Multi-Fidelity Data Aggregation for Information Fusion in Simulation and Experiment
AIAA SCITECH 2023 Forum(2023)
摘要
Great potentials of multi-fidelity (MF) modeling exist in many engineering fields. An MF framework is proposed based on neural networks (NN). The methodology is named Multi-fidelity Data Aggregation Using Convolutional Neural Networks (MDACNN). MDACNN is conducted to integrate low-fidelity (LF) and high-fidelity (HF) data. It is designed to fully utilize the LF data to learn the relationship across fidelities by correlating each HF data with all available LF data. A simulation and an experiment engineering application are presented with different realizations of multi-fidelity. The first problem concerns the finite element simulation process of DTs. Coarse and fine mesh are applied with different accuracies and computational costs. The second problem investigates the internal defects of additively manufactured products. Low/high resolution and full/partial field scanning data are utilized to build DTs with different fidelities. The HF DT contains more details with more data acquiring time, while the LF DT is the opposite. Results show that with MDACNN, predictions can be made with high accuracy and low computational cost compared to HF models.
更多查看译文
关键词
information fusion,aggregation,data,multi-fidelity
AI 理解论文
溯源树
样例
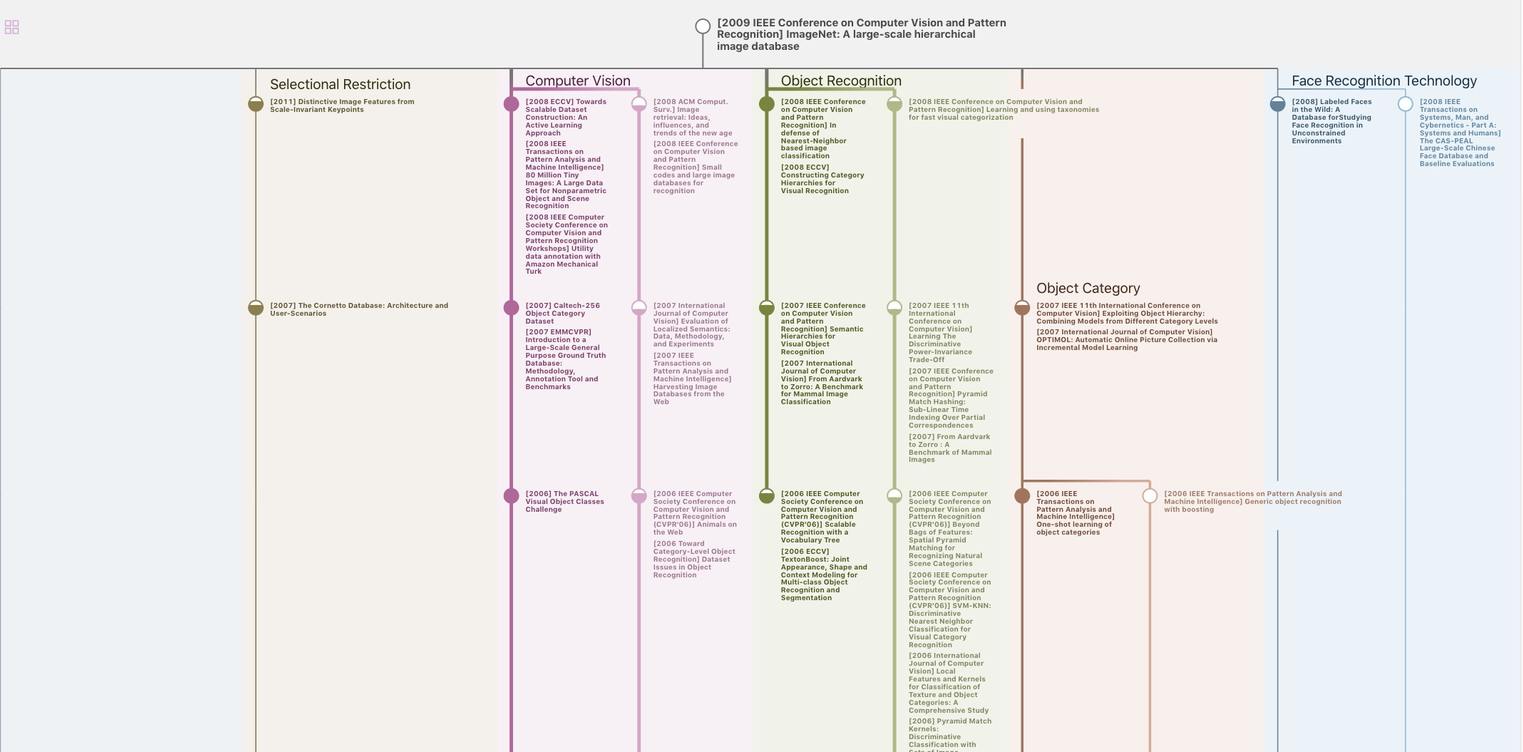
生成溯源树,研究论文发展脉络
Chat Paper
正在生成论文摘要