A Stacking-Based Ensemble Learning Method for Available Nitrogen Soil Prediction with a Handheld Micronear-Infrared Spectrometer
Journal of Applied Spectroscopy(2023)
摘要
Soil-available nitrogen is a vital index related to the growth and development of crops. The real-time and nondestructive detection of the soil-available nitrogen content based on near-infrared (NIR) spectroscopy could improve the accurate management of crop nutrients. In this manuscript, soil NIR spectroscopy and available nitrogen data are used in a stacked framework to develop a reliable and accurate soil-available nitrogen model. The spectral reflectance of the soil samples was collected in the 900 to 1700 nm band with nine pre-processing methods using a handheld micronear-infrared spectrometer. The stacking framework of this manuscript has two layers. Extreme gradient boosting (XGBoost), categorical boosting (CatBoost), a light gradient boosting machine (LightGBM), and a random forest, which are tree-based algorithms, are stacked as base models in the first layer. In the second layer, linear regression is employed in a meta-model to identify the unique learning pattern of the base model. The results show that the range and characteristics of the spectra can be used to make relevant predictions , and the micro-NIR spectra are variable under different pre-treatments. In addition, the stacked model achieves the best performance of all the models tested. Notably, the coefficient of determination (R 2 ) is 0.942, and the relative percent difference is 4.192 with Savitzky–Golay and multiplicative scatter correction. This manuscript presents an efficient method for predicting soil-available nitrogen levels with a handheld micronear-infrared spectrometer.
更多查看译文
关键词
Near-infrared spectrum,Soil-available nitrogen,Handheld micronear-infrared spectrometer,Pretreatment,Stacked ensemble learning method
AI 理解论文
溯源树
样例
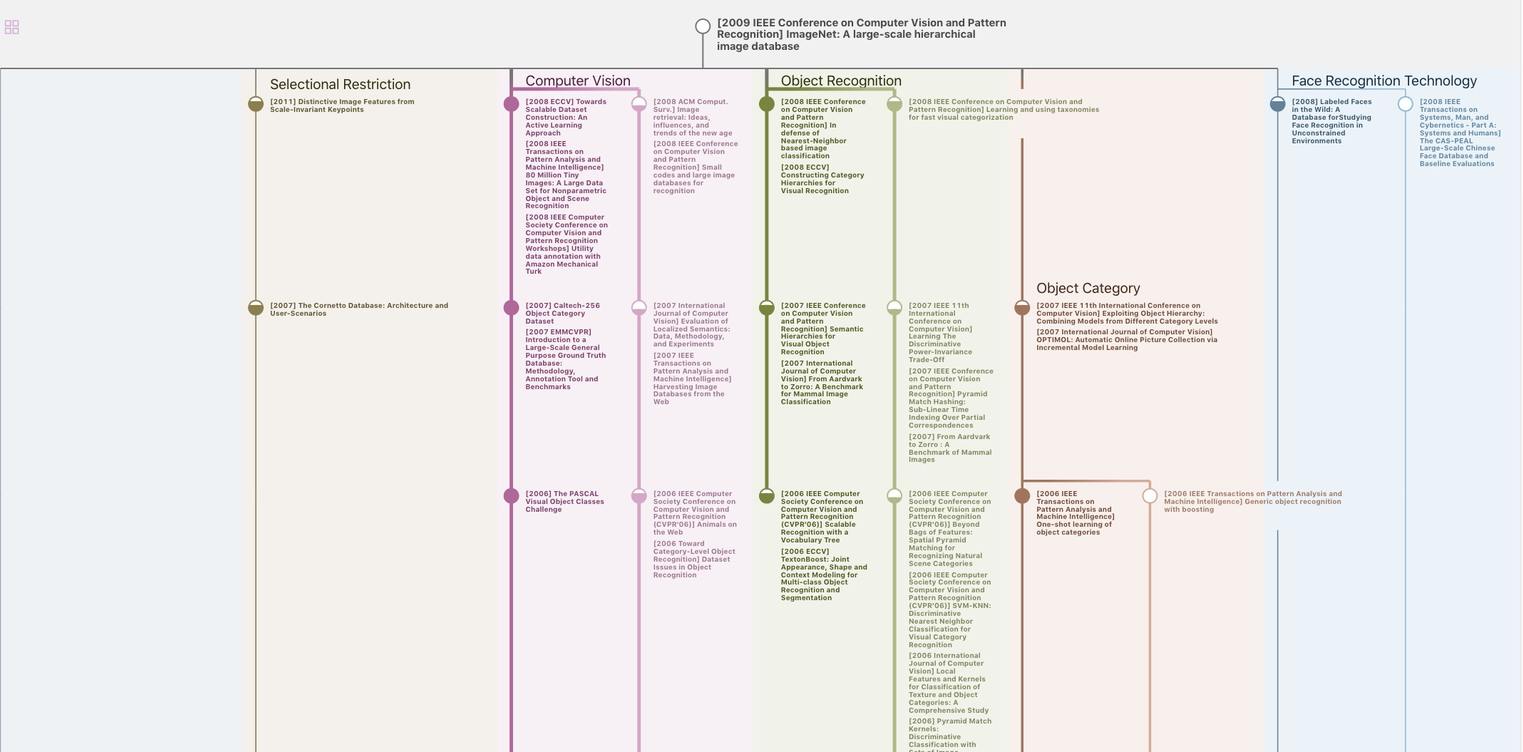
生成溯源树,研究论文发展脉络
Chat Paper
正在生成论文摘要