Calibrating Simulation Models with Sparse Data: Counterfeit Supply Chains During Covid-19
2022 Winter Simulation Conference (WSC)(2022)
摘要
COVID-19 related crimes like counterfeit Personal Protective Equipment (PPE) involve complex supply chains with partly unobservable behavior and sparse data, making it challenging to construct a reliable simulation model. Model calibration can help with this, as it is the process of tuning and estimating the model parameters with observed data of the system. A subset of model calibration techniques seems to be able to deal with sparse data in other fields: Genetic Algorithms and Bayesian Inference. However, it is unknown how these techniques perform when accurately calibrating simulation models with sparse data. This research analyzes the quality-of-fit of these two model calibration techniques for a counterfeit PPE simulation model given an increasing degree of data sparseness. The results demonstrate that these techniques are suitable for calibrating a linear supply chain model with randomly missing values. Further research should focus on other techniques, larger set of models, and structural uncertainty.
更多查看译文
关键词
counterfeit supply chains,simulation models
AI 理解论文
溯源树
样例
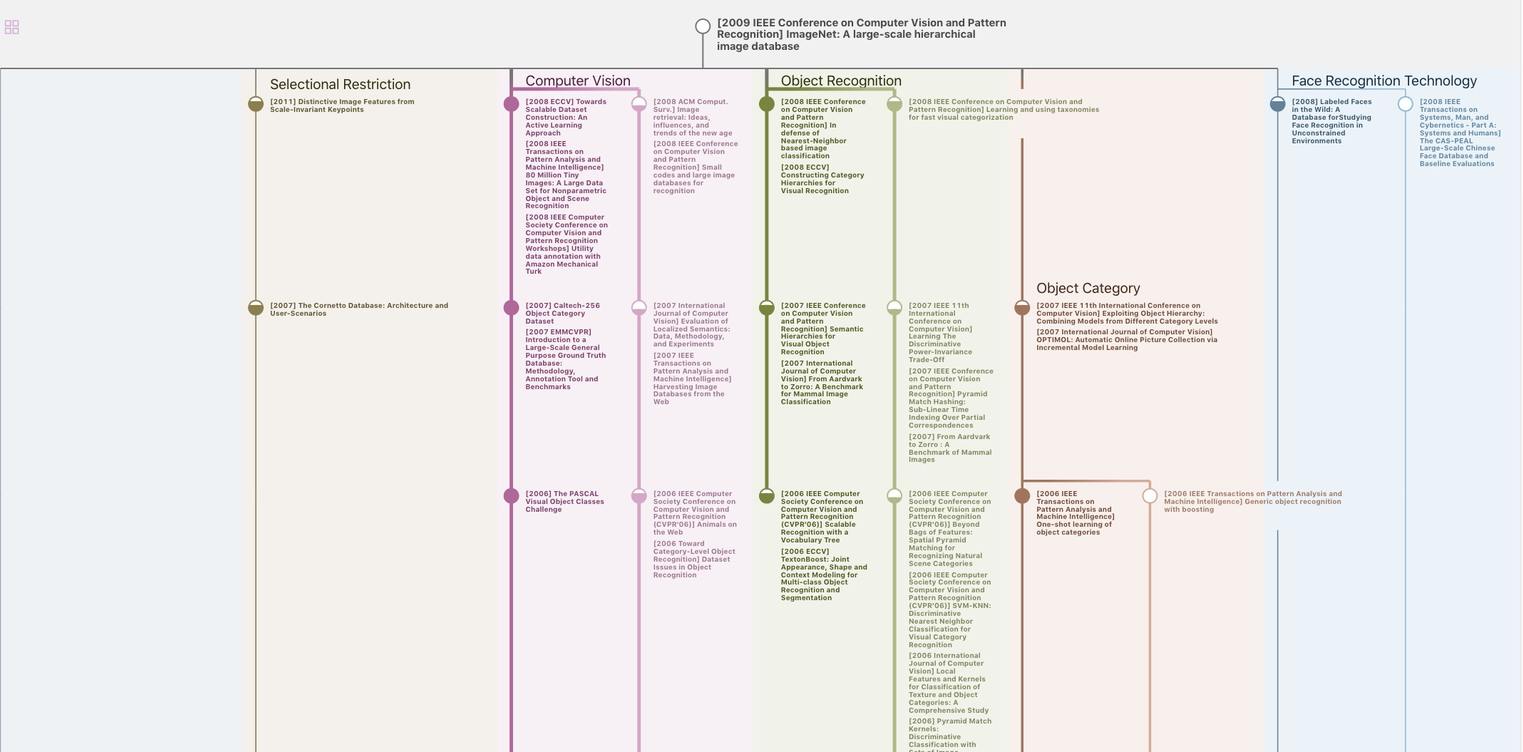
生成溯源树,研究论文发展脉络
Chat Paper
正在生成论文摘要