A relation-aware heterogeneous graph convolutional network for relationship prediction
Information Sciences(2023)
摘要
Most real-world networks are heterogeneous and consist of different types of nodes and edges. Relationships (edges) between nodes of different types carry different semantics, hence relationship prediction in heterogeneous networks aims to predict the existence of a relation between nodes of different types. In this paper, we propose a relation-aware Heterogeneous Graph Convolutional Network architecture for temporal heterogeneous network relationship prediction. In particular, we first present a continuous-time temporal heterogeneous neighbour generation algorithm to capture the continuous-time interactions in the context of a specific node type. The algorithm collects temporal heterogeneous neighbours with K-hops of a node at a recent temporal distance. Moreover, it adds a decaying exponential to ensure that the fewer hops and the closer temporal distances, the more nodes are sampled. Thus, it can capture the evolutionary pattern of interactions in the recent past and collect strongly correlated heterogeneous neighbours for each node. Then the proposed relation-aware Heterogeneous Graph Convolutional Network is used to learn the most relevant relationship to the target relationship to model the heterogeneous graph neural network for predicting target relationships. Our experimental results on three real-world temporal heterogeneous networks indicate significant advances in prediction accuracy and efficiency compared to the state-of-art approaches.
更多查看译文
关键词
Relationship prediction,Temporal heterogeneous networks,Relation-aware heterogeneous graph convolutional network,Network embedding
AI 理解论文
溯源树
样例
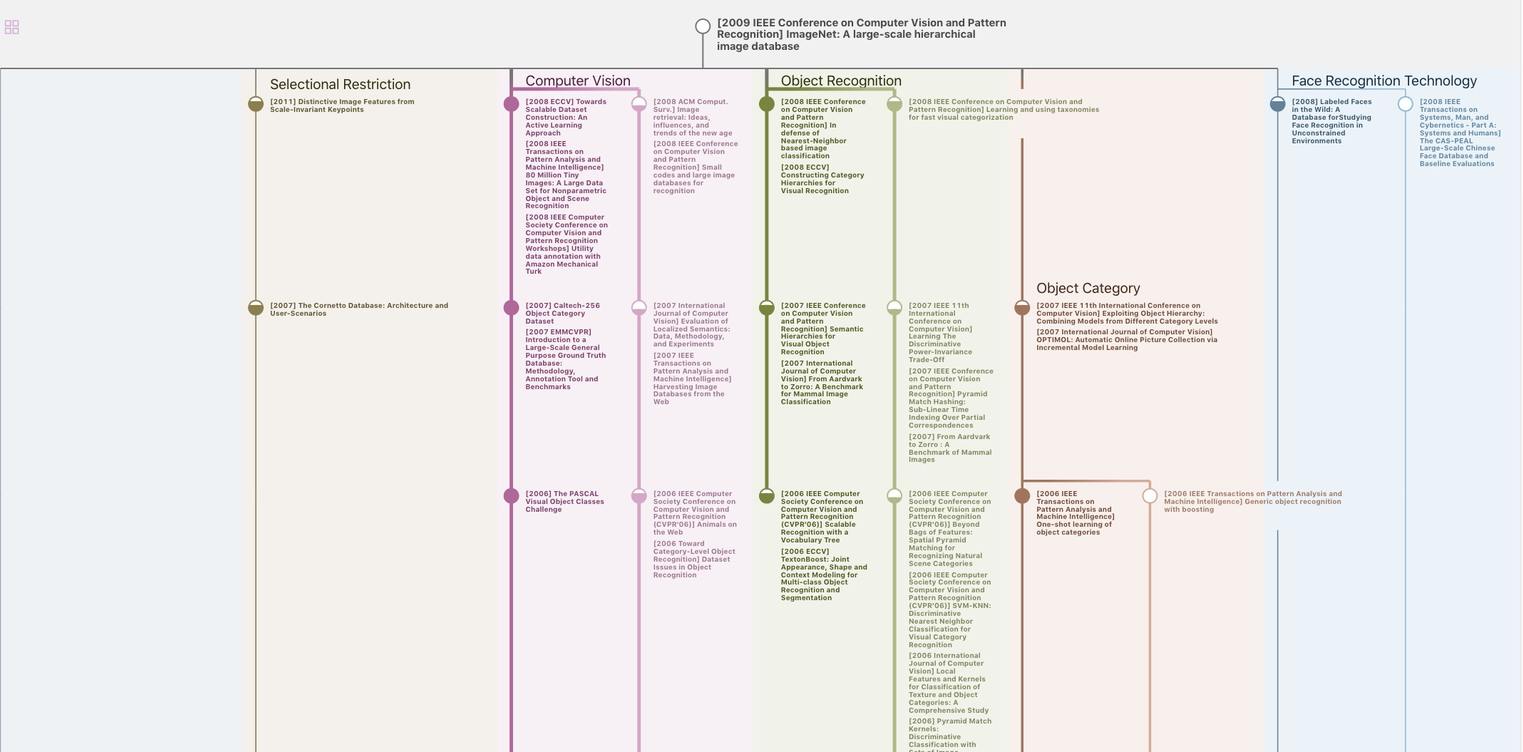
生成溯源树,研究论文发展脉络
Chat Paper
正在生成论文摘要