Multi-stage Locally and Long-range Correlated Feature Fusion for Learned In-loop Filter in VVC
2022 IEEE International Conference on Visual Communications and Image Processing (VCIP)(2022)
摘要
Versatile Video Coding (VVC)/H.266 is currently the state-of-the-art video coding standard with significant improvement in coding efficiency over its predecessor High Efficiency Video Coding (HEVC)/H.26S. Nonetheless, VVC is also block-based video coding technology where decoded pictures contain compression artifacts. In VVC, in-loop filters serve to suppress these compression artifacts. In this paper, convolution neural network (CNN) is utilized to better facilitate the suppression of compression artifacts over VVC. Nonetheless, our approach has uniqueness in obtaining better features by exploiting locally correlated spatial features in the pixel domain as well as long-range correlated spectral features in the discrete cosine transform (DCT) domain. In particular, we utilized CNN-features from DCT transformed input to extract high-frequency components and induce long-range correlation into the spatial CNN-features by employing multi-stage feature fusion. Our experimental result shows that the proposed approach achieves significant coding improvements up to 9.70% on average Bjantegaard Delta (BD)-Bitrate savings under AI configurations for luma (Y) components.
更多查看译文
关键词
Versatile Video Coding (VVC),In-Loop Filter,Convolutional Neural Network (CNN),Receptive-Field,Discrete Cosine Transform (DCT),Long Range Correlation
AI 理解论文
溯源树
样例
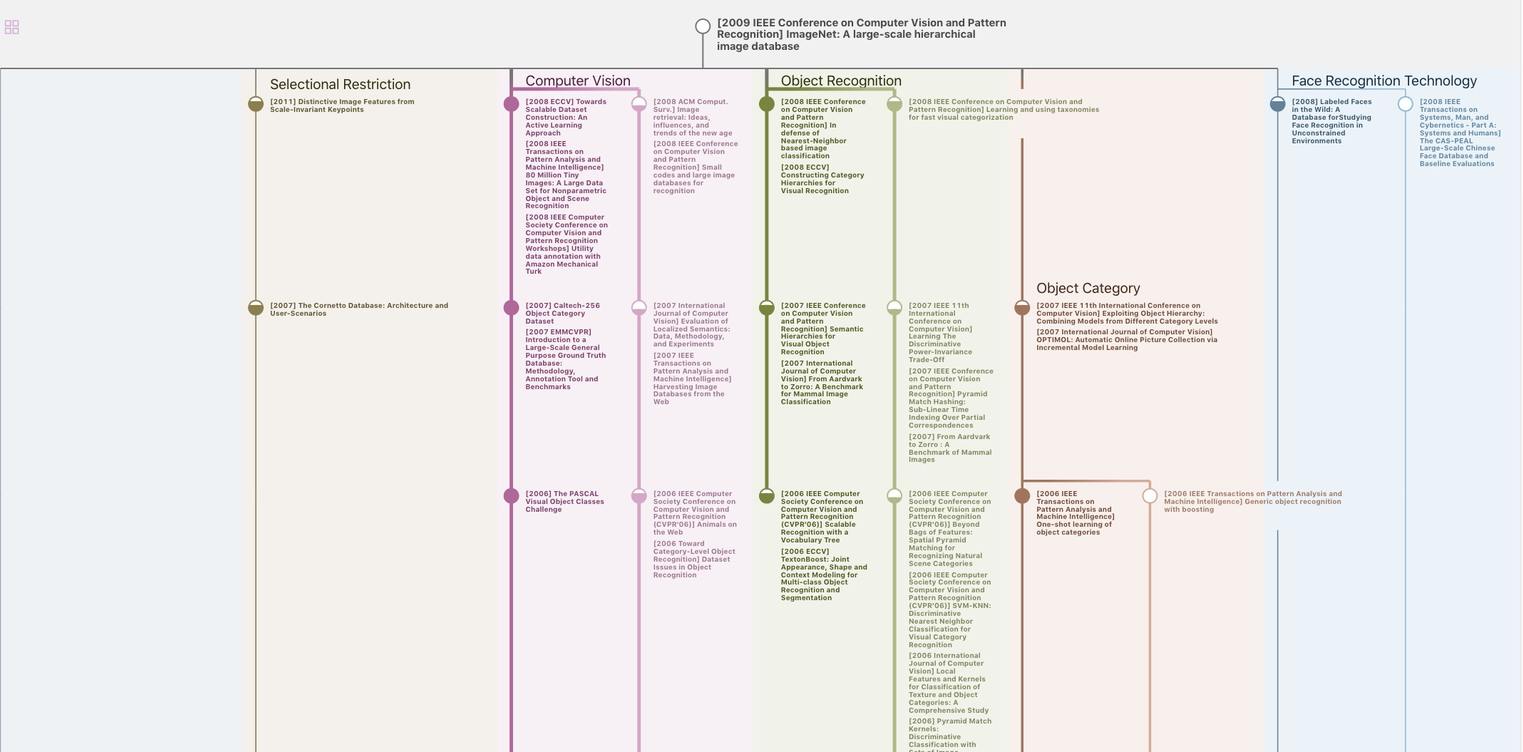
生成溯源树,研究论文发展脉络
Chat Paper
正在生成论文摘要