Action Invariant IMU-Gait for Continuous Authentication
2022 IEEE International Joint Conference on Biometrics (IJCB)(2022)
摘要
Continuous Authentication (CA) is proposed as an alter-native authentication scheme for modern personal devices. Gait is a suitable biometric for CA due to its availability and low resource requirements. However, the drastic change in the gait pattern with changes in actions (such as walking, running or climbing stairs) and changes in terrain (such as walking on a flat surface or down an incline) makes it chal-lenging to deploy in a real-world CA system. We show that standard gait features are influenced by different actions. The gait pattern of an action is also in-fluenced by the actions performed before and immediately after. Therefore, gait features are usually not robust to these action variations. We propose action invariant gait features to address this robustness issue. Our proposed method learns action invariant gait features utilizing a Siamese Net-work architecture with triplet loss and a unique triplet mining protocol. Our evaluations highlight that our action in-variant features are robust to pre and post action impacts and real world action variations. These features allows for a CA system to be enrolled using a single action (walk) and be used across multiple different actions encountered throughout the day.
更多查看译文
AI 理解论文
溯源树
样例
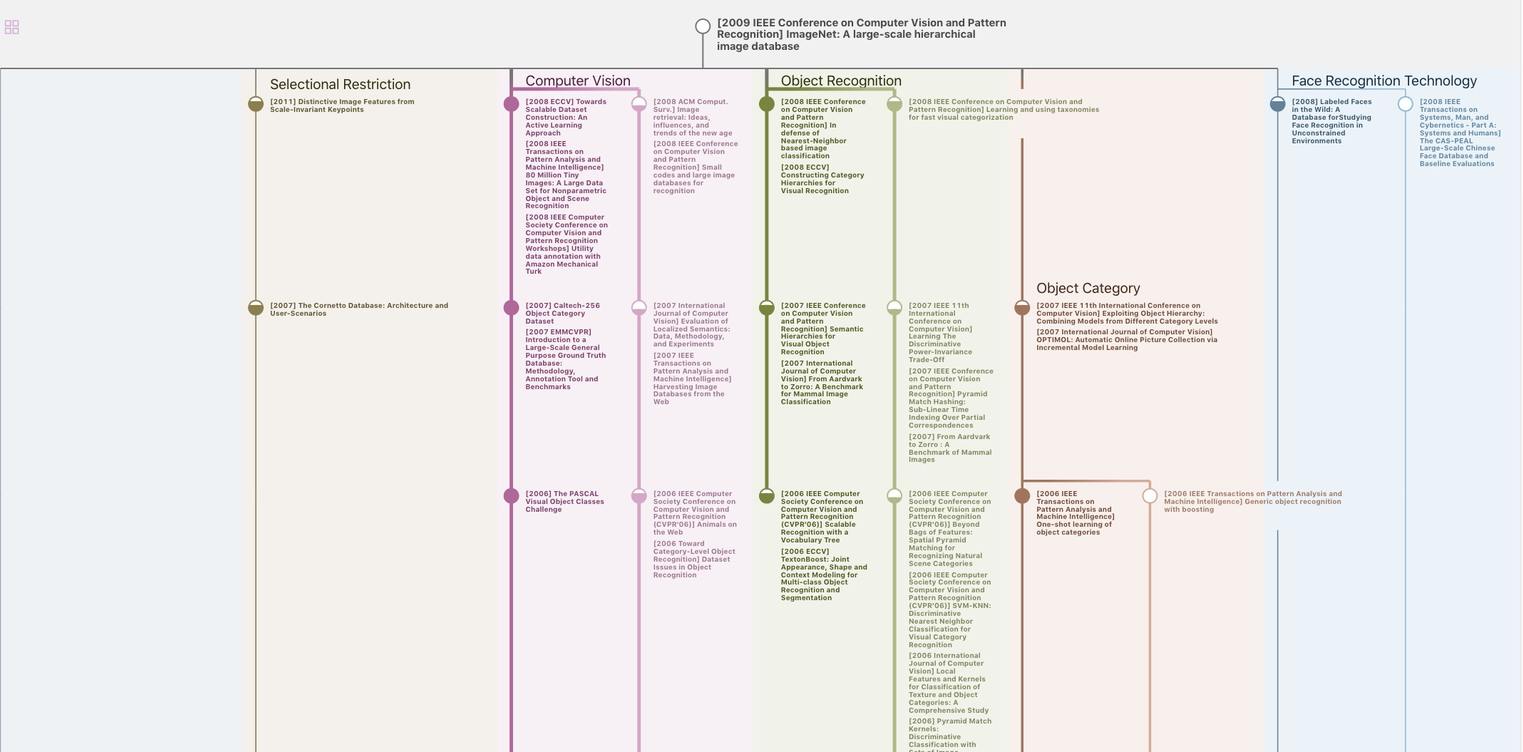
生成溯源树,研究论文发展脉络
Chat Paper
正在生成论文摘要