Combining Computational Screening and Machine Learning to Predict Metal–Organic Framework Adsorbents and Membranes for Removing CH4 or H2 from Air
Membranes(2022)
摘要
Separating and capturing small amounts of CH4 or H2 from a mixture of gases, such as coal mine spent air, at a large scale remains a great challenge. We used large-scale computational screening and machine learning (ML) to simulate and explore the adsorption, diffusion, and permeation properties of 6013 computation-ready experimental metal–organic framework (MOF) adsorbents and MOF membranes (MOFMs) for capturing clean energy gases (CH4 and H2) in air. First, we modeled the relationships between the adsorption and the MOF membrane performance indicators and their characteristic descriptors. Among three ML algorithms, the random forest was found to have the best prediction efficiency for two systems (CH4/(O2 + N2) and H2/(O2 + N2)). Then, the algorithm was further applied to quantitatively analyze the relative importance values of seven MOF descriptors for five performance metrics of the two systems. Furthermore, the 20 best MOFs were also selected. Finally, the commonalities between the high-performance MOFs were analyzed, leading to three types of material design principles: tuned topology, alternative metal nodes, and organic linkers. As a result, this study provides microscopic insights into the capture of trace amounts of CH4 or H2 from air for applications involving coal mine spent air and hydrogen leakage.
更多查看译文
关键词
metal–organic framework,membrane,molecular simulation,computational screening,machine learning
AI 理解论文
溯源树
样例
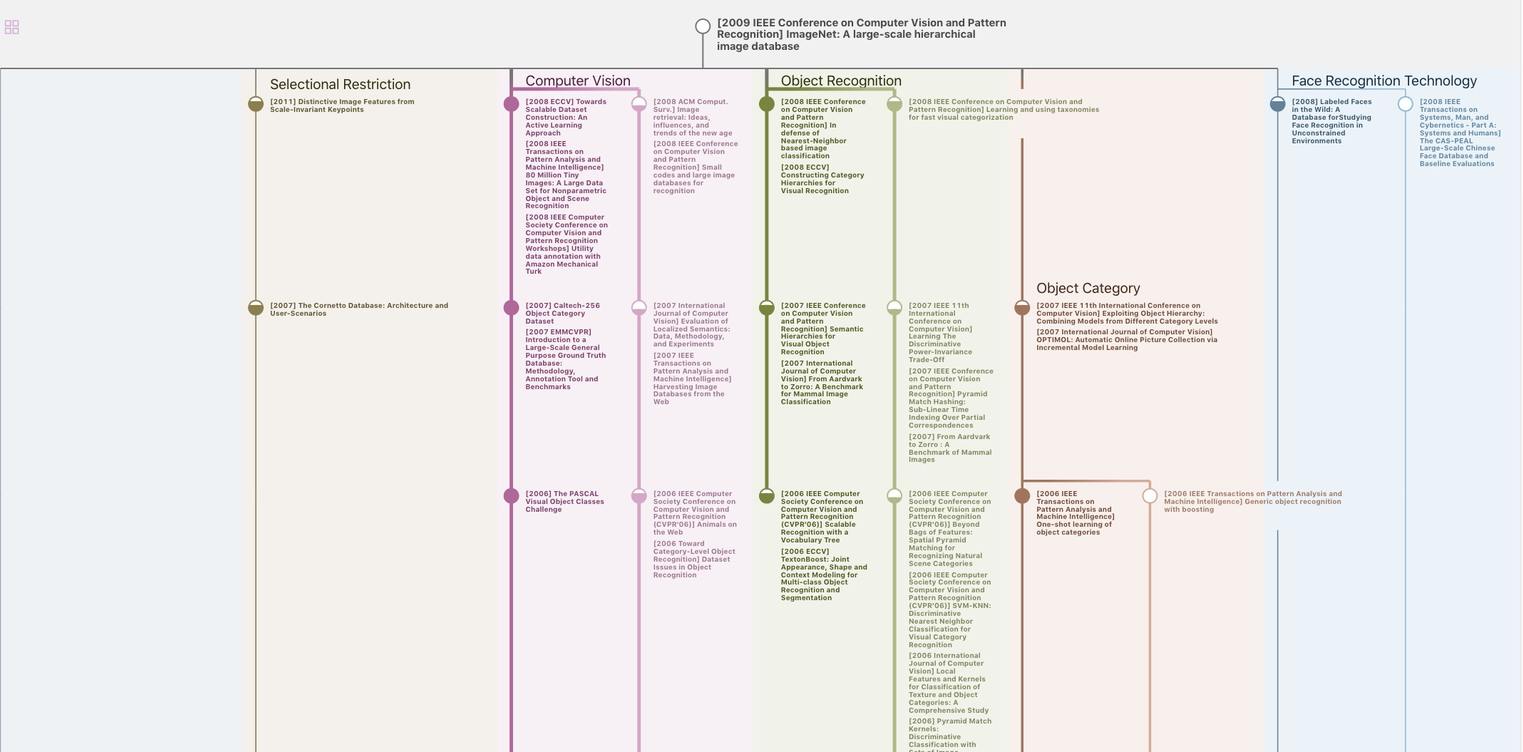
生成溯源树,研究论文发展脉络
Chat Paper
正在生成论文摘要