EARL: An Efficient Approximate HaRdware Framework for AcceLerating Fault Tree Analysis
2022 25th Euromicro Conference on Digital System Design (DSD)(2022)
摘要
This paper proposes an efficient hardware framework that utilizes approximate computing units to mitigate the fault tree (FT) simulation time while considering accuracy and energy. To do so, first, we introduce two hybrid-precision computational units, which can operate in either accurate or approximate modes. Then, we utilize these computational units and an accuracy propagation technique to evaluate the desired accuracy level of each computational part. Finally, EARL, the proposed framework, by taking into account determined accuracy levels, in an offline stage through machine learning technique, predicts the best hardware platform (i.e., CPU, FPGA, or GPU) for executing each benchmark and provides a trade-off among simulation time, accuracy, and energy consumption. To demonstrate EARL's efficiency, we study the case of reliability calculation through fault tree analysis. However, EARL is useful in top-down structured computational problems. Our evaluations show that the proposed framework improves the simulation time and energy consumption of fault tree analysis on average by 78.1%, and 72.6%, respectively, with a negligible accuracy loss.
更多查看译文
关键词
Reliability,Fault Tree Analysis,Approximate Computing,Monte Carlo Simulation
AI 理解论文
溯源树
样例
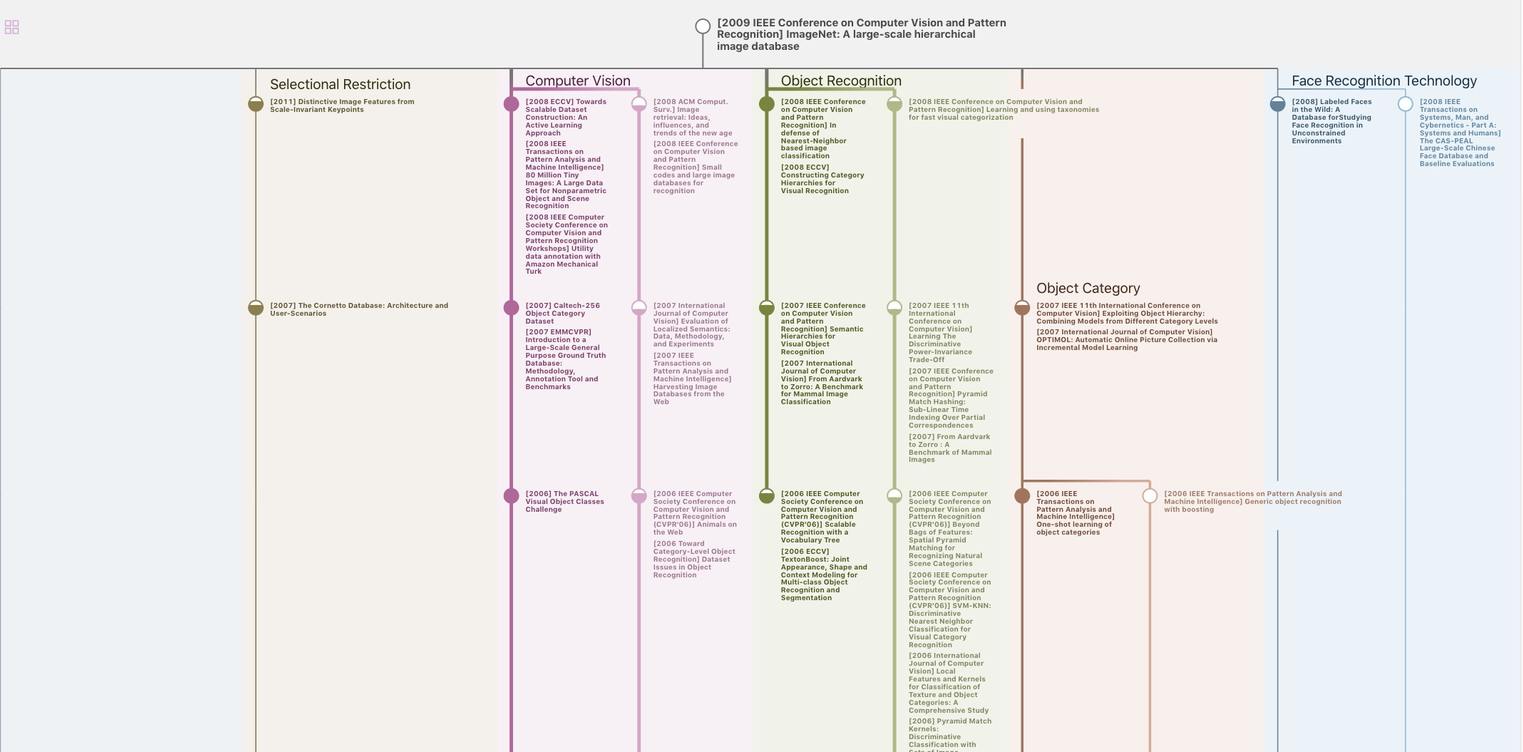
生成溯源树,研究论文发展脉络
Chat Paper
正在生成论文摘要