COMA: efficient structure-constrained molecular generation using contractive and margin losses
J. Cheminformatics(2023)
摘要
Background Structure-constrained molecular generation is a promising approach to drug discovery. The goal of structure-constrained molecular generation is to produce a novel molecule that is similar to a given source molecule (e.g. hit molecules) but has enhanced chemical properties (for lead optimization). Many structure-constrained molecular generation models with superior performance in improving chemical properties have been proposed; however, they still have difficulty producing many novel molecules that satisfy both the high structural similarities to each source molecule and improved molecular properties. Methods We propose a structure-constrained molecular generation model that utilizes contractive and margin loss terms to simultaneously achieve property improvement and high structural similarity. The proposed model has two training phases; a generator first learns molecular representation vectors using metric learning with contractive and margin losses and then explores optimized molecular structure for target property improvement via reinforcement learning. Results We demonstrate the superiority of our proposed method by comparing it with various state-of-the-art baselines and through ablation studies. Furthermore, we demonstrate the use of our method in drug discovery using an example of sorafenib-like molecular generation in patients with drug resistance.
更多查看译文
关键词
Contrastive learning,Deep generative model,Drug design,Goal-directed molecular generation,Metric learning,Molecular optimization,Reinforcement learning,Structure-constrained molecular generation
AI 理解论文
溯源树
样例
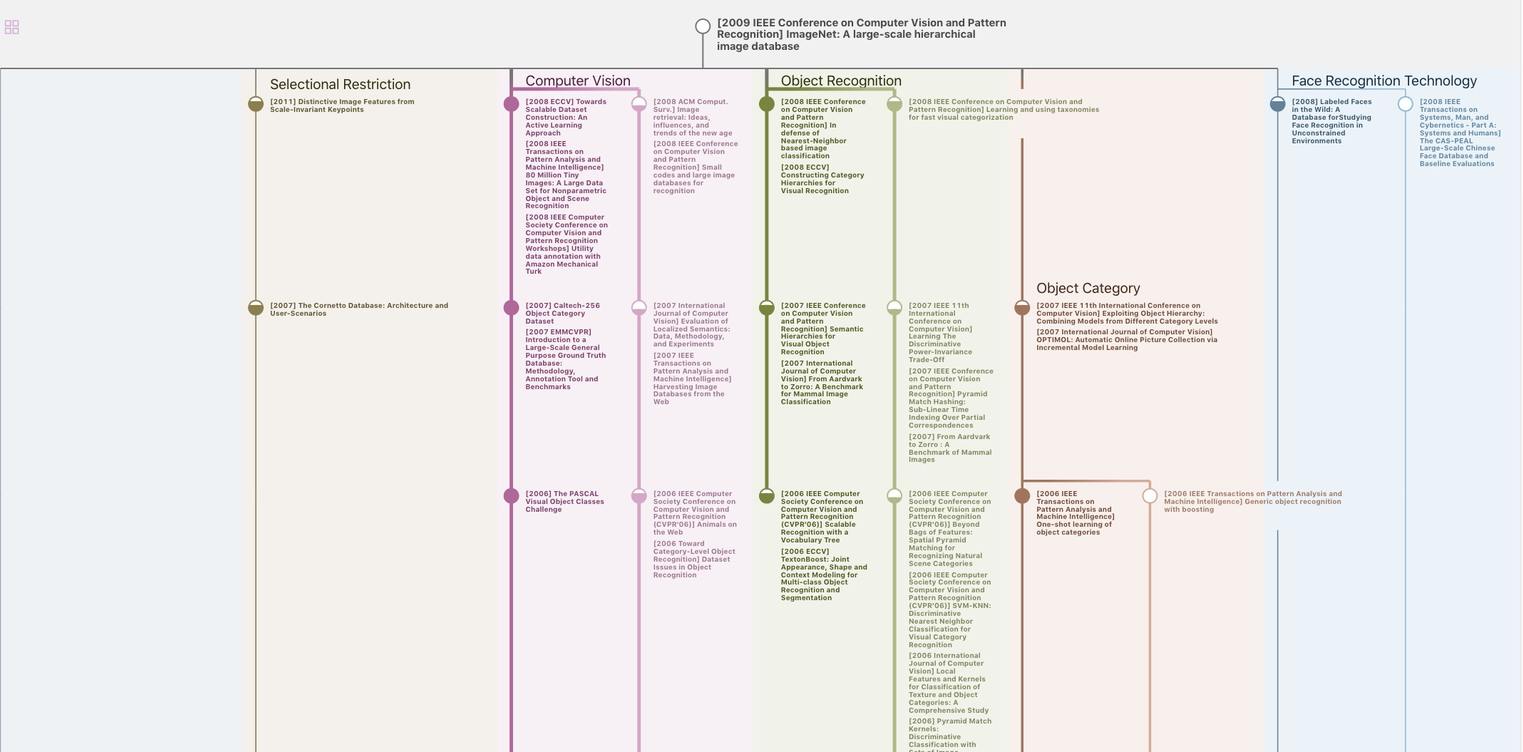
生成溯源树,研究论文发展脉络
Chat Paper
正在生成论文摘要