On Uncertainty Quantification in Materials Modeling and Discovery: Applications of GE's BHM and IDACE
AIAA SCITECH 2023 Forum(2023)
摘要
The coupling of artificial intelligence and materials characterizations has been a center piece of almost all materials discovery efforts since 1990. Furthermore, with the constant development in probabilistic machine learning tools (i.e. machine learning models that can efficiently propagate uncertainty from inputs to outputs), the synergistic interplay between materials expert knowledge and machine learning model accuracy has been a major driver for state-of-the-art materials research. However, there still exist major challenges facing the machine learning community today when it comes to materials discovery. Be it properly representing uncertainties in the data, or leveraging materials expert knowledge in the learning phase, or actively learning from the usually-sparse, and in many cases non-comprehensive, data sets available to the machine learning expert. These challenges need to be overcome to accelerate research endeavors in the domain of material discovery. To this end, General Electric Research (GER) has been developing and applying highly robust, industry-grade tools that can do tasks like uncertainty quantification (UQ), probabilistic calibration, meta-modelling, active learning, and many more. Application and demonstration of two such tools namely Bayesian Hybrid Modeling (GEBHM) and Intelligent Design and Analysis of Computer Experiments (IDACE) are presented in this paper in the context of materials modeling and discovery.
更多查看译文
关键词
uncertainty quantification,materials modeling
AI 理解论文
溯源树
样例
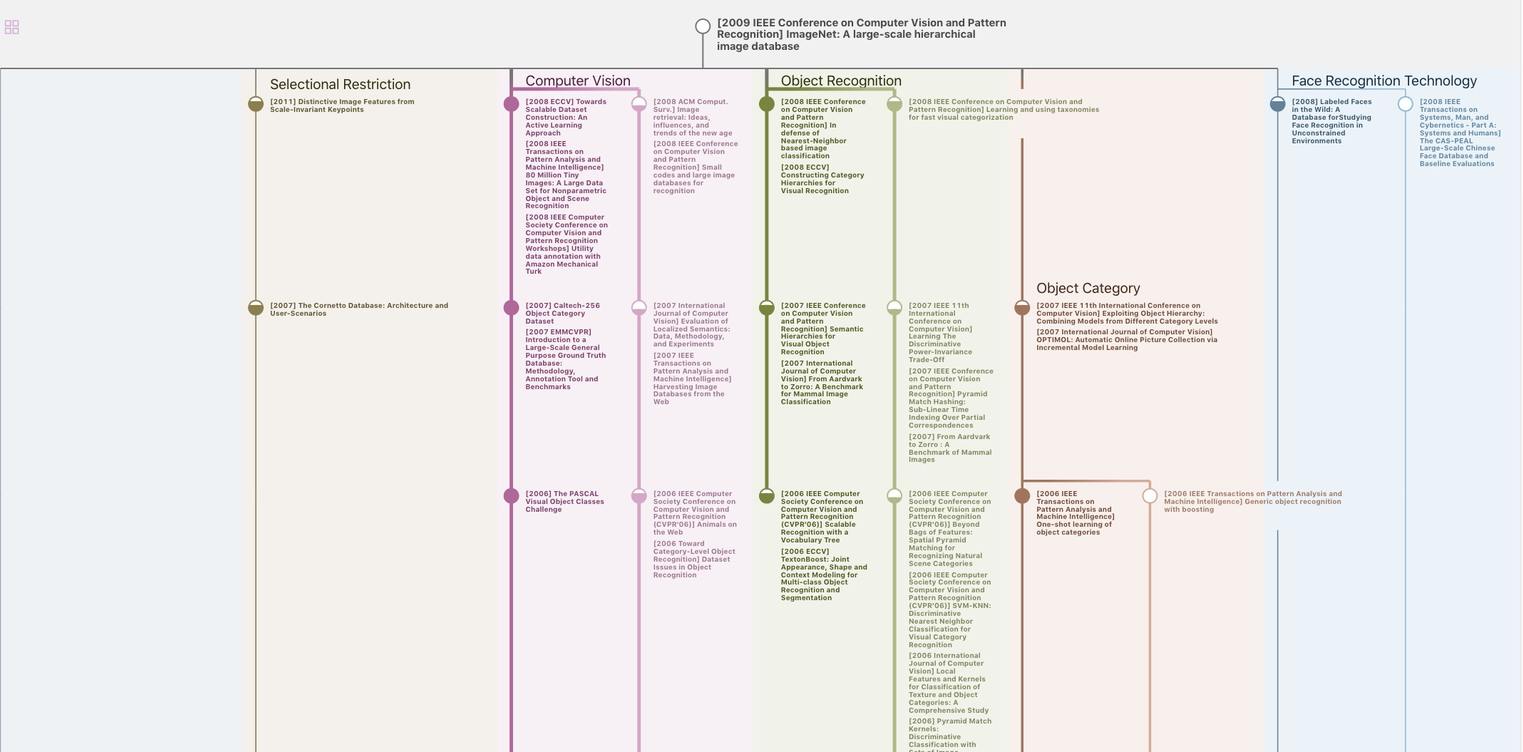
生成溯源树,研究论文发展脉络
Chat Paper
正在生成论文摘要