ClusterST: Clustering Spatial–Temporal Network for Traffic Forecasting
IEEE Transactions on Intelligent Transportation Systems(2023)
摘要
Traffic forecasting aims to capture complex spatial-temporal dependencies and non-linear dynamics, which plays an indispensable role in intelligent transportation systems and other domains like neuroscience, climate, etc. Most recent works rely on graph convolutional networks (GCN) to model the dependencies and the dynamics. However, the over-smoothing issue of GCN would produce indistinguishable features among nodes, leading to poor expressivity and weak capability of modeling complex dependencies and dynamics. To address this issue, we present a novel clustering spatial-temporal (ClusterST) unit, which incorporates unsupervised learning into GCN for extracting discriminative features. Specifically, we first exploit a neural network to learn a dynamic clustering, i.e., learning to partition the neighbors of each node into clusters at each time step. Two probabilistic losses are proposed to improve the separability of clusters. Then, the extracted features of different clusters can be distinguished. Based on the dynamically formed clusters, a vanilla GCN is applied to aggregate features within each cluster. By purely exploiting such a ClusterST unit, large improvements over the state-of-the-art are achieved. Furthermore, ClusterST units with a different number of clusters can be regarded as basic components to construct an inception-like ClusterST network for going deeper. We evaluate the framework on two real-world large-scale traffic datasets and observe an average improvement of 18.19% and 7.62% over state-of-the-art baselines, respectively. The code and models will be publicly available.
更多查看译文
关键词
Traffic forecasting,graph convolutional network,spatial-temporal networks,over-smoothing
AI 理解论文
溯源树
样例
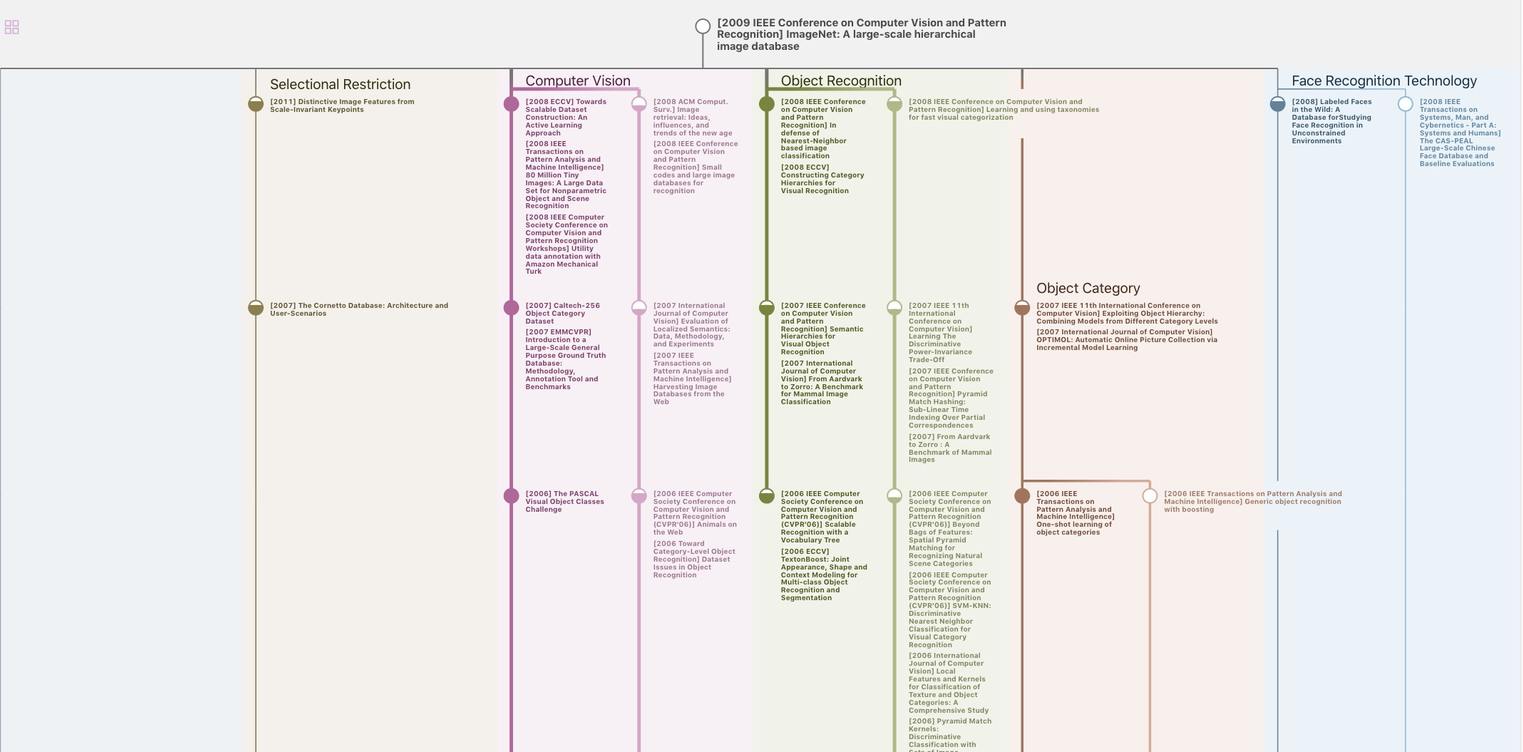
生成溯源树,研究论文发展脉络
Chat Paper
正在生成论文摘要