Evolutionary feature selection on high dimensional data using a search space reduction approach
Engineering Applications of Artificial Intelligence(2023)
摘要
Feature selection is becoming more and more a challenging task due to the increase of the dimensionality of the data. The complexity of the interactions among features and the size of the search space make it unfeasible to find the optimal subset of features. In order to reduce the search space, feature grouping has arisen as an approach that allows to cluster feature according to the shared information about the class. On the other hand, metaheuristic algorithms have proven to achieve sub-optimal solutions within a reasonable time. In this work we propose a Scatter Search (SS) strategy that uses feature grouping to generate an initial population comprised of diverse and high quality solutions. Solutions are then evolved by applying random mechanisms in combination with the feature group structure, with the objective of maintaining during the search a population of good and, at the same time, as diverse as possible solutions. Not only does the proposed strategy provide the best subset of features found but it also reduces the redundancy structure of the data. We test the strategy on high dimensional data from biomedical and text-mining domains. The results are compared with those obtained by other adaptations of SS and other popular strategies. Results show that the proposed strategy can find, on average, the smallest subsets of features without degrading the performance of the classifier.
更多查看译文
关键词
Feature selection,High-dimensional data,Scatter search,Clustering,Feature grouping,Search space reduction
AI 理解论文
溯源树
样例
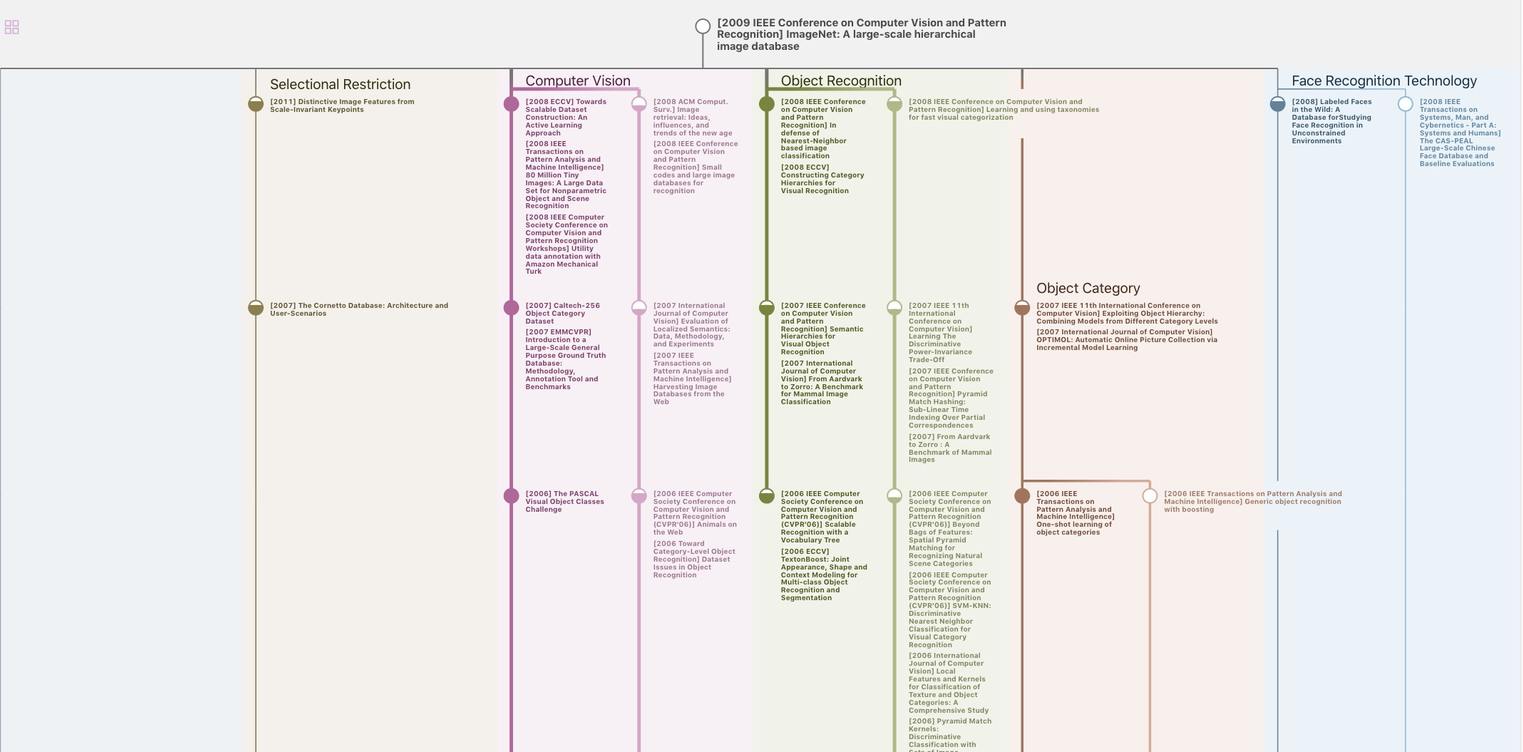
生成溯源树,研究论文发展脉络
Chat Paper
正在生成论文摘要