Robust Variable Selection with Optimality Guarantees for High-Dimensional Logistic Regression
STATS(2021)
摘要
High-dimensional classification studies have become widespread across various domains. The large dimensionality, coupled with the possible presence of data contamination, motivates the use of robust, sparse estimation methods to improve model interpretability and ensure the majority of observations agree with the underlying parametric model. In this study, we propose a robust and sparse estimator for logistic regression models, which simultaneously tackles the presence of outliers and/or irrelevant features. Specifically, we propose the use of L-0-constraints and mixed-integer conic programming techniques to solve the underlying double combinatorial problem in a framework that allows one to pursue optimality guarantees. We use our proposal to investigate the main drivers of honey bee (Apis mellifera) loss through the annual winter loss survey data collected by the Pennsylvania State Beekeepers Association. Previous studies mainly focused on predictive performance, however our approach produces a more interpretable classification model and provides evidence for several outlying observations within the survey data. We compare our proposal with existing heuristic methods and non-robust procedures, demonstrating its effectiveness. In addition to the application to honey bee loss, we present a simulation study where our proposal outperforms other methods across most performance measures and settings.
更多查看译文
关键词
classification, logistic slippage model, mixed-integer conic programming, model selection, honey bee loss, outlier detection, robust estimation
AI 理解论文
溯源树
样例
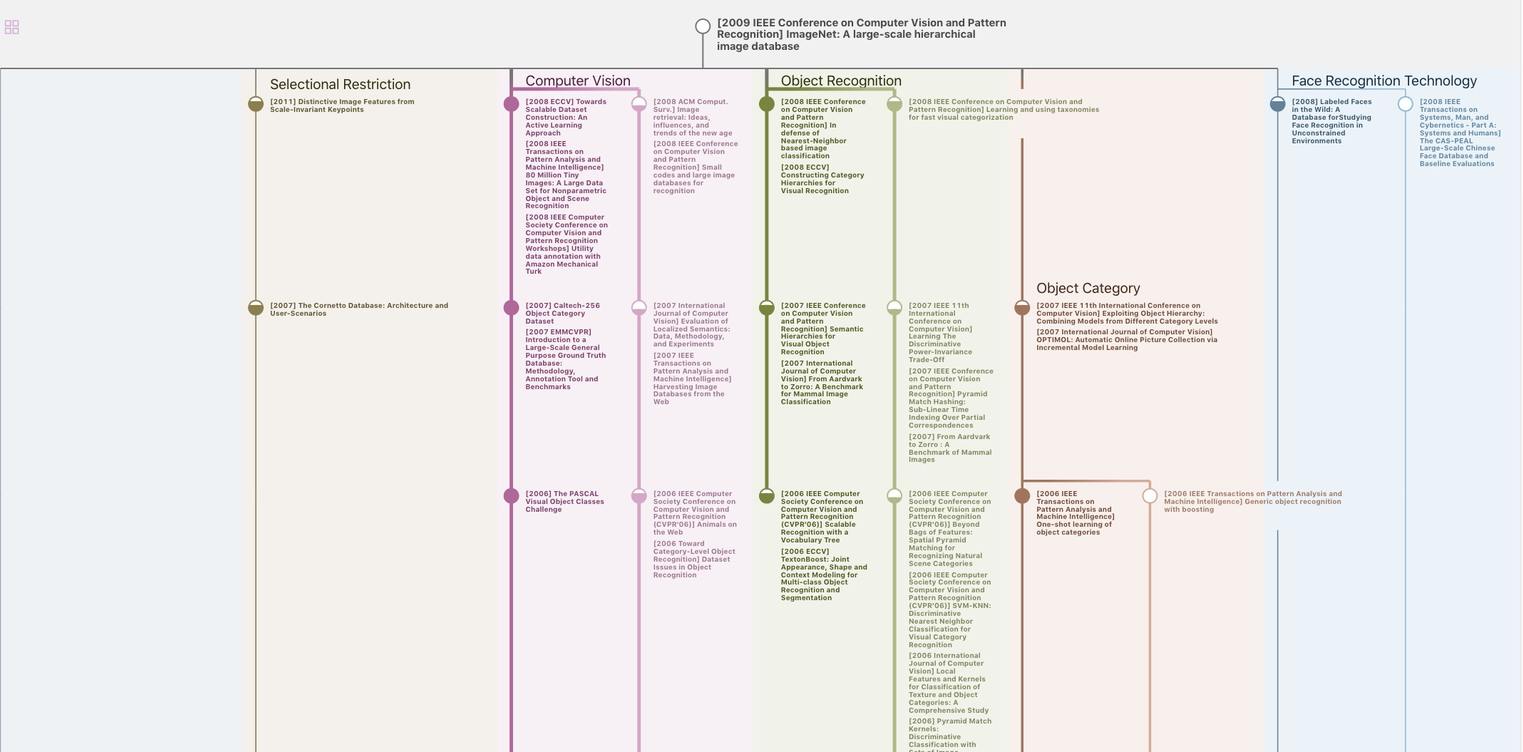
生成溯源树,研究论文发展脉络
Chat Paper
正在生成论文摘要