Domain-specific feature elimination: multi-source domain adaptation for image classification
FRONTIERS OF COMPUTER SCIENCE(2022)
摘要
Multi-source domain adaptation utilizes multiple source domains to learn the knowledge and transfers it to an unlabeled target domain. To address the problem, most of the existing methods aim to minimize the domain shift by auxiliary distribution alignment objectives, which reduces the effect of domain-specific features. However, without explicitly modeling the domain-specific features, it is not easy to guarantee that the domain-invariant representation extracted from input domains contains domain-specific information as few as possible. In this work, we present a different perspective on MSDA, which employs the idea of feature elimination to reduce the influence of domain-specific features. We design two different ways to extract domain-specific features and total features and construct the domain-invariant representations by eliminating the domain-specific features from total features. The experimental results on different domain adaptation datasets demonstrate the effectiveness of our method and the generalization ability of our model.
更多查看译文
关键词
multi-source domain adaptation,generalization,feature elimination
AI 理解论文
溯源树
样例
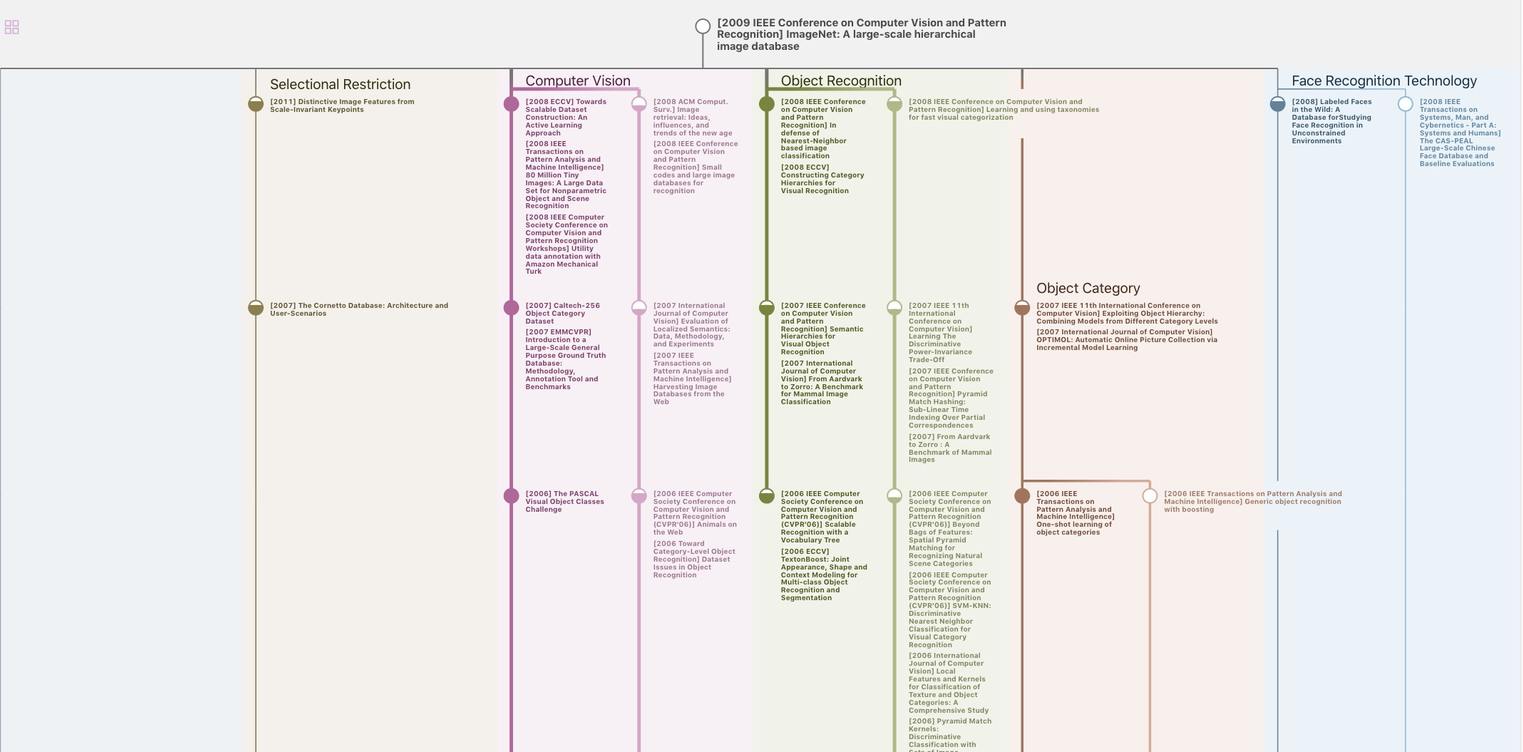
生成溯源树,研究论文发展脉络
Chat Paper
正在生成论文摘要