HiFlash: Communication-Efficient Hierarchical Federated Learning with Adaptive Staleness Control and Heterogeneity-aware Client-Edge Association
arxiv(2023)
摘要
Federated learning (FL) is a promising paradigm that enables collaboratively learning a shared model across massive clients while keeping the training data locally. However, for many existing FL systems, clients need to frequently exchange model parameters of large data size with the remote cloud server directly via wide-area networks (WAN), leading to significant communication overhead and long transmission time. To mitigate the communication bottleneck, we resort to the hierarchical federated learning paradigm of HiFL, which reaps the benefits of mobile edge computing and combines synchronous client-edge model aggregation and asynchronous edge-cloud model aggregation together to greatly reduce the traffic volumes of WAN transmissions. Specifically, we first analyze the convergence bound of HiFL theoretically and identify the key controllable factors for model performance improvement. We then advocate an enhanced design of HiFlash by innovatively integrating deep reinforcement learning based adaptive staleness control and heterogeneity-aware client-edge association strategy to boost the system efficiency and mitigate the staleness effect without compromising model accuracy. Extensive experiments corroborate the superior performance of HiFlash in model accuracy, communication reduction, and system efficiency.
更多查看译文
关键词
Client-edge association,federated learning,hierarchical mechanism,staleness control
AI 理解论文
溯源树
样例
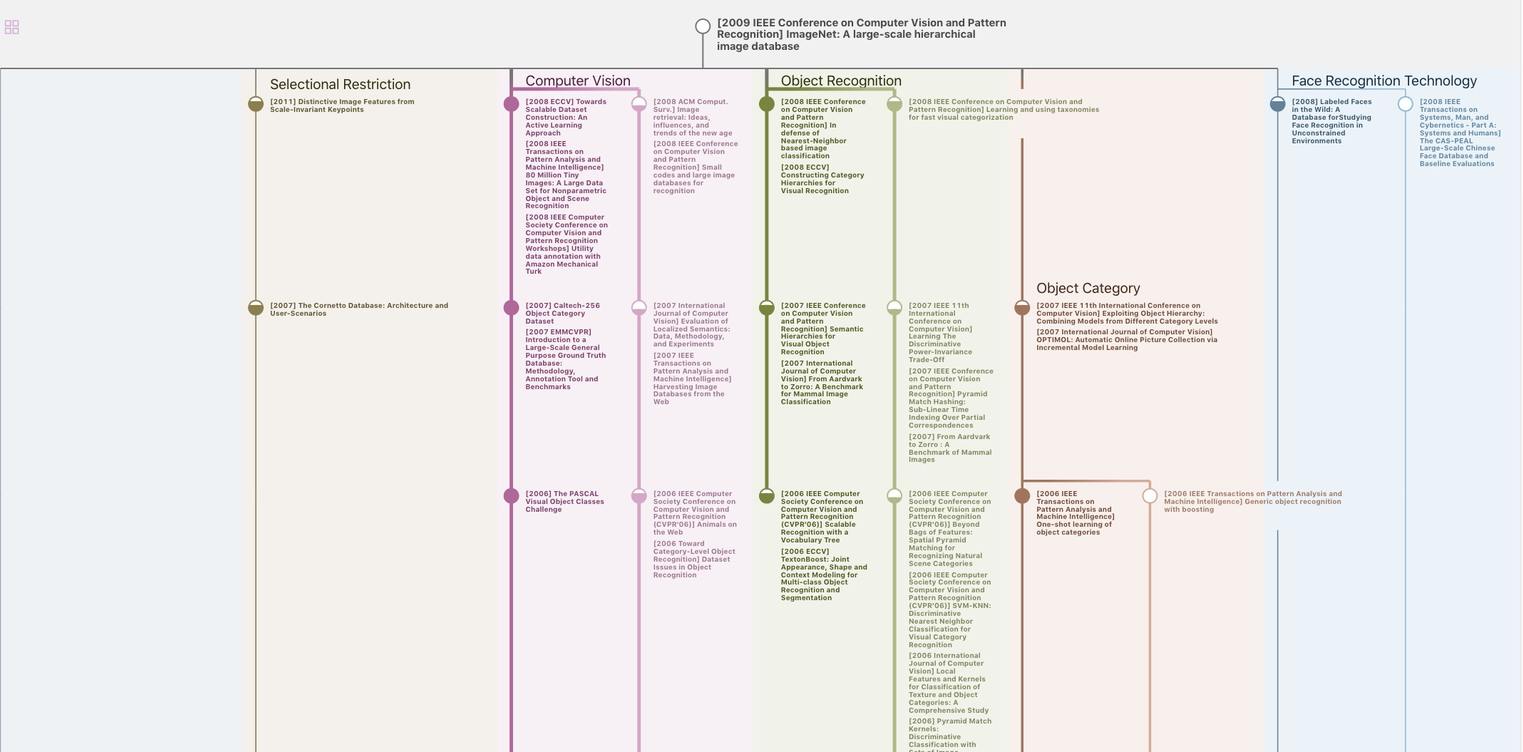
生成溯源树,研究论文发展脉络
Chat Paper
正在生成论文摘要