A meta-methodology for preserving narrow objects when using spatial contextual classifiers for remote sensing data
INTERNATIONAL JOURNAL OF REMOTE SENSING(2022)
摘要
In the field of land-cover classification with remote sensing images, methods that analyse purely the spectral information of individual pixels generally produce noisy results, due to salt-and-pepper effects. The use of methods that also incorporate spatial contextual information into classification, often defined as contextual or spectral-spatial approaches, is an effective strategy for reducing the occurrence of punctual noises and, consequently, improving accuracy. However, contextual methods still present a critical limitation: an over smoothing performance on certain classes can cause the loss of details on important spatial structures. They may overlook salient punctual and linear objects that can be efficiently classified using purely spectral information, particularly over areas of rapid class transition. This issue is commonly observed with the classification of medium spatial-resolution images that include narrow class structures, such as rivers and roads. To solve this problem, we present a strategy for contextual classification that allows adjusting a trade-off between noise smoothing and the preservation of small spatial details. The proposed strategy comprises a meta-methodology, in the sense that it does not depend on specific pixel-based and contextual classifiers. The meta-methodology for improving contextual classification methods (Meta-CTX) consists in performing a separability analysis, at the pixel level, based on the class membership estimates provided by a pixel-based classifier. The Meta-CTX analyses the distance between class membership estimates in order to identify pixels that are expected to be accurately classified using purely spectral information. The Meta-CTX preserves the per-pixel classification of these pixels. It uses spatial contextual information only to classify pixels that are more susceptible to classification errors. The experimental results indicate that the Meta-CTX can efficiently combine noise smoothing with the preservation of small spatial details in remote sensing image classification.
更多查看译文
关键词
spatial contextual classifiers,narrow objects,preserving,meta-methodology
AI 理解论文
溯源树
样例
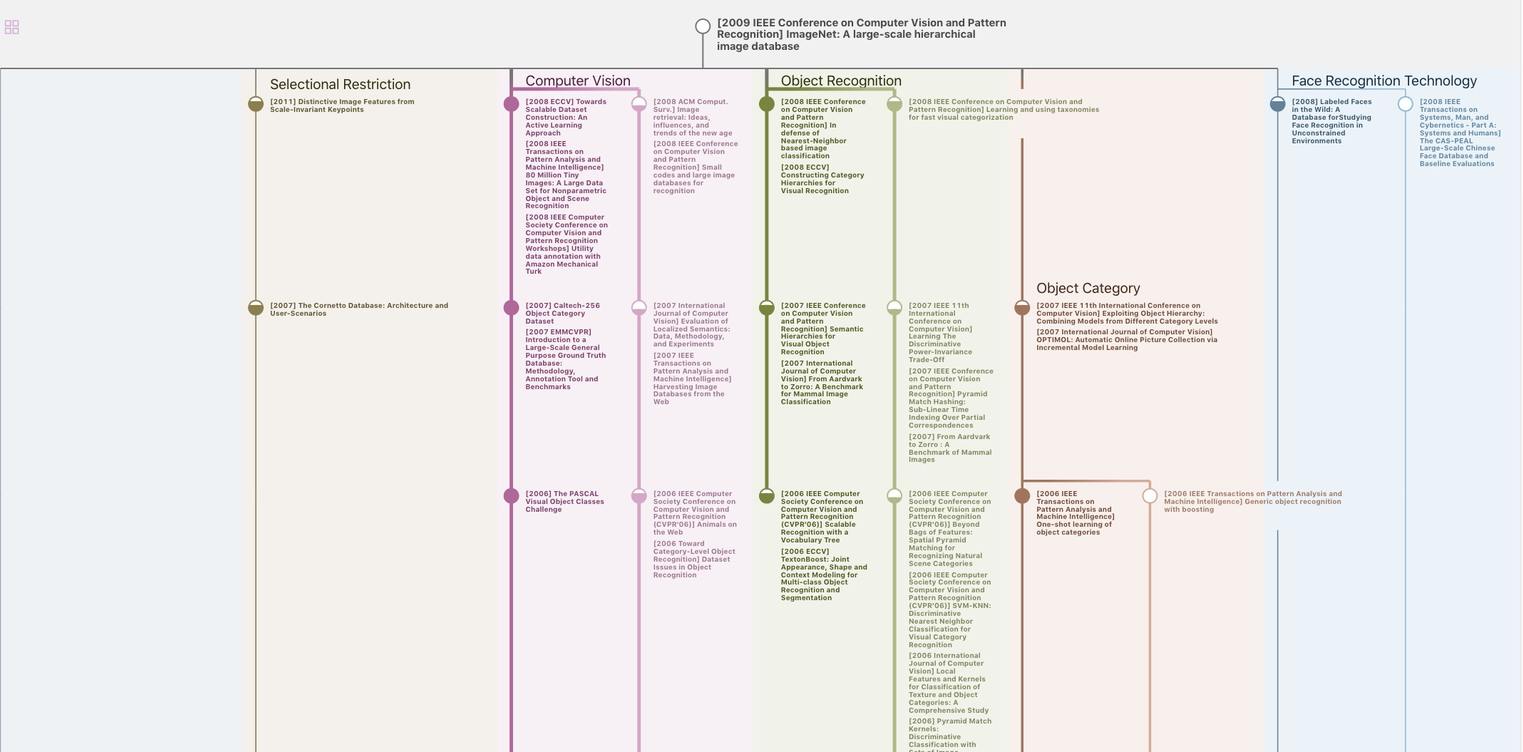
生成溯源树,研究论文发展脉络
Chat Paper
正在生成论文摘要