Turbulence modeling for compressible flows using discrepancy tensor-basis neural networks and extrapolation detection
AIAA SCITECH 2023 Forum(2023)
摘要
The Reynolds-averaged Navier–Stokes (RANS) equations remain a workhorse technology for simulating compressible fluid flows of practical interest. Due to model-form errors, however, RANS models can yield erroneous predictions that preclude their use on mission-critical problems. This work presents a data-driven turbulence modeling strategy aimed at improving RANS models for compressible fluid flows. The strategy outlined has three core aspects: (1) prediction for the discrepancy in the Reynolds stress tensor and turbulent heat flux via machine learning (ML), (2) estimating uncertainties in ML model outputs via out-of-distribution detection, and (3) multi-step training strategies to improve feature-response consistency. Results are presented across a range of cases publicly available on NASA’s turbulence modeling resource involving wall-bounded flows, jet flows, and hypersonic boundary layer flows with cold walls. We find that one ML turbulence model is able to provide consistent improvements for numerous quantities-of-interest across all cases.
更多查看译文
关键词
compressible flows,turbulence,extrapolation detection,neural networks,tensor-basis
AI 理解论文
溯源树
样例
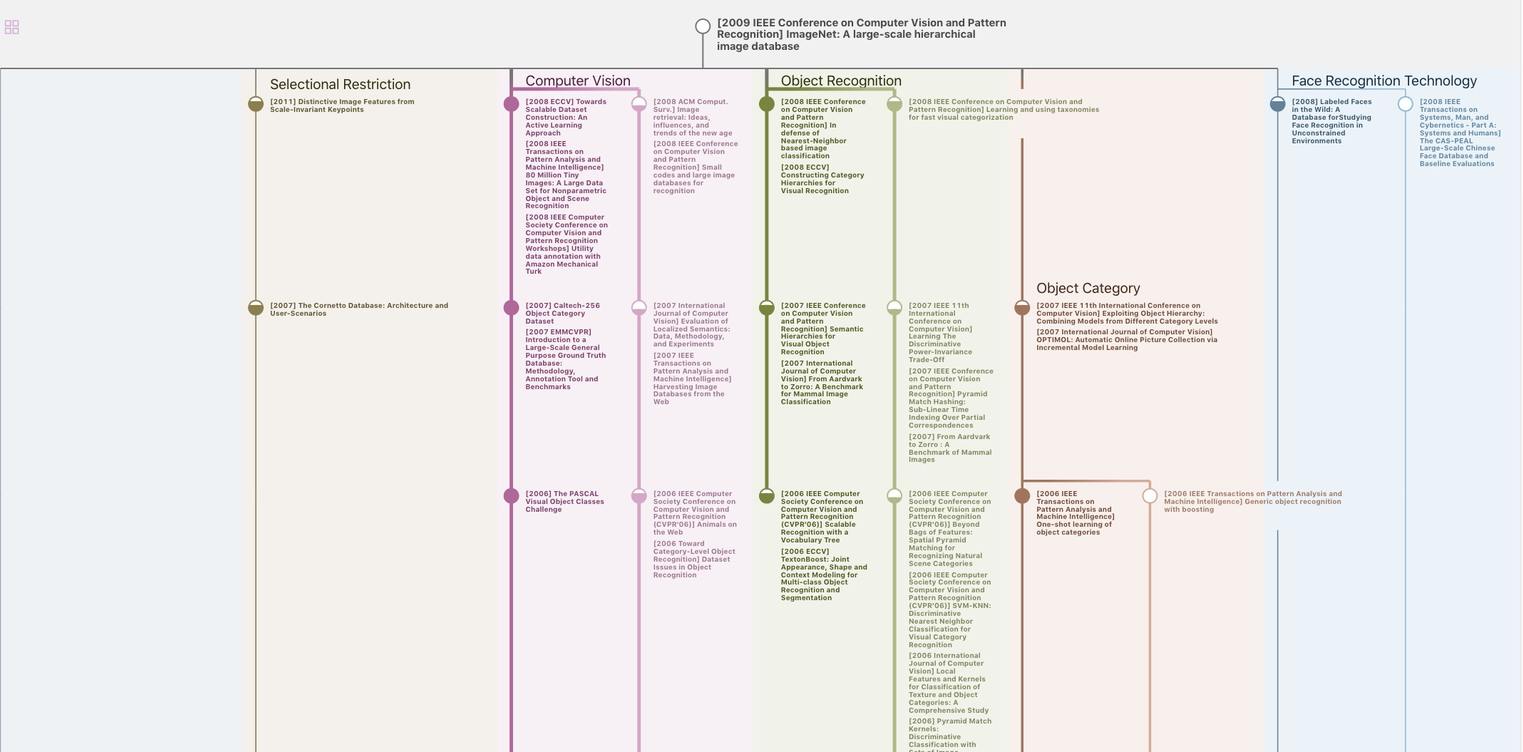
生成溯源树,研究论文发展脉络
Chat Paper
正在生成论文摘要