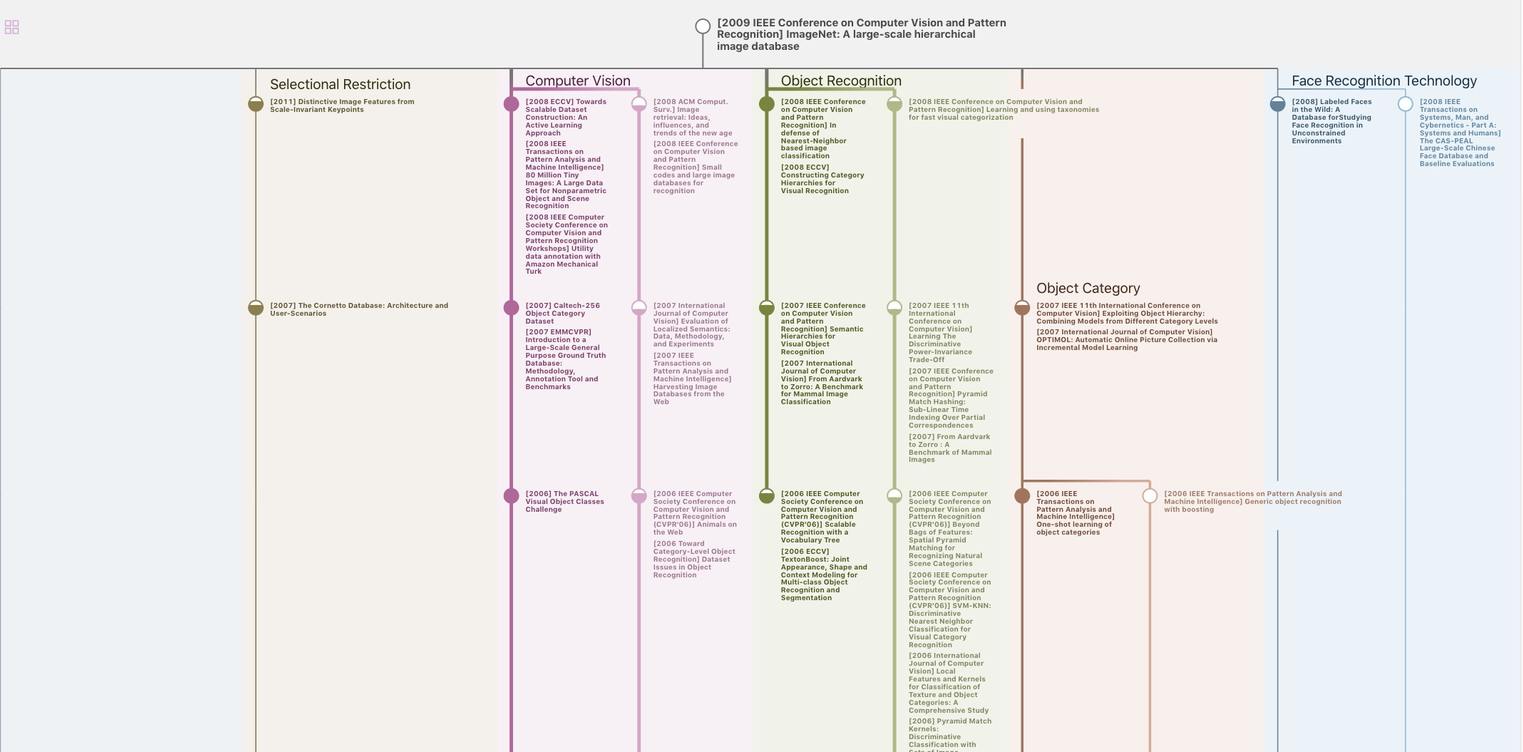
生成溯源树,研究论文发展脉络
Chat Paper
正在生成论文摘要