Antenna Array Calibration Via Gaussian Process Models
arxiv(2023)
摘要
Antenna array calibration is necessary to maintain the high fidelity of beam patterns across a wide range of advanced antenna systems and to ensure channel reciprocity in time division duplexing schemes. Despite the continuous development in this area, most existing solutions are optimised for specific radio architectures, require standardised over-the-air data transmission, or serve as extensions of conventional methods. The diversity of communication protocols and hardware creates a problematic case, since this diversity requires to design or update the calibration procedures for each new advanced antenna system. In this study, we formulate antenna calibration in an alternative way, namely as a task of functional approximation, and address it via Bayesian machine learning. Our contributions are three-fold. Firstly, we define a parameter space, based on near-field measurements, that captures the underlying hardware impairments corresponding to each radiating element, their positional offsets, as well as the mutual coupling effects between antenna elements. Secondly, Gaussian process regression is used to form models from a sparse set of the aforementioned near-field data. Once deployed, the learned non-parametric models effectively serve to continuously transform the beamforming weights of the system, resulting in corrected beam patterns. Lastly, we demonstrate the viability of the described methodology for both digital and analog beamforming antenna arrays of different scales and discuss its further extension to support real-time operation with dynamic hardware impairments.
更多查看译文
关键词
calibration
AI 理解论文
溯源树
样例
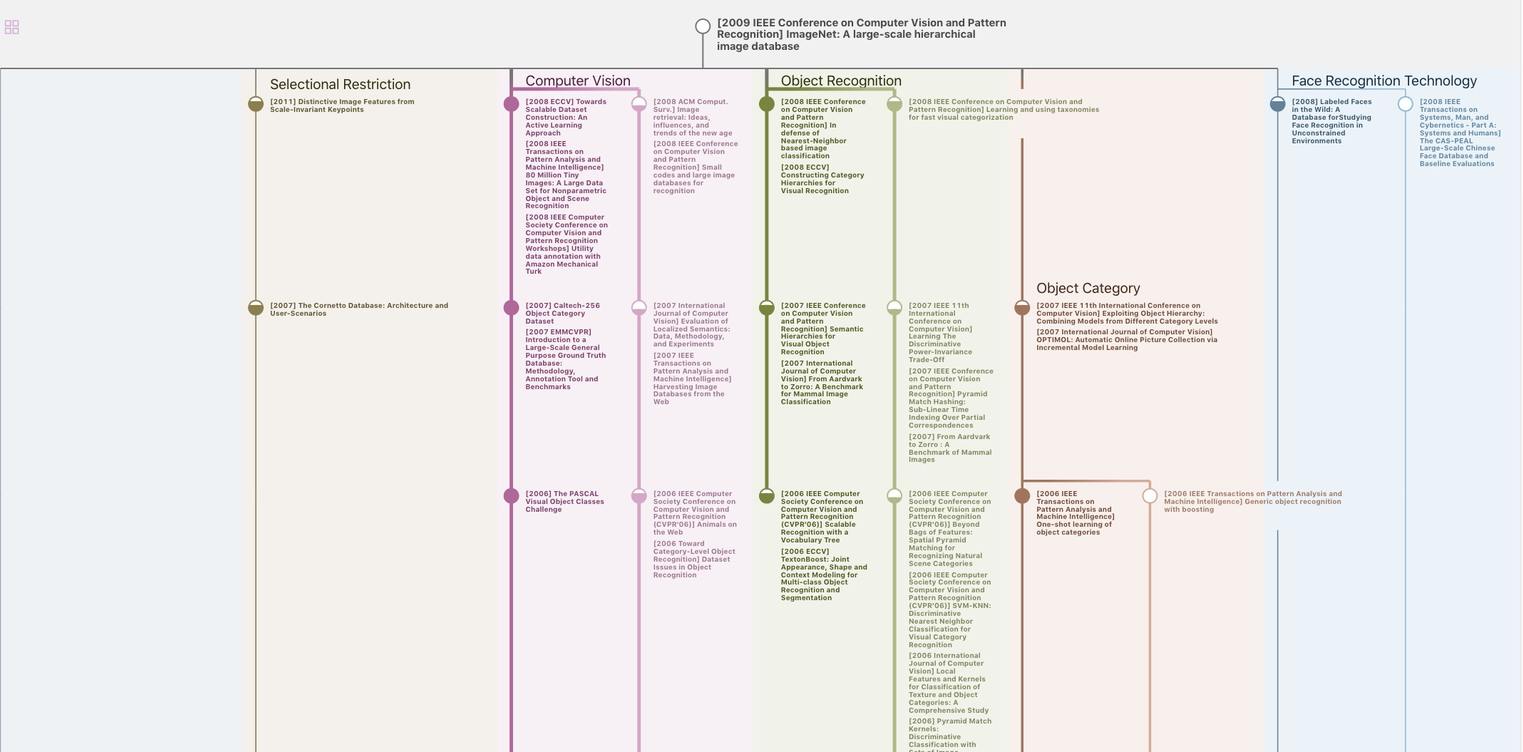
生成溯源树,研究论文发展脉络
Chat Paper
正在生成论文摘要