Real-Time On-Demand Design of Circuit-Analog Plasmonic Stack Metamaterials by Divide-and-Conquer Deep Learning
LASER & PHOTONICS REVIEWS(2022)
摘要
The design of plasmonic stack metamaterials (PSMs) is critical due to their promising potentials in the fields of optical absorbers, sensors, and thermal irradiation. Compared with the classical circuit-based optimization, the design by deep learning (DL) has attracted greater attention, since it is not essential to obtain their equivalent circuit parameters. Currently, a DL model for their higher-precision design, especially with complicated spectral features, is still quite in demand. Here, a divide-and-conquer DL model based on a bidirectional artificial neural network is proposed. As proof-of-concept examples, the PSMs consisting of two metal/dielectric/metal/dielectric subwavelength stacks are adopted to demonstrate the validity of the paradigm. It demonstrates a significant prediction error reduction of 37.5% with the 47.8% decrease of training parameters than the conventional method in the forward network, which supports a powerful inverse design from spectra to PSM structures. Furthermore, a flexible tool based on the free customer definition, which facilitates the real-time design of PSMs with various circuit-analog functions, is developed. The fabrication and measurement experiments verify the design performance of the method. The study enhances the precision and convenience of on-demand circuit-analog PSMs and will provide a guide for fast high-performance inverse design of many other metamaterials.
更多查看译文
关键词
deep learning,inverse design,metamaterials,neural networks
AI 理解论文
溯源树
样例
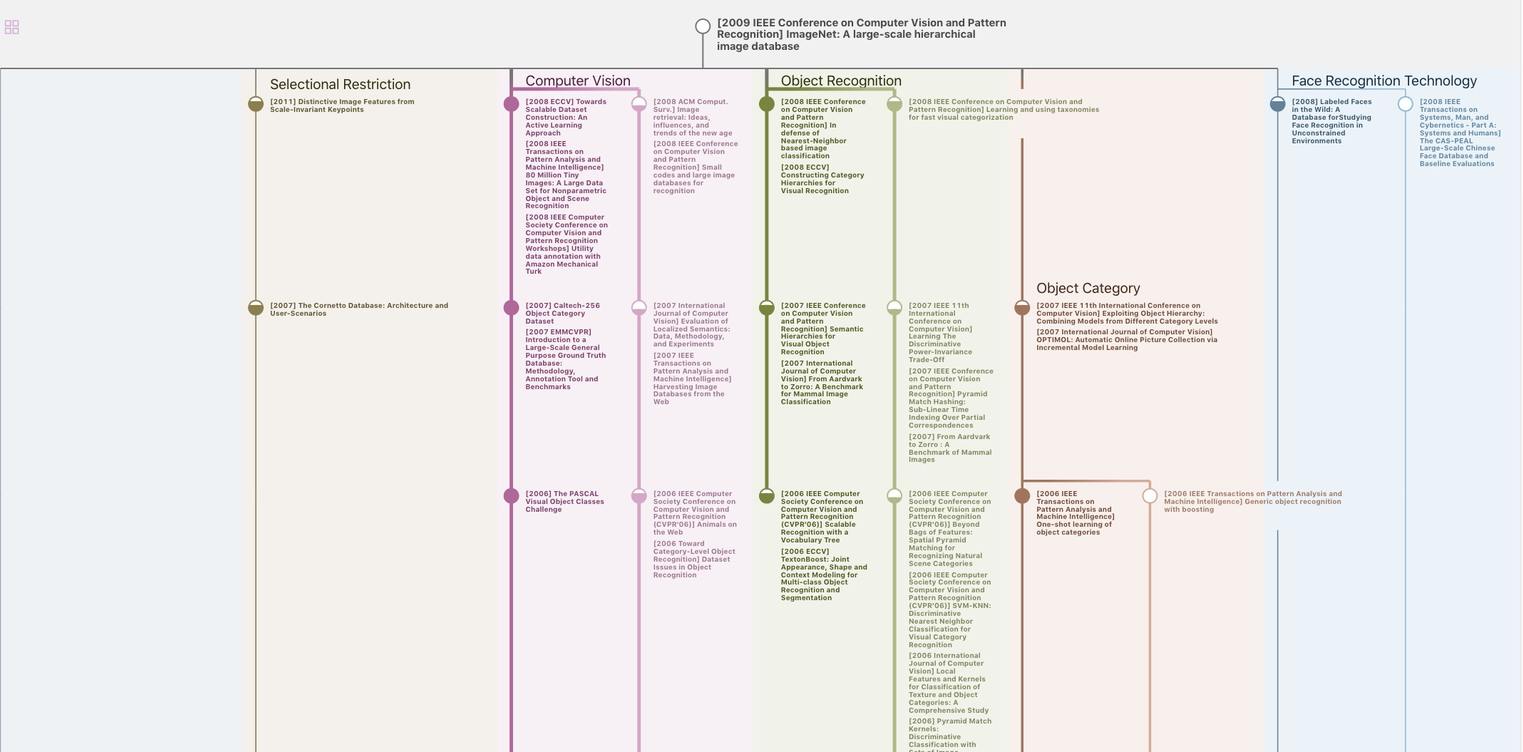
生成溯源树,研究论文发展脉络
Chat Paper
正在生成论文摘要