Real-time evaluation of the dynamic Young's modulus for composite formations based on the drilling parameters using different machine learning algorithms
Frontiers in Earth Science(2022)
摘要
The dynamic Young's modulus (E-dyn) is a parameter needed for optimizing different aspects related to oil well designing. Currently, E-dyn is determined from the knowledge of the formation bulk density, in addition to the shear and compressional velocities, which are not always available. This study introduces three machine learning (ML) models, namely, random forest (RF), adaptive neuro-fuzzy inference system with subtractive clustering (ANFIS-SC), and support vector regression (SVR), for estimation of the E-dyn from only the real-time available drilling parameters. The ML models were learned on 2054 datasets collected from Well-A and then tested and validated on 871 and 2912 datasets from Well-B and Well-C, respectively. The results showed that the three optimized ML models accurately predicted the E-dyn in the three oil wells considered in this study. The optimized SVR model outperformed both the RF and ANFIS-SC models in evaluating the E-dyn in all three wells. For the validation data, the E-dyn was assessed accurately with low average absolute percentage errors of 3.64%, 6.74%, and 1.03% using the optimized RF, ANFIS-SC, and SVR models, respectively.
更多查看译文
关键词
dynamic Young's modulus,drilling parameters,machine learning models,real-time prediction,composite formation
AI 理解论文
溯源树
样例
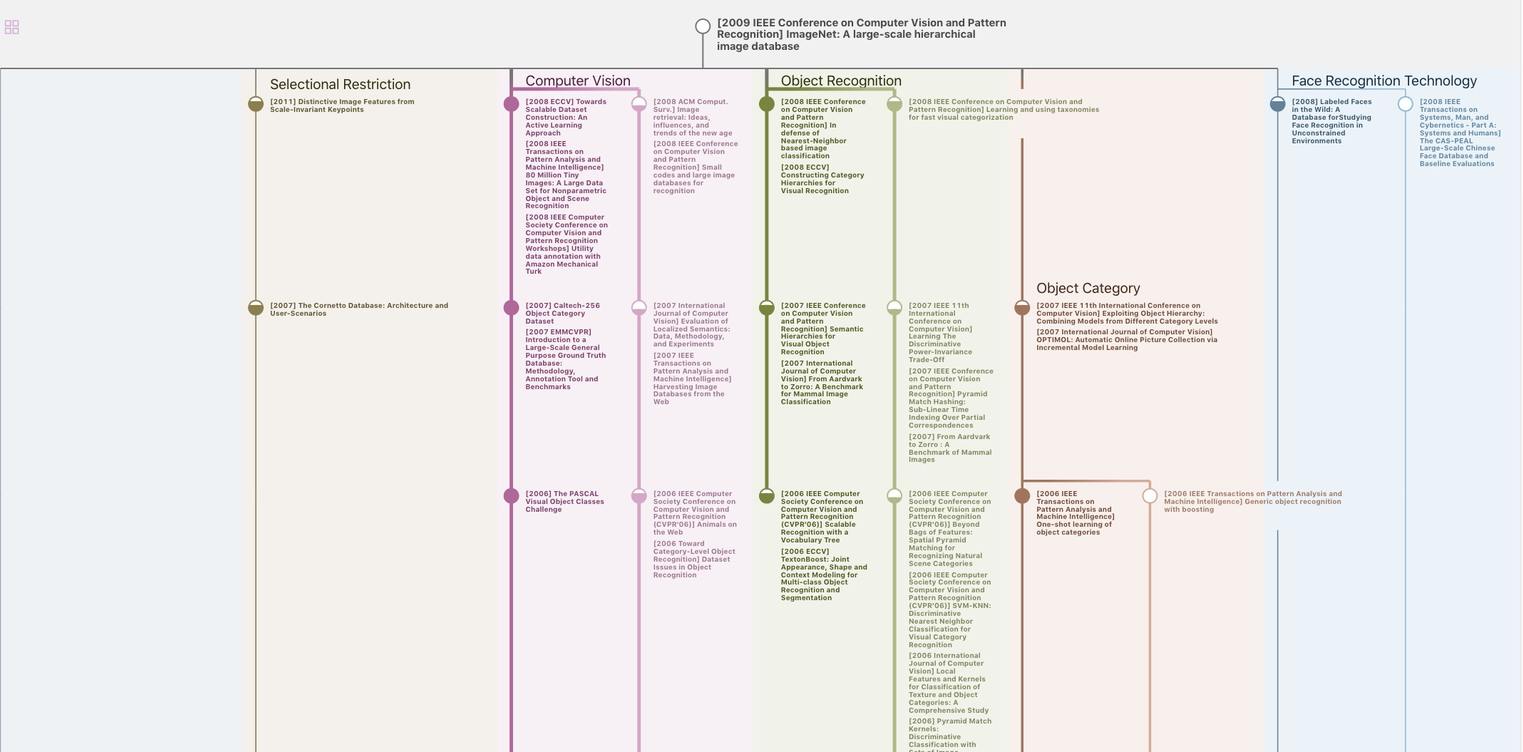
生成溯源树,研究论文发展脉络
Chat Paper
正在生成论文摘要