A Bayesian Uncertainty Quantification Approach for Agent-Based Modeling of Networked Anagram Games
2022 Winter Simulation Conference (WSC)(2022)
摘要
In group anagram games, players cooperate to form words by sharing letters that they are initially given. The aim is to form as many words as possible as a group, within five minutes. Players take several different actions: requesting letters from their neighbors, replying to letter requests, and forming words. Agent-based models (ABMs) for the game compute likelihoods of each player's next action, which contain uncertainty, as they are estimated from experimental data. We adopt a Bayesian approach as a natural means of quantifying uncertainty, to enhance the ABM for the group anagram game. Specifically, a Bayesian nonparametric clustering method is used to group player behaviors into different clusters without pre-specifying the number of clusters. Bayesian multi-nominal regression is adopted to model the transition probabilities among different actions of the players in the ABM. We describe the methodology and the benefits of it, and perform agent-based simulations of the game.
更多查看译文
关键词
bayesian uncertainty quantification approach,uncertainty quantification,games,agent-based
AI 理解论文
溯源树
样例
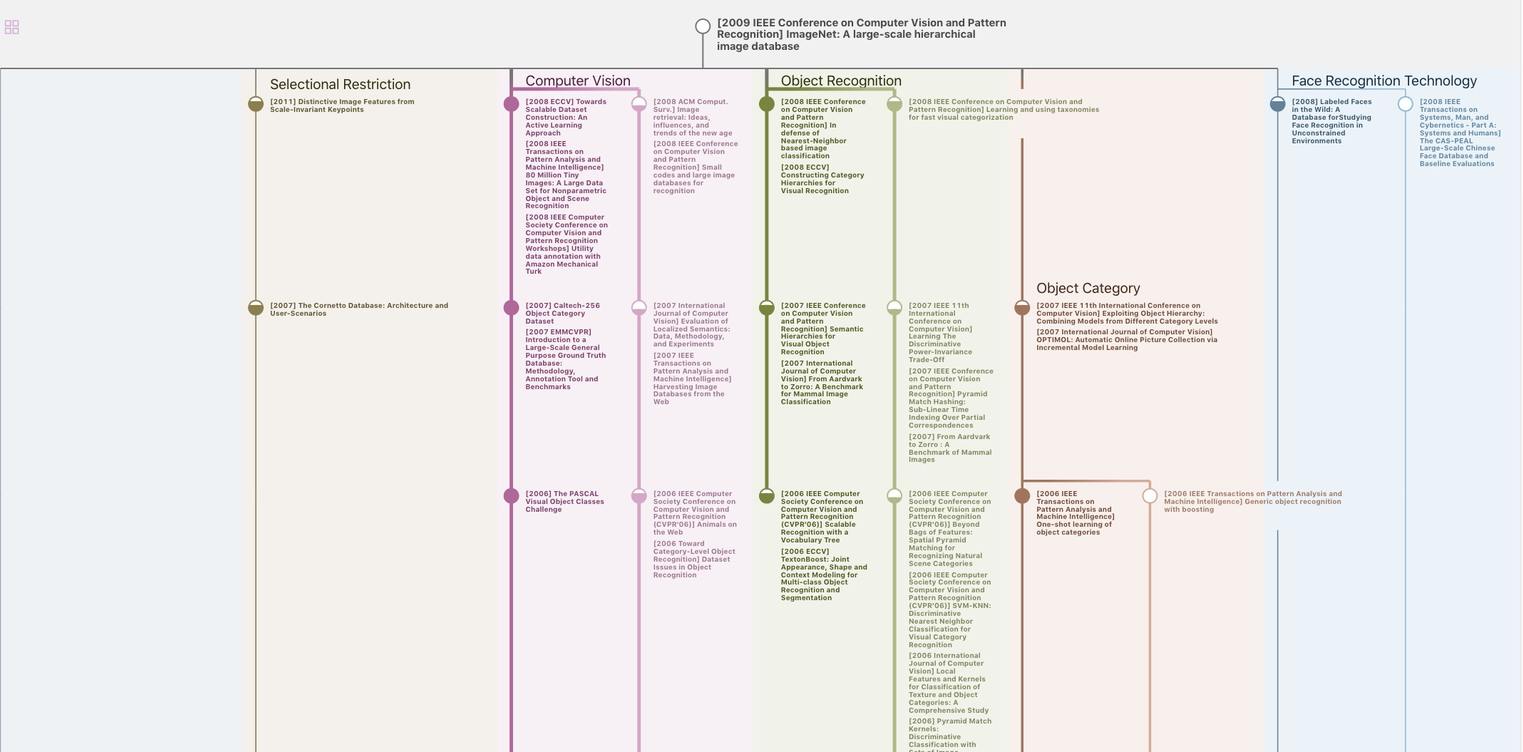
生成溯源树,研究论文发展脉络
Chat Paper
正在生成论文摘要