HCE: Improving Performance and Efficiency with Heterogeneously Compressed Neural Network Ensemble
arxiv(2023)
摘要
Ensemble learning has gain attention in resent deep learning research as a way to further boost the accuracy and generalizability of deep neural network (DNN) models. Recent ensemble training method explores different training algorithms or settings on multiple sub-models with the same model architecture, which lead to significant burden on memory and computation cost of the ensemble model. Meanwhile, the heurtsically induced diversity may not lead to significant performance gain. We propose a new prespective on exploring the intrinsic diversity within a model architecture to build efficient DNN ensemble. We make an intriguing observation that pruning and quantization, while both leading to efficient model architecture at the cost of small accuracy drop, leads to distinct behavior in the decision boundary. To this end, we propose Heterogeneously Compressed Ensemble (HCE), where we build an efficient ensemble with the pruned and quantized variants from a pretrained DNN model. An diversity-aware training objective is proposed to further boost the performance of the HCE ensemble. Experiemnt result shows that HCE achieves significant improvement in the efficiency-accuracy tradeoff comparing to both traditional DNN ensemble training methods and previous model compression methods.
更多查看译文
关键词
compressed,neural network,performance
AI 理解论文
溯源树
样例
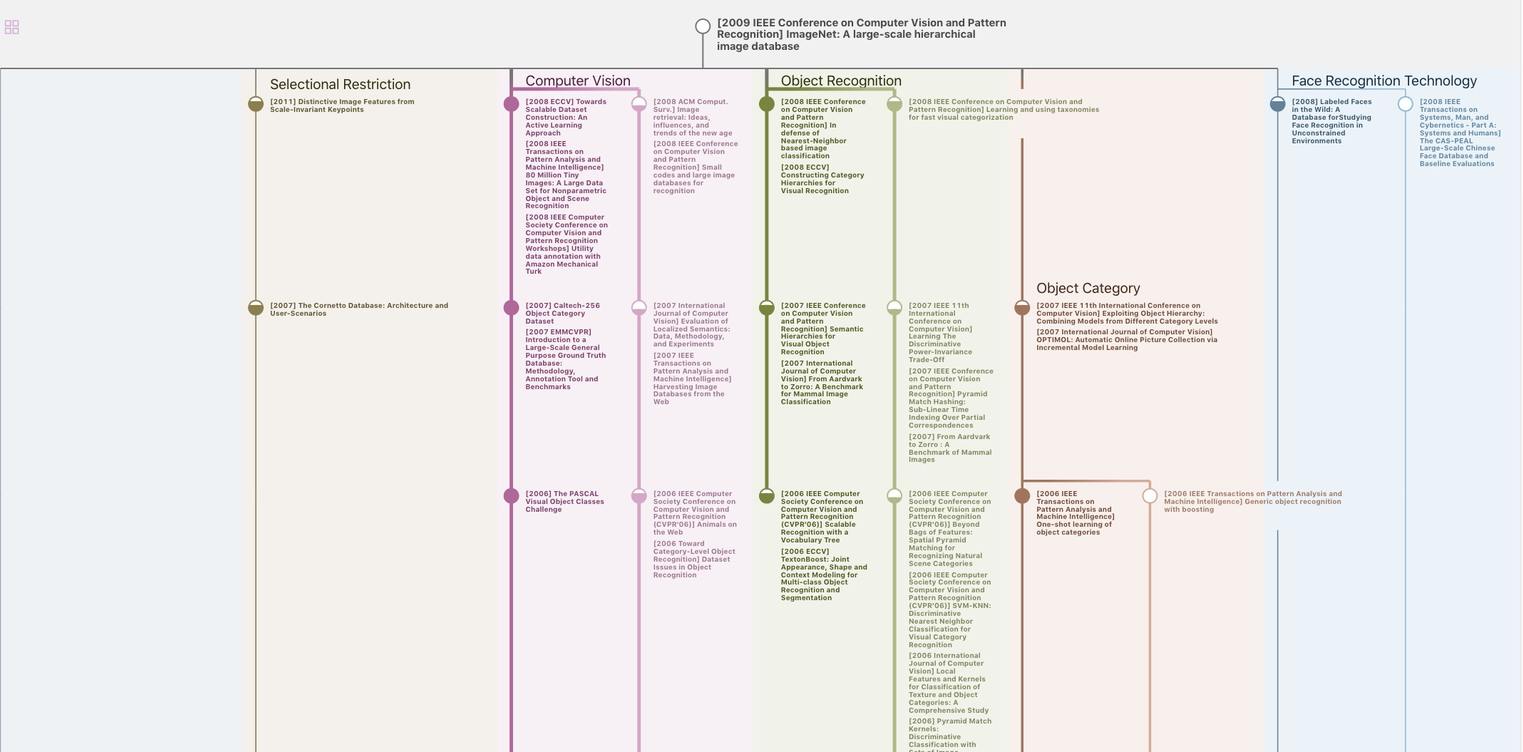
生成溯源树,研究论文发展脉络
Chat Paper
正在生成论文摘要