Adversarial Blur-Deblur Network for Robust UAV Tracking
IEEE Robotics and Automation Letters(2023)
摘要
Unmanned aerial vehicle (UAV) tracking has been widely applied in real-world applications such as surveillance and monitoring. However, the inherent high maneuverability and agility of UAV often lead to motion blur, which can impair the visual appearance of the target object and easily degrade the existing trackers. To overcome this challenge, this work proposes a tracking-oriented adversarial blur-deblur network (ABDNet), composed of a novel deblurrer to recover the visual appearance of the tracked object, and a brand-new blur generator to produce realistic blurry images for adversarial training. More specifically, the deblurrer progressively refines the features through pixel-wise, spatial-wise, and channel-wise stages to achieve excellent deblurring performance. The blur generator adaptively fuses an image sequence with a learnable kernel to create realistic blurry images. During training, ABDNet is plugged into the state-of-the-art real-time trackers and trained with blurring-deblurring loss as well as tracking loss. During inference, the blur generator is removed, while the deblurrer and the tracker can work together for UAV tracking. Extensive experiments in both public datasets and real-world testing have validated the effectiveness of ABDNet.
更多查看译文
关键词
Unmanned aerial vehicle,visual object tracking,adversarial training,realistic blur generator,robust image deblurrer
AI 理解论文
溯源树
样例
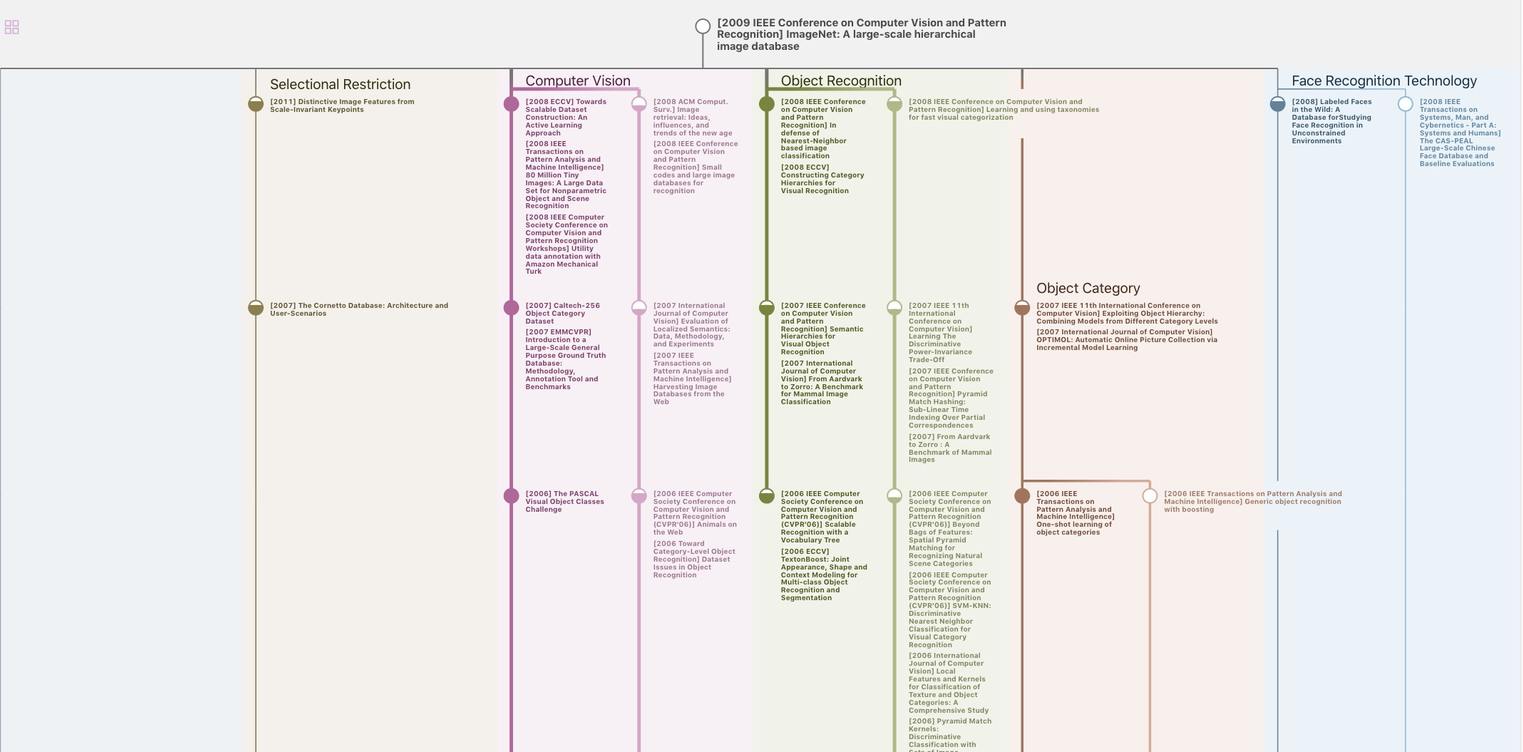
生成溯源树,研究论文发展脉络
Chat Paper
正在生成论文摘要