Bayesian and Multi-Armed Contextual Meta-Optimization for Efficient Wireless Radio Resource Management
arxiv(2023)
摘要
Optimal resource allocation in modern communication networks calls for the optimization of objective functions that are only accessible via costly separate evaluations for each candidate solution. The conventional approach carries out the optimization of resource-allocation parameters for each system configuration, characterized, e.g., by topology and traffic statistics, using global search methods such as Bayesian optimization (BO). These methods tend to require a large number of iterations, and hence a large number of key performance indicator (KPI) evaluations. In this paper, we propose the use of meta-learning to transfer knowledge from data collected from related, but distinct, configurations in order to speed up optimization on new network configurations. Specifically, we combine meta-learning with BO, as well as with multi-armed bandit (MAB) optimization, with the latter having the potential advantage of operating directly on a discrete search space. Furthermore, we introduce novel contextual meta-BO and meta-MAB algorithms, in which transfer of knowledge across configurations occurs at the level of a mapping from graph-based contextual information to resource-allocation parameters. Experiments for the problem of open loop power control (OLPC) parameter optimization for the uplink of multi-cell multi-antenna systems provide insights into the potential benefits of meta-learning and contextual optimization.
更多查看译文
关键词
Wireless resource allocation,meta-learning,open loop power control,Bayesian optimization,bandit optimization
AI 理解论文
溯源树
样例
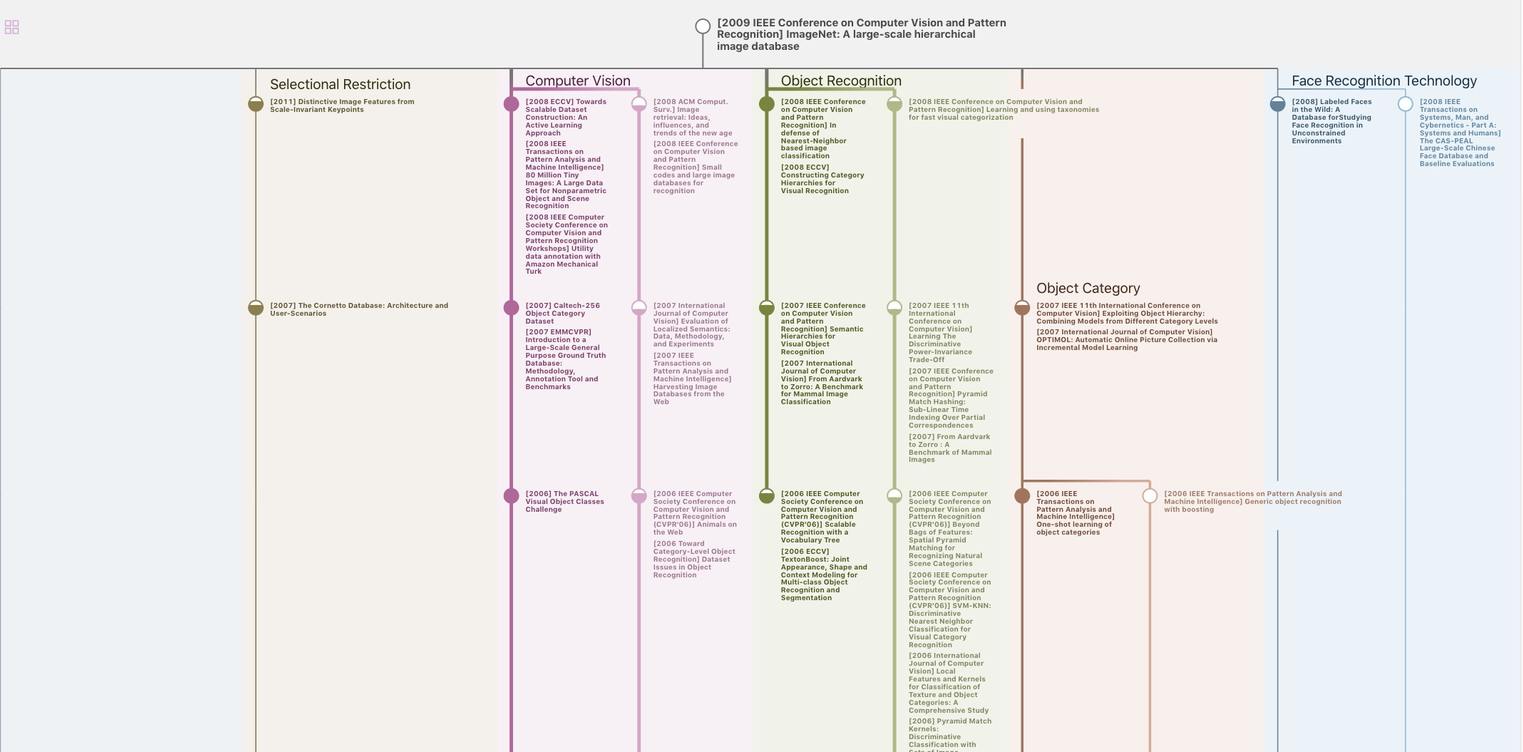
生成溯源树,研究论文发展脉络
Chat Paper
正在生成论文摘要