Fast Incomplete Multi-View Clustering With View-Independent Anchors
IEEE TRANSACTIONS ON NEURAL NETWORKS AND LEARNING SYSTEMS(2022)
摘要
Multi-view clustering (MVC) methods aim to exploit consistent and complementary information among each view and achieve encouraging performance improvement than single-view counterparts. In practical applications, it is common to obtain instances with partially available information, raising researches of incomplete multi-view clustering (IMC) issues. Recently, several fast IMC methods have been proposed to process the large-scale partial data. Though with considerable acceleration, these methods seek view-shared anchors and ignore specific information among single views. To tackle the above issue, we propose a fast IMC with view-independent anchors (FIMVC-VIA) method in this article. Specifically, we learn individual anchors based on the diversity of distribution among each incomplete view and construct a unified anchor graph following the principle of consistent clustering structure. By constructing an anchor graph instead of pairwise full graph, the time and space complexities of our proposed FIMVC-VIA are proven to be linearly related to the number of samples, which can efficiently solve the large-scale task. The experiment performed on benchmarks with different missing rate illustrates the improvement in complexity and effectiveness of our method compared with other IMC methods. Our code is publicly available at https://github.com/Tracesource/ FIMVC-VIA.
更多查看译文
关键词
Anchor graph,incomplete multi-view clustering (IMC),large-scale clustering,multi-view clustering (MVC)
AI 理解论文
溯源树
样例
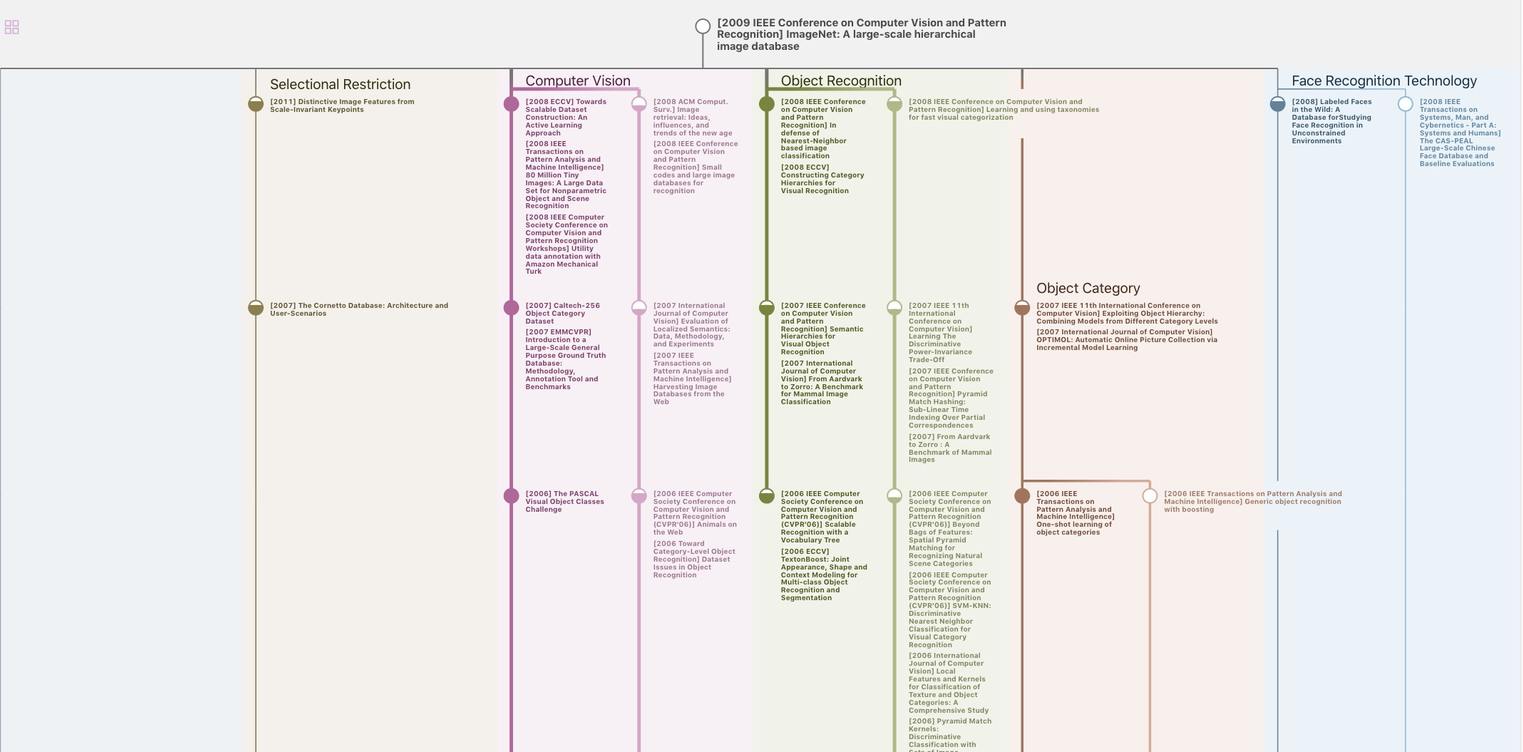
生成溯源树,研究论文发展脉络
Chat Paper
正在生成论文摘要