Uncovering specific mechanisms across cell types in dynamical models
biorxiv(2023)
摘要
Ordinary differential equations are frequently employed for mathematical modeling of biological systems. The identification of mechanisms that are specific to certain cell types is crucial for building useful models and to gain insights into the underlying biological processes. Regularization techniques have been proposed and applied to identify mechanisms specific to two cell types, e.g., healthy and cancer cells, including the LASSO (least absolute shrinkage and selection operator). However, when analyzing more than two cell types, these approaches are not consistent, and require the selection of a reference cell type, which can affect the results.
To make the regularization approach applicable to identifying cell-type specific mechanisms in any number of cell types, we propose to incorporate the clustered LASSO into the framework of ordinary differential equation modeling by penalizing the pairwise differences of the logarithmized fold-change parameters encoding a specific mechanism in different cell types. The symmetry introduced by this approach renders the results independent of the reference cell type. We discuss the necessary adaptations of state-of-the-art numerical optimization techniques and the process of model selection for this method. We assess the performance with realistic biological models and synthetic data, and demonstrate that it outperforms existing approaches. Finally, we also exemplify its application to published biological models including experimental data, and link the results to independent biological measurements.
Contact adrian.hauber{at}fdm.uni-freiburg.de
Author Summary Mathematical models enable insights into biological systems beyond what is possible in the wet lab alone. However, constructing useful models can be challenging, since they both need a certain amount of complexity to adequately describe real-world observations, and simultaneously enough simplicity to enable understanding of these observations and precise predictions. Regularization techniques were suggested to tackle this challenge, especially when building models that describe two different types of cells, such as healthy and cancer cells. Typically, both cell types have a large portion of biological mechanisms in common, and the task is to identify the relevant differences that need to be included into the model.
For more than two types of cells, the existing approaches are not readily applicable, because they require defining one of the cell types as reference, which potentially influences the results. In this work, we present a regularization method that is independent from the choice of a reference. We demonstrate its working principle and compare its performance to existing approaches. Since we implemented this method in a freely available software package, it is accessible to a broad range of researchers and will facilitate the construction of useful mathematical models for multiple types of cells.
### Competing Interest Statement
The authors have declared no competing interest.
更多查看译文
关键词
cell types,dynamical models,mechanisms
AI 理解论文
溯源树
样例
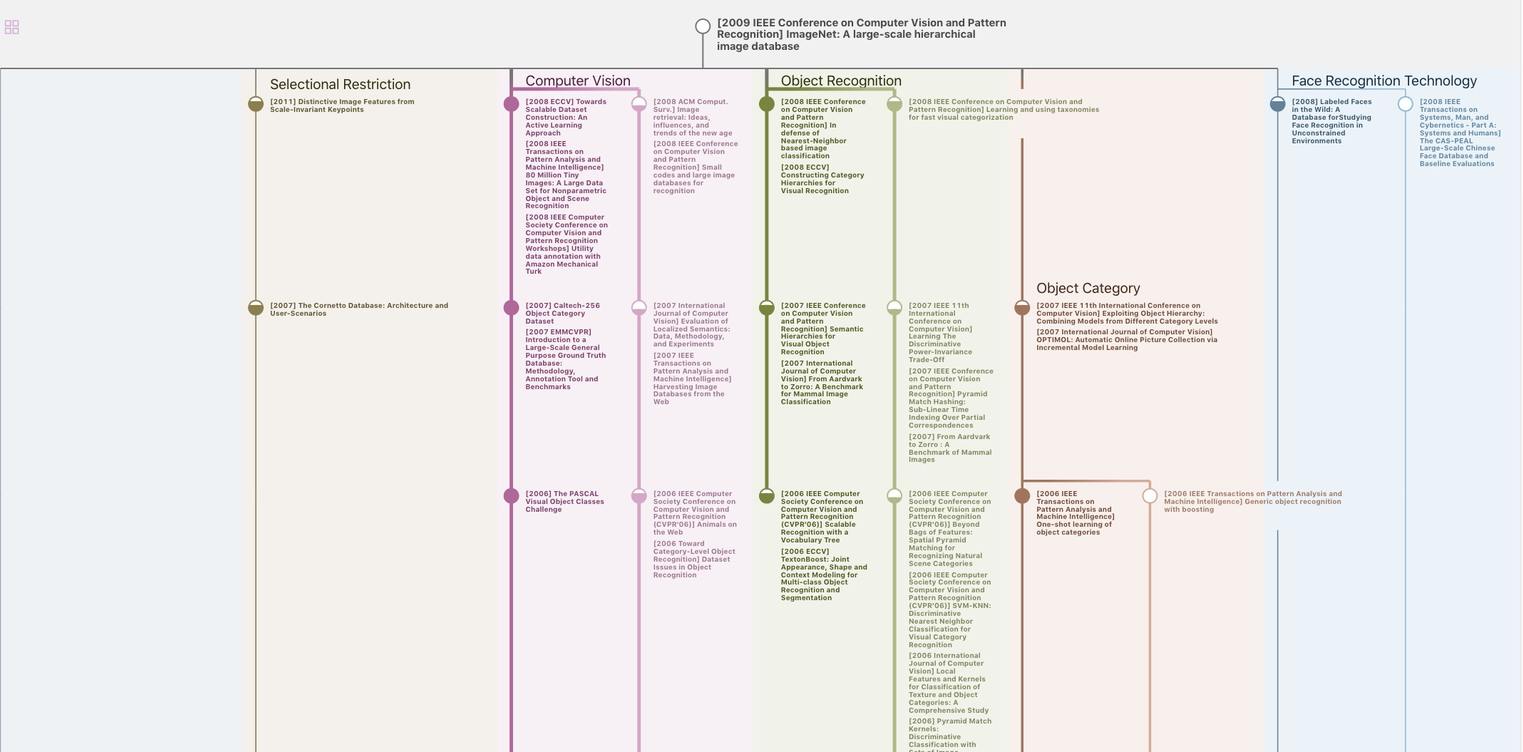
生成溯源树,研究论文发展脉络
Chat Paper
正在生成论文摘要