Compression scenarios for Federated Learning in Smart Manufacturing
Procedia Computer Science(2023)
摘要
Recent advances in Industrial Internet of Things (IIoT) and communication technologies have provided new concepts of smart manufacturing and paved the way for the new era of Industry 4.0. The huge amount of generated data from machinery, connected systems, and edge devices can be exploited to extract actionable insights that can enhance the decision-making process in smart manufacturing, targeting to improve the return of investment. Conventionally, smart decisions are obtained from centralised data using predefined machine/deep learning models. To achieve better accuracy, these models require training based on diverse, unbiased, and comprehensive datasets, which may not be feasible in distributed and remote facilities due to the high scalability of smart manufacturing and data privacy concerns. Federated learning stands as a primary solution to these issues by bringing collaborative smart manufacturing without requiring centralised data training rather than model sharing. However, these models with many parameters result in big size, which put pressure on the communication channels due to frequent transfers from server to clients and vice versa and may face communication delays and model corruption. In addition, bigger models create issues for edge devices with limited memory and processing capacity which needs consideration. Therefore, effective compression methods are mandatory to ensure Communication-Efficient Federated Learning. In this paper, first, we discuss some possible application opportunities of FL in smart manufacturing, especially for the automotive industry but that can be extended for others. Secondly, we highlight FL communication challenge in smart manufacturing for which we present the compression concept and objectives in FL. It is followed by discussing the state-of-the-art compression techniques in FL. Thirdly, a special focus is given to NNR and DeepCABAC for their demonstrated high compression gains with a wide variety of deep models. Finally, we gave some directions for compressing the FL models in the future.
更多查看译文
关键词
federated learning,compression scenarios,manufacturing
AI 理解论文
溯源树
样例
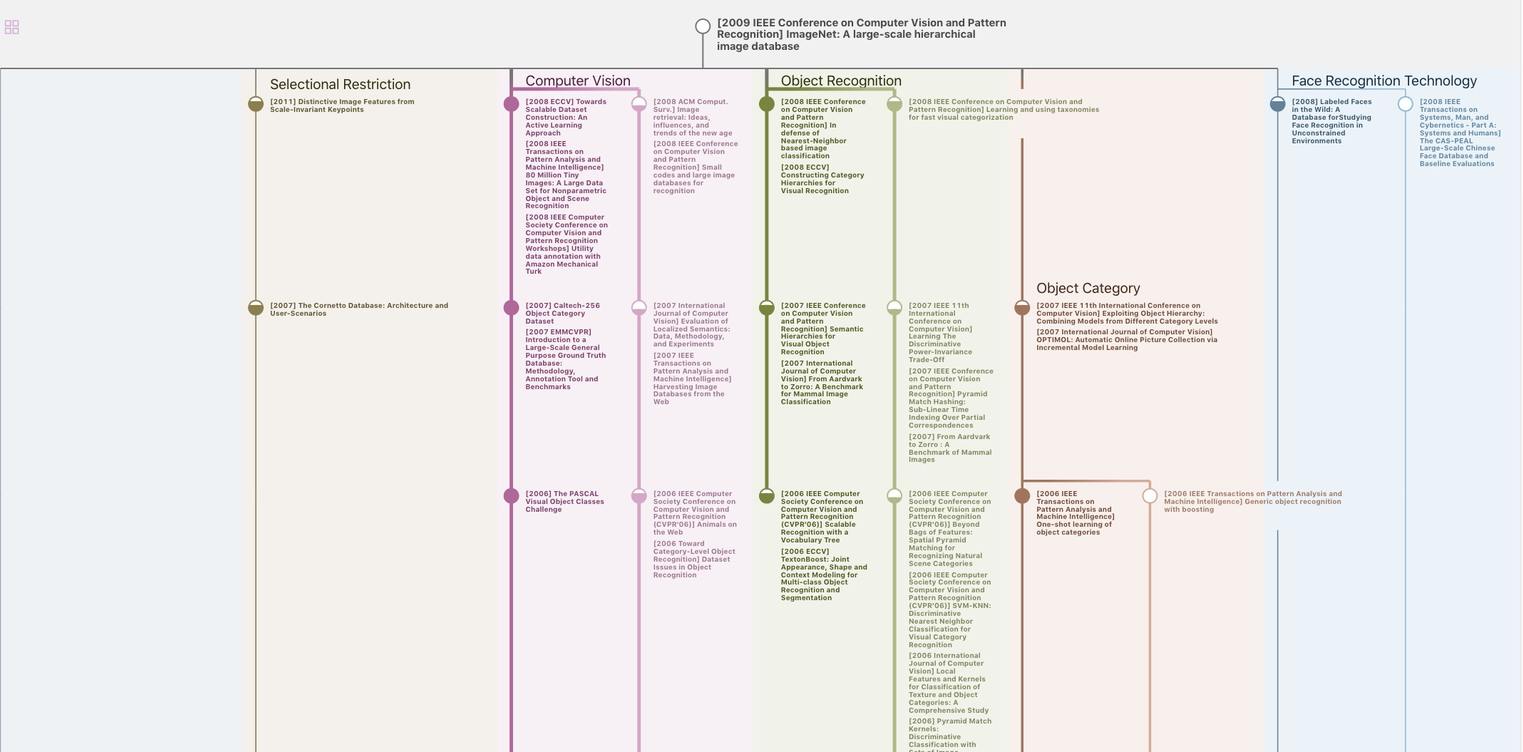
生成溯源树,研究论文发展脉络
Chat Paper
正在生成论文摘要