Question-Attentive Review-Level Recommendation Explanation
2022 IEEE International Conference on Big Data (Big Data)(2022)
摘要
Recommendation explanations help to improve their acceptance by end users. The form of explanation of interest here is presenting an existing review of the recommended item. The challenge is in selecting a suitable review, which is customarily addressed by assessing the relative importance of each review to the recommendation objective. Our focus is on improving review-level explanation by leveraging additional information in the form of questions and answers (QA). The proposed framework employs QA in an attention mechanism that aligns reviews to various QAs of an item and assesses their contribution jointly to the recommendation objective. The benefits are two-fold. For one, QA aids in selecting more useful reviews. For another, QA itself could accompany a well-aligned review in an expanded form of explanation. Experiments showcase the efficacies of our method as compared to baselines in identifying useful reviews and QAs, while maintaining parity in recommendation performance.
更多查看译文
关键词
question-attentive,review-level
AI 理解论文
溯源树
样例
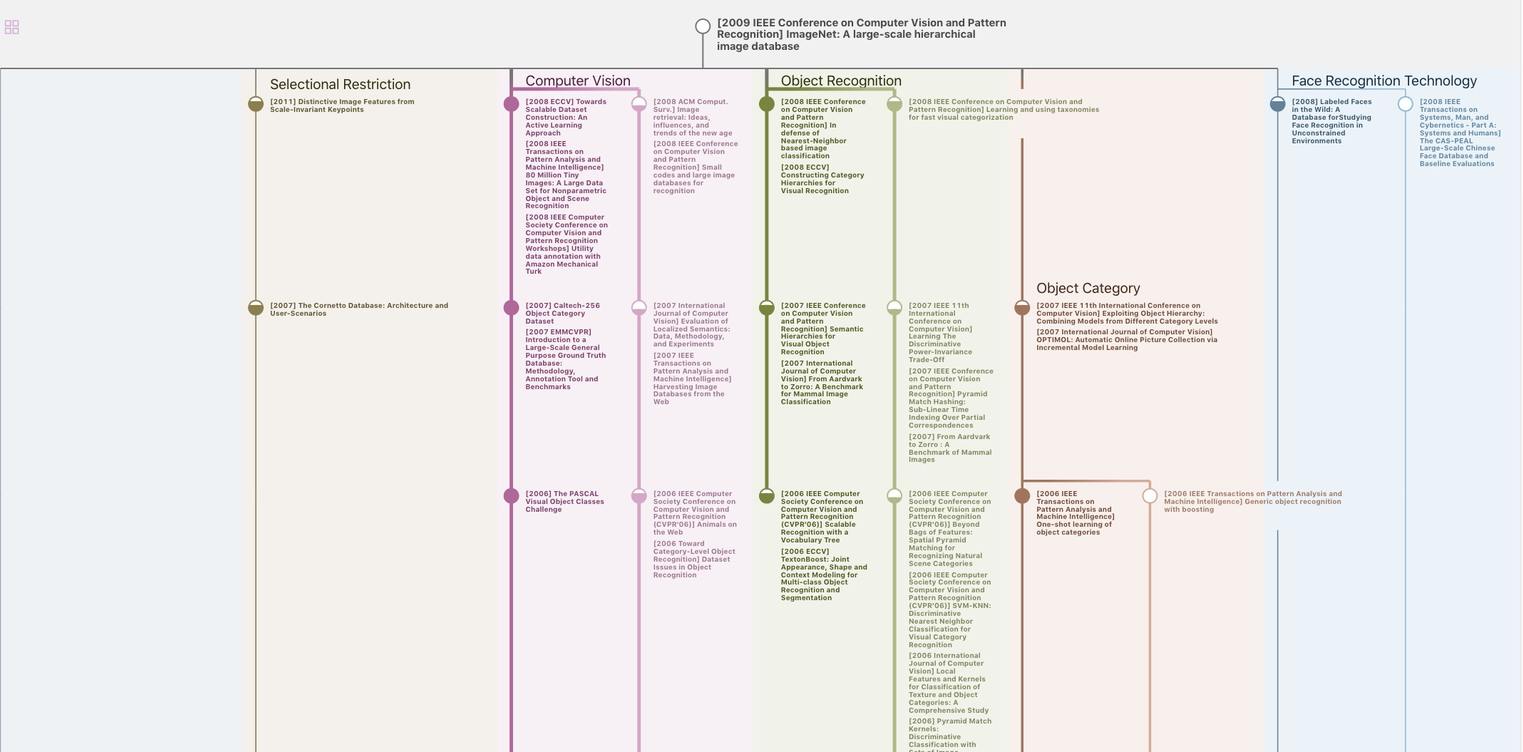
生成溯源树,研究论文发展脉络
Chat Paper
正在生成论文摘要