Efficient Sampling of Bernoulli-Gaussian-Mixtures for Sparse Signal Restoration
IEEE TRANSACTIONS ON SIGNAL PROCESSING(2022)
摘要
This paper introduces a new family of prior models called Bernoulli-Gaussian-Mixtures (BGM), with a view to efficiently address sparse linear inverse problems or sparse linear regression, in the Bayesian framework. The BGM family is based on continuous Location and Scale Mixtures of Gaussians (LSMG), which includes a wide range of symmetric and asymmetric heavy-tailed probability distributions. Particular attention is paid to the decomposition of probability laws as Gaussian mixtures, from which we derive a Partially Collapsed Gibbs Sampler (PCGS) for the BGM, in a systematic way. PCGS is shown to be more efficient than the standard Gibbs sampler, both in terms of number of iterations and CPU time. Moreover, special attention is paid to BGM involving a density defined over a real half-line. An asymptotically exact LSMG approximation is introduced, which allows us to expand the applicability of PCGS to cases such as BGM models with a non-negative support.
更多查看译文
关键词
Sparsity, MCMC, partially collapsed sampling, continuous Gaussian mixtures, non-negativity
AI 理解论文
溯源树
样例
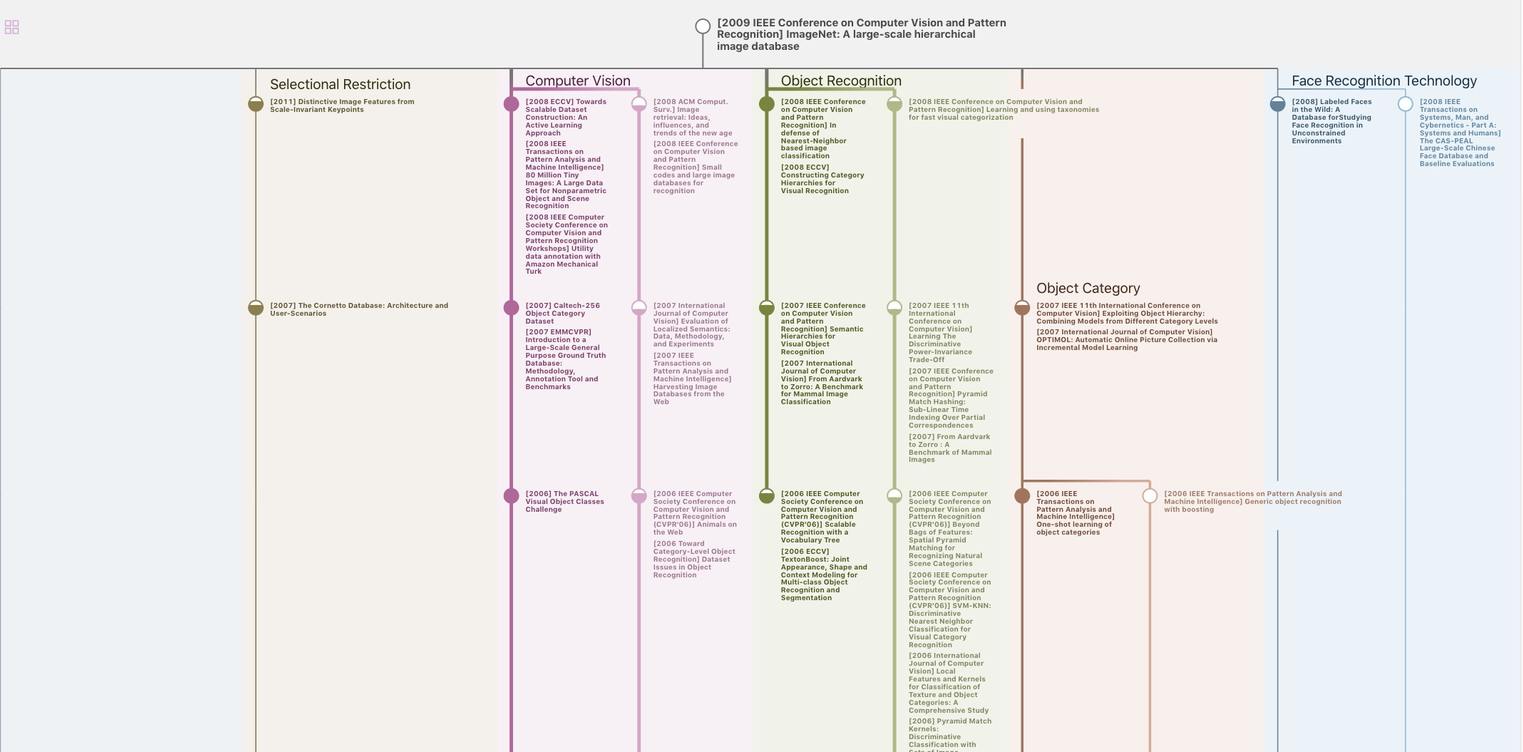
生成溯源树,研究论文发展脉络
Chat Paper
正在生成论文摘要