One Step Ahead Energy Load Forecasting: A Multi-model approach utilizing Machine and Deep Learning
2022 57TH INTERNATIONAL UNIVERSITIES POWER ENGINEERING CONFERENCE (UPEC 2022): BIG DATA AND SMART GRIDS(2022)
摘要
Emerging Energy Load Forecasting (ELF) methodologies assist Distribution System Operators (DSOs) and Aggregators. Energy imbalance among consumption and generation could also be managed with high prediction accuracy, as well as smart grid applications, like Demand Response (DR) events. This study aims to test several algorithms as a solution for ELF. The proposed methodology utilizes machine/deep learning models for time-series forecasting in the domain of energy consumption. Via result comparison it has been illustrated that Neural Networks (NNs), both artificial NNs such as Multilayer Perceptron (MLP) and Long Short-Term Memory (LSTM) recurrent NNs with Extreme Gradient Boosting (XGBoost) were the more accurate ones among other models, showcasing Mean Absolute Error (MAE), R-squared ( R-2), Root Mean Squared Error (RMSE) and Coefficient Variation of Root Mean Squared Error (CVRMSE) values equal to 1.281, 0.98, 2.238 and 0.147, respectively.
更多查看译文
关键词
energy load forecasting,machine learning,deep learning,time series forecasting,sliding window,regression
AI 理解论文
溯源树
样例
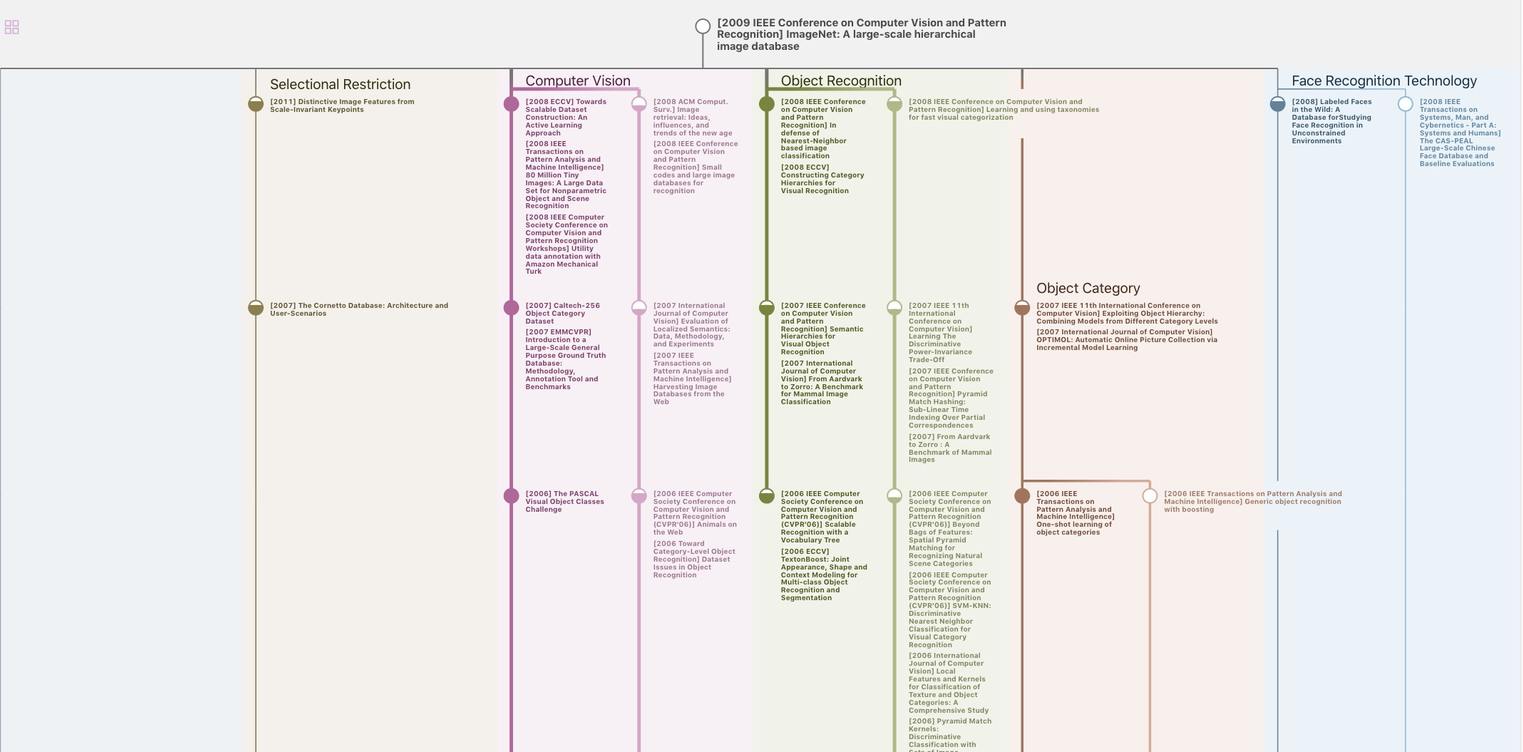
生成溯源树,研究论文发展脉络
Chat Paper
正在生成论文摘要