Machine learning using structural representations for discovery of high temperature superconductors
arxiv(2023)
摘要
The expansiveness of compositional phase space is too vast to fully search using current theoretical tools for many emergent problems in condensed matter physics. The reliance on a deep chemical understanding is one method to identify local minima of relevance to investigate further, minimizing sample space. Utilizing machine learning methods can permit a deeper appreciation of correlations in higher order parameter space and be trained to behave as a predictive tool in the exploration of new materials. We have applied this approach in our search for new high temperature superconductors by incorporating models which can differentiate structural polymorphisms, in a pressure landscape, a critical component for understanding high temperature superconductivity. Our development of a representation for machine learning superconductivity with structural properties allows fast predictions of superconducting transition temperatures ($T_c$) providing a $r^2$ above 0.94.
更多查看译文
关键词
high
AI 理解论文
溯源树
样例
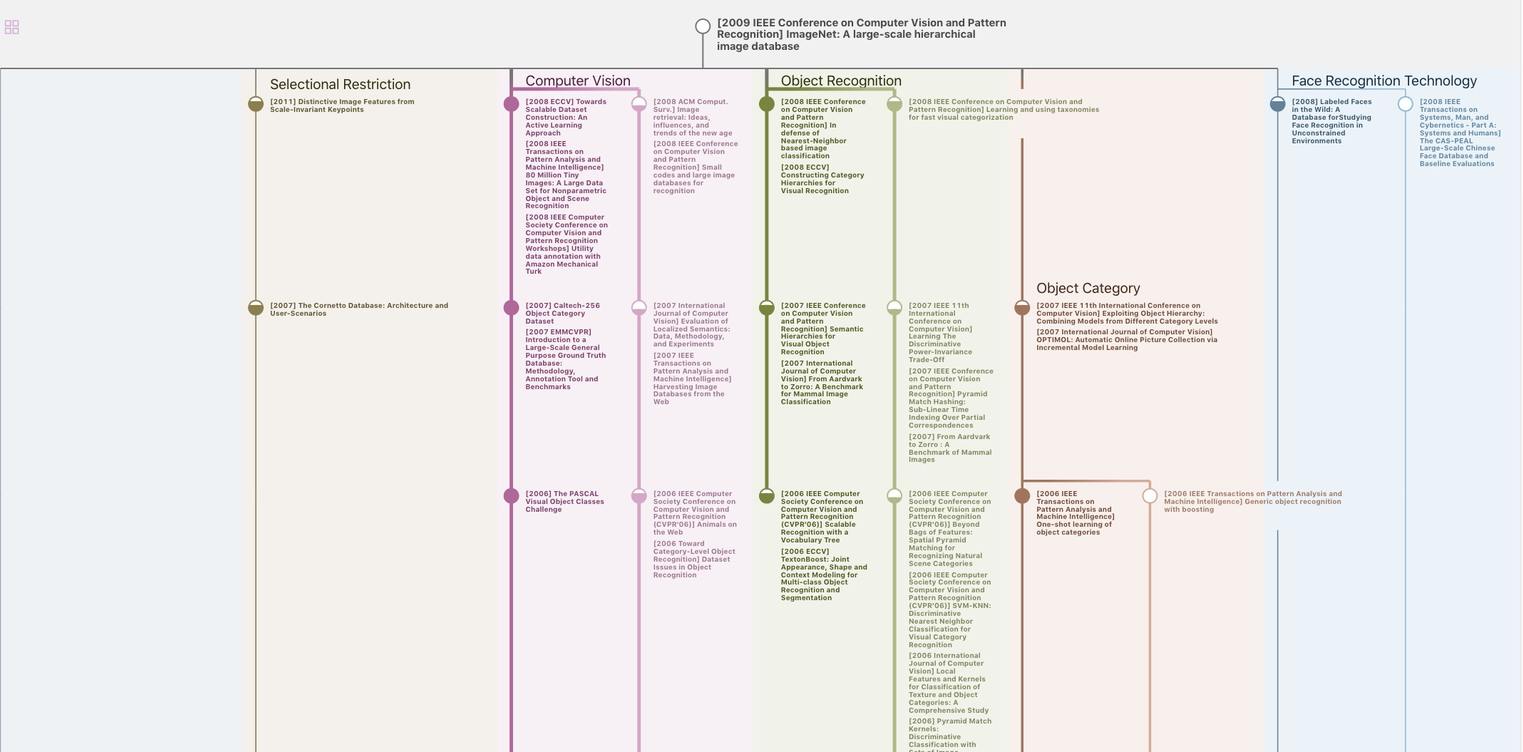
生成溯源树,研究论文发展脉络
Chat Paper
正在生成论文摘要