ResNet-LSTM for Real-Time PM2.5 and PM₁₀ Estimation Using Sequential Smartphone Images
IEEE Access(2020)
摘要
Attempts have been made to estimate PM2.5 and PM10 values from smartphone images, given that deploying highly accurate air pollution monitors throughout a city is a highly expensive undertaking. Departing from previous machine learning studies which primarily focus on pollutant estimation based on single day-time images, our proposed deep learning model integrates Residual Network (ResNet) with Long Short-Term Memory (LSTM), extracting spatial-temporal features of sequential images taken from smartphones instead for estimating PM2.5 and PM10 values of a particular location at a particular time. Our methodology is as follows: First, we calibrated two small portable air quality sensors using the reference instruments placed in the official air quality monitoring station, located at Central, Hong Kong (HK). Second, we verified experimentally that any PM2.5 and PM10 values obtained via our calibrated sensors remain constant within a radius of 500 meters. Third, 3024 outdoor day-time and night-time images of the same building were taken and labelled with corresponding PM2.5 and PM10 ground truth values obtained via the calibrated sensors. Fourth, the proposed ResNet-LSTM was constructed and extended by incorporating meteorological information and one short path. Results have shown that, as compared to the best baselines, ResNet-LSTM has achieved 6.56% and 6.74% reduction in MAE and SMAPE for PM2.5 estimation, and 13.25% and 11.03% reduction in MAE and SMAPE for PM10 estimation, respectively. Further, after incorporating domain-specific meteorological features and one short path, Met-ResNet-LSTM-SP has achieved the best performance, with 24.25% and 20.17% reduction in MAE and SMAPE for PM2.5 estimation, and 28.06% and 24.57% reduction in MAE and SMAPE for PM10 estimation, respectively. In future, our deep-learning image-based air pollution estimation study will incorporate sequential images obtained from 24-hr operating traffic surveillance cameras distributed across all parts of the city in HK, to provide full-day and more fine-grained image-based air pollution estimation for the city.
更多查看译文
关键词
Deep learning,PM2.5 and PM₁₀ estimation,ResNet-LSTM,Met-ResNet-LSTM,ResNet-LSTM-SP,Met-ResNet-LSTM-SP
AI 理解论文
溯源树
样例
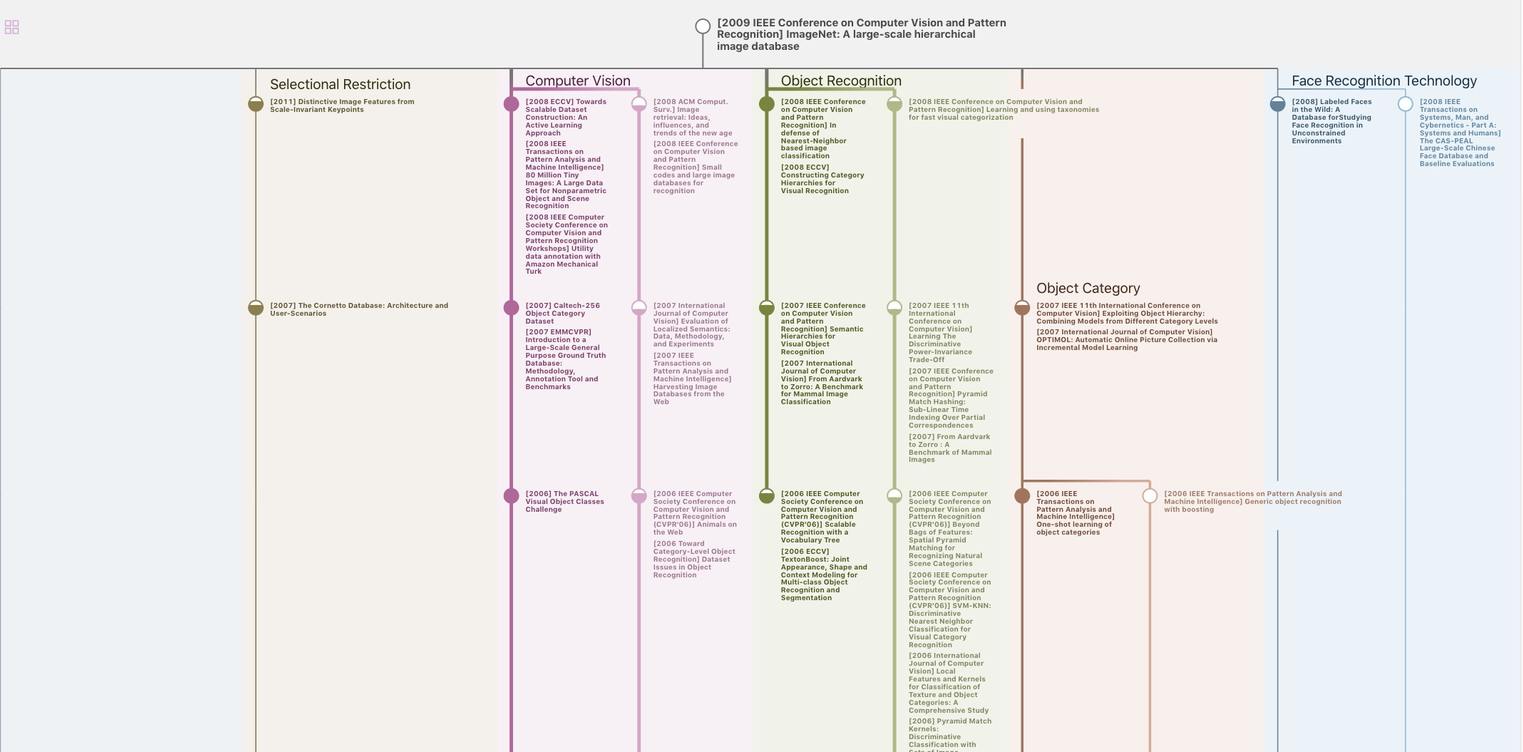
生成溯源树,研究论文发展脉络
Chat Paper
正在生成论文摘要