Computational screening and machine learning of hydrophobic metal-organic frameworks for removal of chemical warfare agents from air
Applied Materials Today(2023)
摘要
The removal of chemical warfare agents from humid air is particularly important for the battlefield and warfare agent leakage scenarios. In this work, the capture efficiency of hydrophobic Computation-Ready, Experimentalmetal-organic frameworks (CORE-MOFs) toward four warfare agents (mustard gas (HD), 2-chloroethyl ethyl sulfide (2-CEES), Soman, and Dimethyl methanephosphonate (DMMP)) in air were obtained by combining highthroughput computational screening (HTCS) and machine learning (ML). First, the relationships between the structures and performance indicators (adsorption capacity (N), selectivity (S), and comprehensive trade-off between S and N (TSN)) of the MOFs were established through HTCS. After comparison, there was a strong correlation between the porosity, Henry's coefficient (KH), and the capture performance. Then, three machine learning algorithms, decision tree (DT), random forest (RF), and extreme gradient boosting (XGB), were used to predict the performances of the MOFs. Compared with N and S, TSN had a better prediction effect (average correlation coefficient (R) > 0.95) for the four warfare agents, and the XGB model was suitable for the prediction of the MOF-warfare agent system behavior. KH had the highest relative importance based on big data analysis. Finally, based on TSN, 10 MOFs with the highest performances were screened for the adsorption of four warfare agents, showing that MOF materials with open metal sites had excellent adsorption performances toward the four warfare agents. HTCS and ML were used to understand the relationships between the MOFs and four warfare agents from different perspectives, which provides a new direction for the efficient screening of MOFs to capture these four warfare agents in air.
更多查看译文
关键词
Warfare agents,Metal -organic framework,Adsorption,Machine learning
AI 理解论文
溯源树
样例
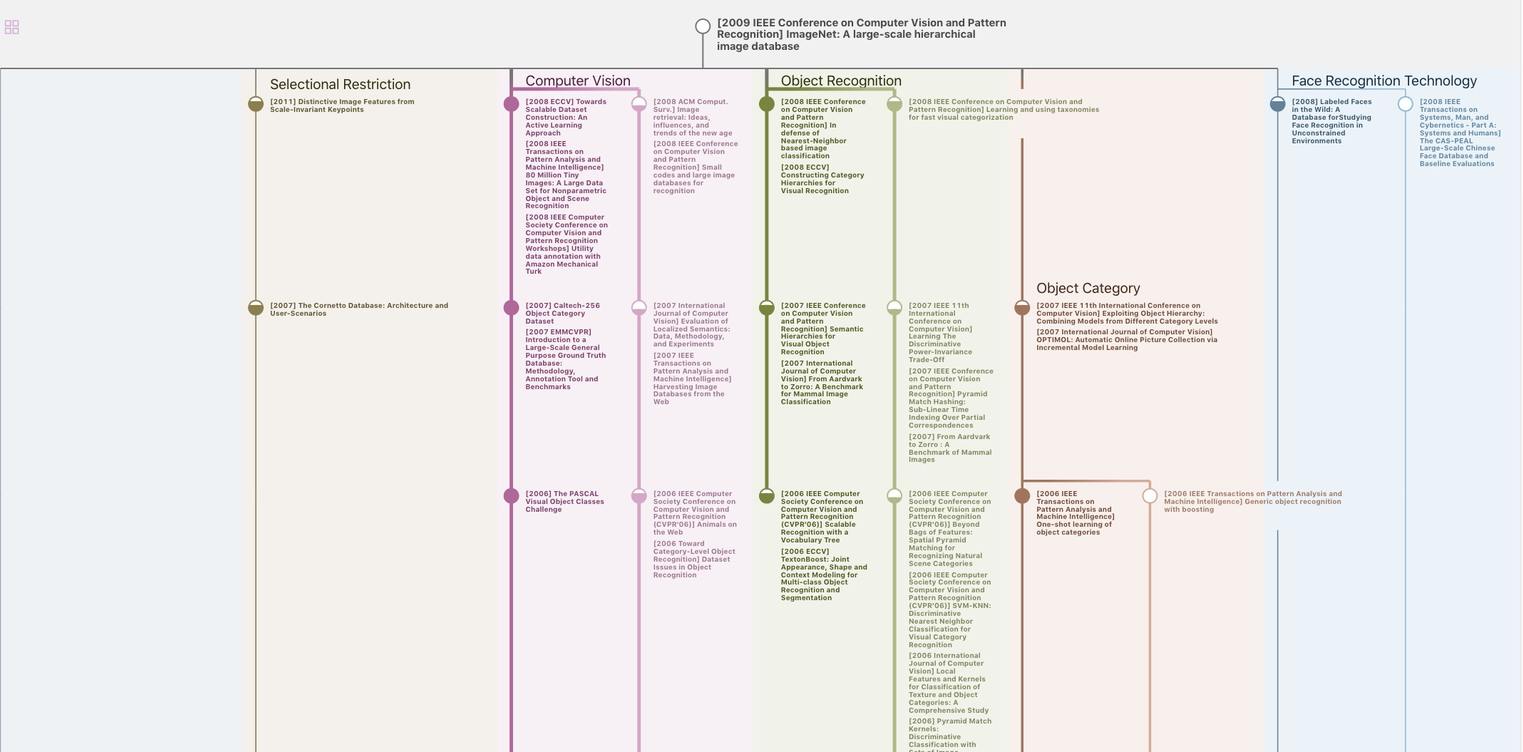
生成溯源树,研究论文发展脉络
Chat Paper
正在生成论文摘要