Sub-grid Scale Modeling of Meso-scale Hurricane Boundary Layer Flows using Machine Learning
AIAA SCITECH 2023 Forum(2023)
摘要
Modeling turbulent flows in natural hazard like hurricanes at the meso-scale is still challenging. Large-eddy simulations (LES) can be used to solve meso-scale atmospheric flows, either in an implicit approach or augmented with an explicit sub-grid scale (SGS) model. Most SGS models assume that the turbulent kinetic energy is solely transported from large scales to smaller ones. Therefore, the SGS model is purely dissipative. However, it has been realized that quantifying and modeling the opposite energy transport from small scales to larger ones (i.e., energy backscatter) in conditions like hurricane modelling is important to incorporate correct dynamics into the complex system. In the meso-scale regime there is very limited work on characterizing effects of inter-scale energy transfer. Data-driven machine/deep learning methodology is examined in this work as possible alternatives to traditional SGS modeling approaches. Basic feed-forward neural network is used to model the meso-scale energy transfer, including both forward energy cascade and energy backscatter, using filtered input data from fine-scale LES simulation of a hurricane-like vortex.
更多查看译文
关键词
boundary layer,modeling,sub-grid,meso-scale
AI 理解论文
溯源树
样例
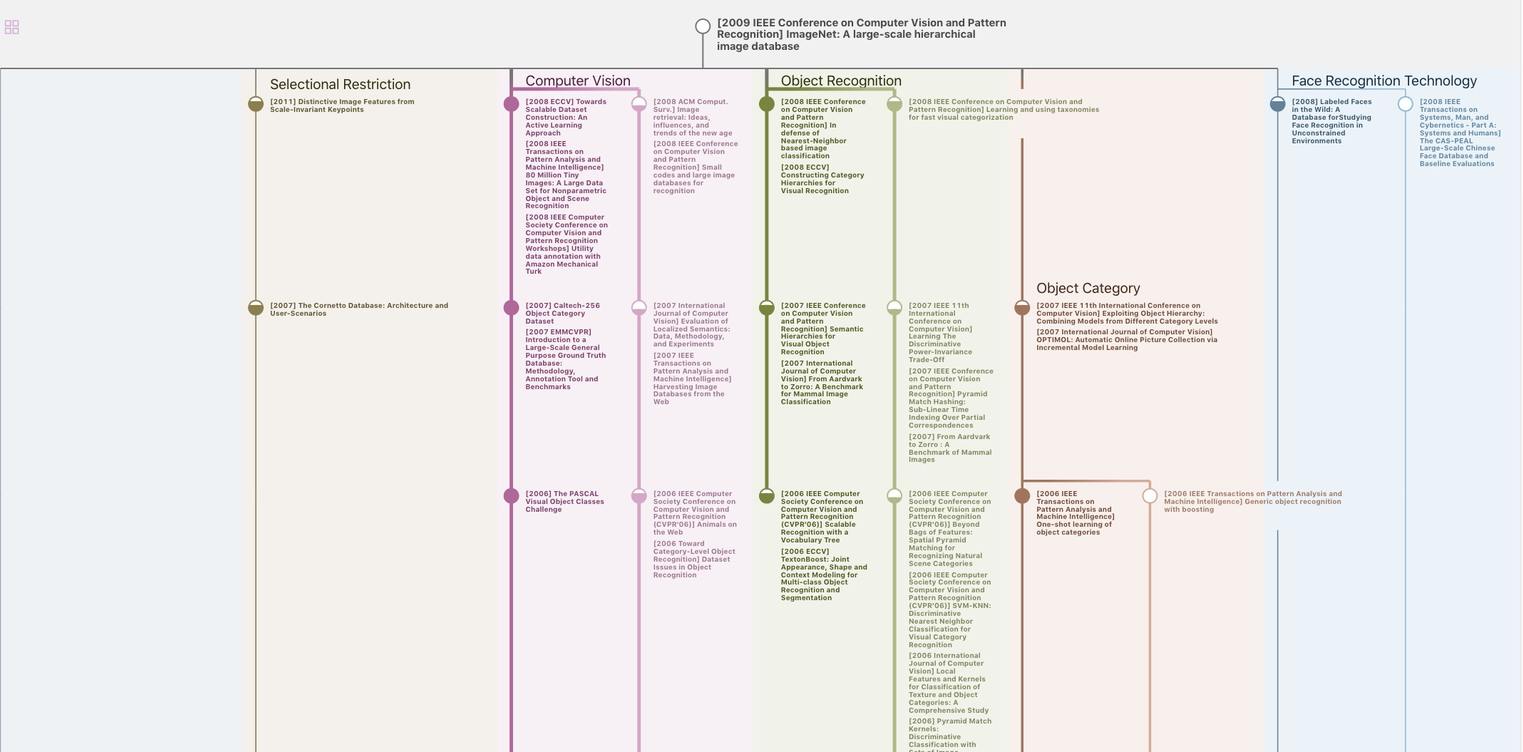
生成溯源树,研究论文发展脉络
Chat Paper
正在生成论文摘要