Re-embedding data to strengthen recovery guarantees of clustering
arxiv(2023)
摘要
We propose a clustering method that involves chaining four known techniques into a pipeline yielding an algorithm with stronger recovery guarantees than any of the four components separately. Given $n$ points in $\mathbb R^d$, the first component of our pipeline, which we call leapfrog distances, is reminiscent of density-based clustering, yielding an $n\times n$ distance matrix. The leapfrog distances are then translated to new embeddings using multidimensional scaling and spectral methods, two other known techniques, yielding new embeddings of the $n$ points in $\mathbb R^{d'}$, where $d'$ satisfies $d'\ll d$ in general. Finally, sum-of-norms (SON) clustering is applied to the re-embedded points. Although the fourth step (SON clustering) can in principle be replaced by any other clustering method, our focus is on provable guarantees of recovery of underlying structure. Therefore, we establish that the re-embedding improves recovery SON clustering, since SON clustering is a well-studied method that already has provable guarantees.
更多查看译文
关键词
clustering,recovery guarantees,re-embedding
AI 理解论文
溯源树
样例
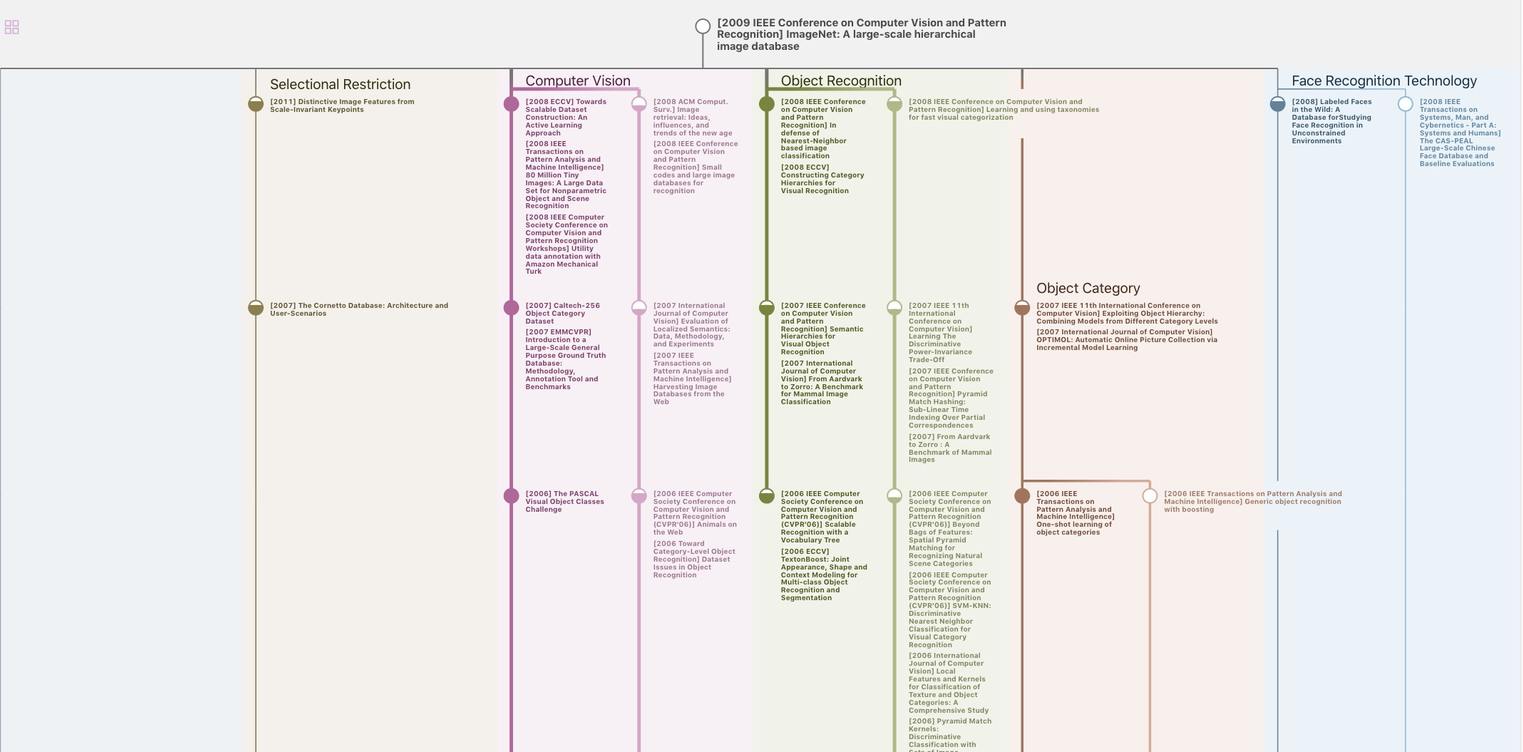
生成溯源树,研究论文发展脉络
Chat Paper
正在生成论文摘要