Vision transformer-based weakly supervised histopathological image analysis of primary brain tumors.
iScience(2023)
摘要
Diagnosis of primary brain tumors relies heavily on histopathology. Although various computational pathology methods have been developed for automated diagnosis of primary brain tumors, they usually require neuropathologists' annotation of region of interests or selection of image patches on whole-slide images (WSI). We developed an end-to-end Vision Transformer (ViT) - based deep learning architecture for brain tumor WSI analysis, yielding a highly interpretable deep-learning model, ViT-WSI. Based on the principle of weakly supervised machine learning, ViT-WSI accomplishes the task of major primary brain tumor type and subtype classification. Using a systematic gradient-based attribution analysis procedure, ViT-WSI can discover diagnostic histopathological features for primary brain tumors. Furthermore, we demonstrated that ViT-WSI has high predictive power of inferring the status of three diagnostic glioma molecular markers, mutation, mutation, and methylation, directly from H&E-stained histopathological images, with patient level AUC scores of 0.960, 0.874, and 0.845, respectively.
更多查看译文
关键词
Cancer,Machine learning,Pathology
AI 理解论文
溯源树
样例
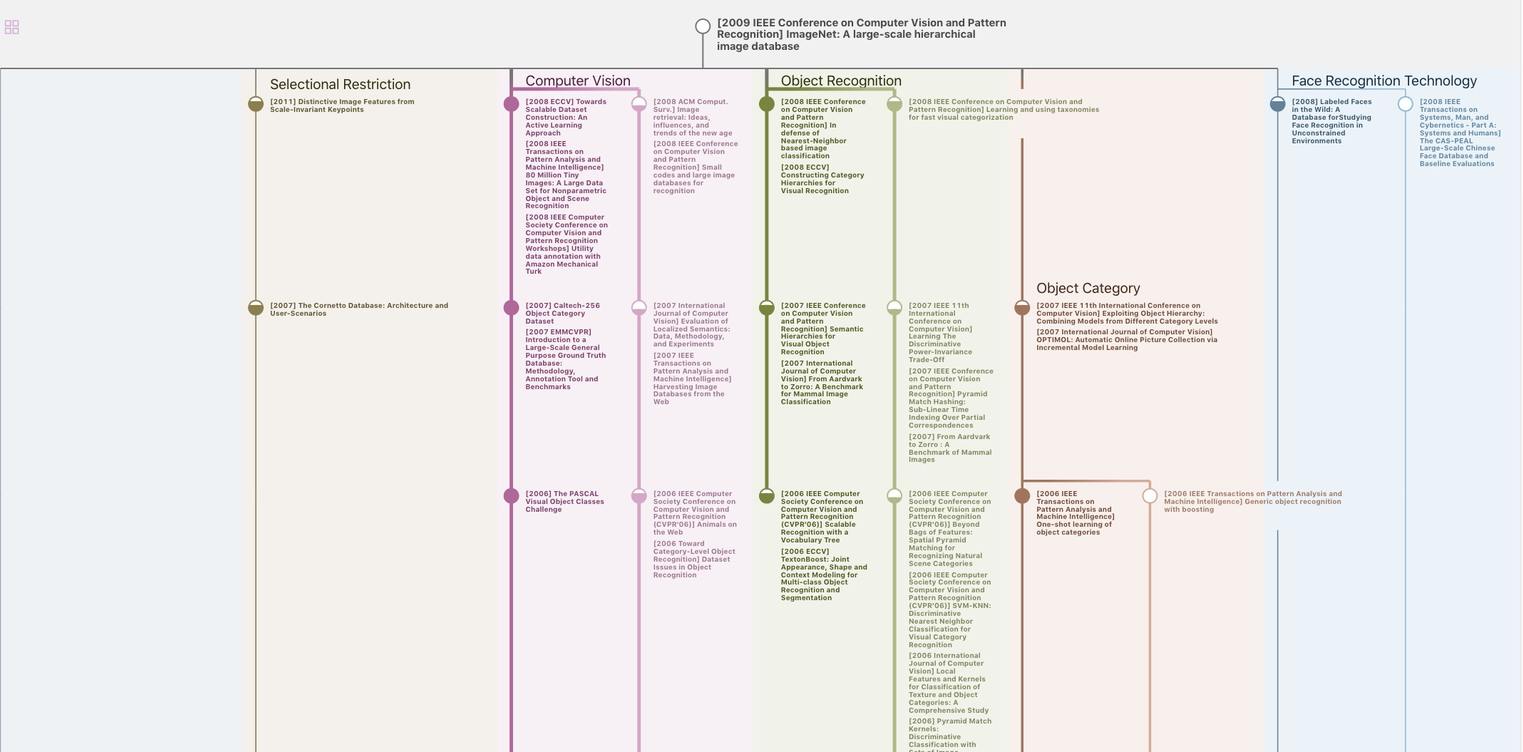
生成溯源树,研究论文发展脉络
Chat Paper
正在生成论文摘要