Airport Runway Configuration Management with Offline Model-Free Reinforcement Learning
AIAA SCITECH 2023 Forum(2023)
摘要
Runway configuration management (RCM) deals with the optimal selection of runways to operate on (for arrivals and departures) based on traffic, surface wind speed, wind direction and other environmental variables. RCM is one of the most challenging tasks in air traffic management, as it relies on operational and environmental variables (e.g., weather forecast) that are highly uncertain and complex to model. In this paper, an innovative and automated approach is deployed using offline model-free reinforcement learning to provide decision-support for RCM. The proposed technology processes historical data about variables of interest, decisions made regarding RCM, and their subsequent outcome, to identify a policy that would encourage good decisions and avoid the poor ones. The policy search is guided by an appropriately chosen weighted utility function (e.g., based on minimizing delays and go-arounds). Finally, the performance of the proposed tool is validated using Charlotte Douglas International Airport as the case study, which shows that the proposed method is superior to other conventional rule-based approaches.
更多查看译文
关键词
reinforcement learning,model-free
AI 理解论文
溯源树
样例
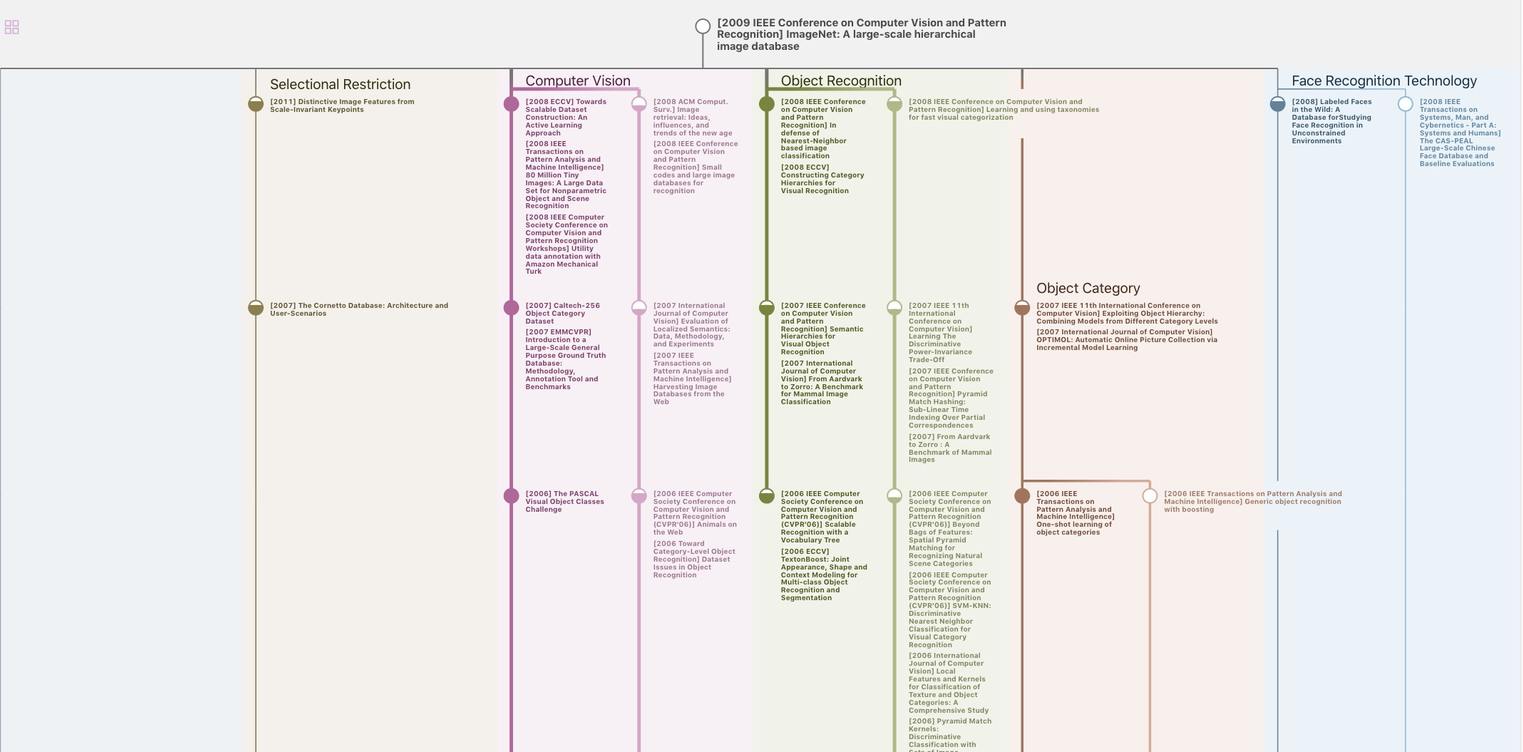
生成溯源树,研究论文发展脉络
Chat Paper
正在生成论文摘要