Brain-inspired computing for in-process melt pool characterization in additive manufacturing
CIRP Journal of Manufacturing Science and Technology(2023)
摘要
Melt pool dynamics represent key information on defect creation in the laser powder bed fusion (LBPF) additive manufacturing process. In-situ sensing of the melt pool is now integrated with LPBF machines. Infrared cameras, optical emission spectroscopies, and photodiodes are utilized for real-time monitoring of thermal process signatures to mitigate defects for improving build quality. The detection of phenomena, including melt pool oscillations, laser modulation, plume formation, and spatter calls upon significant data acquisition rates, and photodiodes alone offer the necessary bandwidth. However, the high data velocity presents a significant computational challenge for training machine learning methods to supervise real-time monitoring. We propose a novel cognitive-based hyperdimensional computing (HDC) for one-pass learning of defects using measurements from photodiodes. We introduce several sampling strategies, namely, no sampling, temporal sampling, spatial sampling, and spatial-temporal sampling for in-situ defect detection. Experimental results based on a real-world case build with overhang layers show that HDC is better at detecting defects than SVM (by 103.150%), KNN (by 104.545%), LDA (by 40.999%), and QDA (by 51.346%) while requires merely 0.273 +/- 0.008 s for training. The proposed HDC is shown to be effective for fast single-pass training and eliminates the necessity of costly retraining for in-process monitoring in various additive manufacturing processes.(c) 2022 CIRP.
更多查看译文
关键词
Additive manufacturing,Quality monitoring,Defect detection,Cognitive computing
AI 理解论文
溯源树
样例
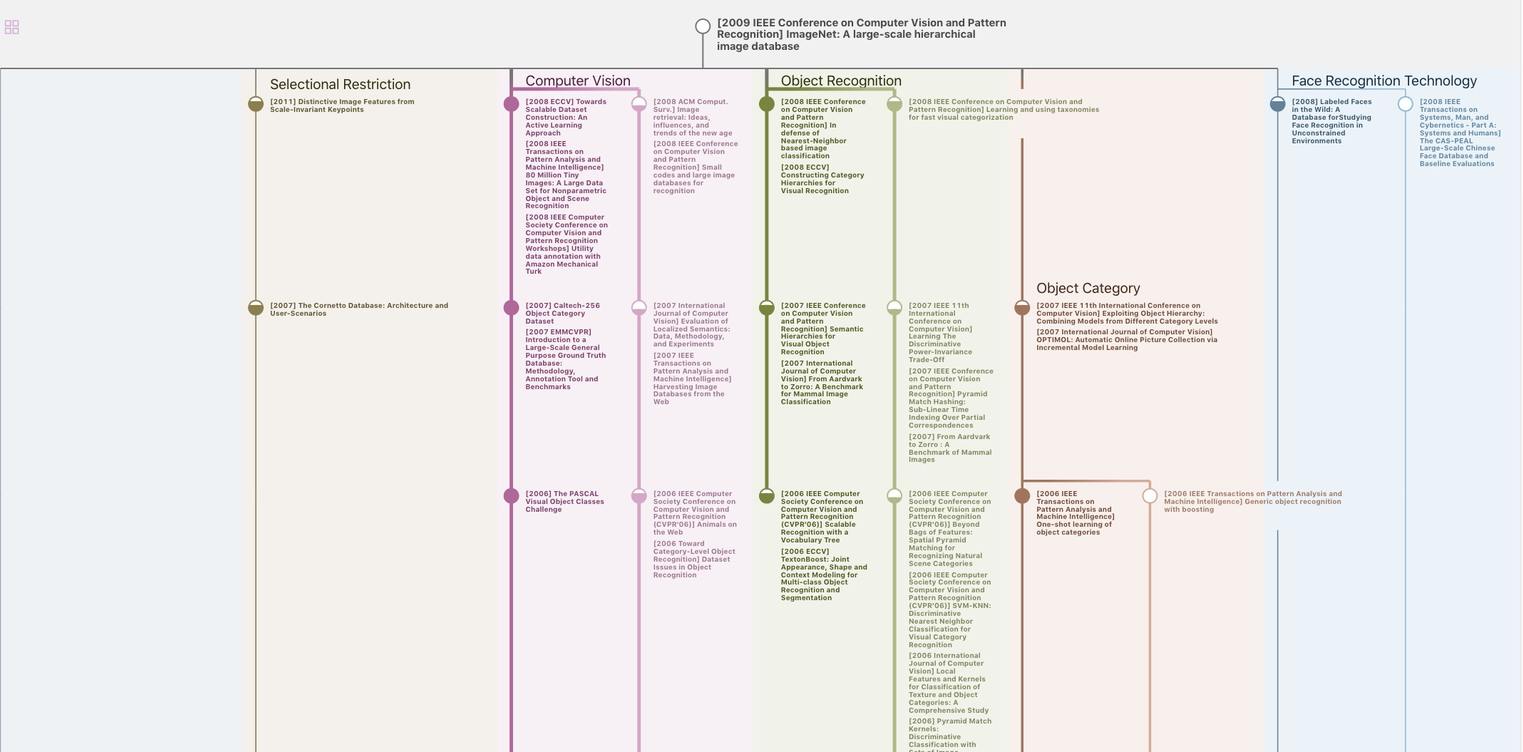
生成溯源树,研究论文发展脉络
Chat Paper
正在生成论文摘要