Enabling Virtual Met Masts for wind energy applications through machine learning-methods
ENERGY AND AI(2023)
摘要
As wind is the basis of all wind energy projects, a precise knowledge about its availability is needed. For an analysis of the site-specific wind conditions, Virtual Meteorological Masts (VMMs) are frequently used. VMMs make use of site calibrated numerical data to provide precise wind estimates during all phases of a wind energy project. Typically, numerical data are used for the long-term correlation that is required for estimating the yield of new wind farm projects. However, VMMs can also be used to fill data gaps or during the operational phase as an additional reference data set to detect degrading sensors. The value of a VMM directly depends on its ability and precision to reproduce site-specific environmental conditions. Commonly, linear regression is used as state of the art to correct reference data to the site-specific conditions. In this study, a framework of 10 different machine-learning methods is tested to investigated the benefit of more advanced methods on two offshore and one onshore site. We find significantly improving correlations between the VMMs and the reference data when using more advanced methods and present the most promising ones. The K-Nearest Neighbors and AdaBoost regressors show the best results in our study, but Multi-Output Mixture of Gaussian Processes is also very promising. The use of more advanced regression models lead to decreased uncertainties; hence those methods should find its way into industrial applications. The recommended regression models can serve as a starting point for the development of end-user applications and services.
更多查看译文
关键词
Virtual Met Mast (VMM),Wind power,Machine learning,Reanalysis data,Site assessment,Weather Research and Forecasting,Model (WRF),Measure-Correlate-Predict (MCP)
AI 理解论文
溯源树
样例
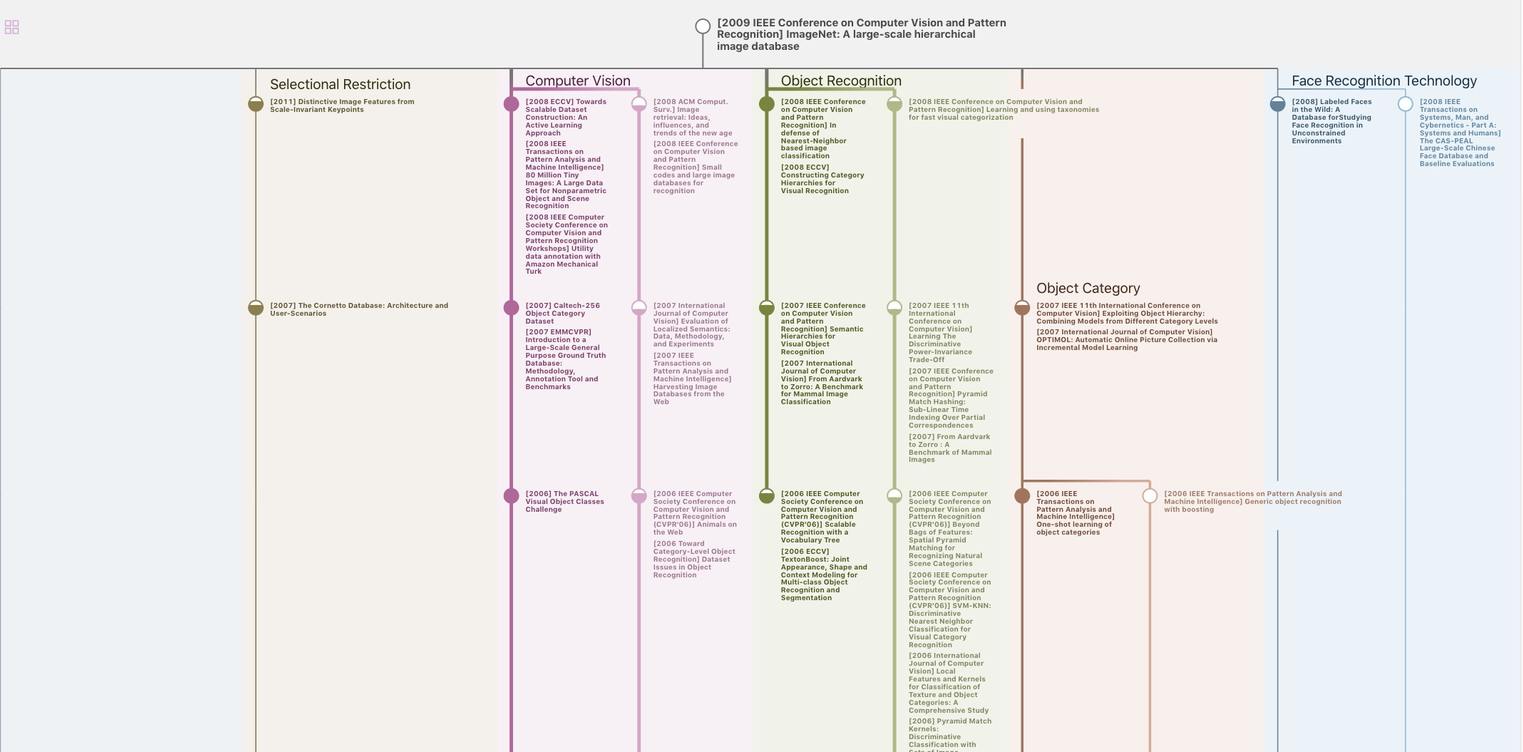
生成溯源树,研究论文发展脉络
Chat Paper
正在生成论文摘要