Federated Learning through Goal Programming: a Computational Study in Cancer Detection
2022 5th International Conference on Signal Processing and Information Security (ICSPIS)(2022)
摘要
In the era of big data, Federated Learning has emerged as a promising way of learning from scattered nodes. In its essence, Federated Learning is a distributed learning methodology allowing model training on a large corpus of decentralized data. Federated Learning can be a sound solution to the data silos problem in healthcare. However, not all Federated Learning approaches are privacy compliant, resulting in data leakages that prevent its usage in healthcare applications. This computational study investigates whether a privacy-compliant Federated Learning framework is suitable for cancer detection. To this end, we tested the Federated Goal Programming framework in the context of cancer detection. Under such a framework, the models are aggregated based on the cross-performance at each node. Data leakage is prevented since training happens only locally, and only the prediction rules are shared. We tested this approach under three publicly available datasets and different ML models. The results show that the Federated Goal Programming framework is suitable for cancer detection.
更多查看译文
关键词
Machine Learning,Federated Learning,Multiple Criteria Decision Making,Medicine,Cancer Detection
AI 理解论文
溯源树
样例
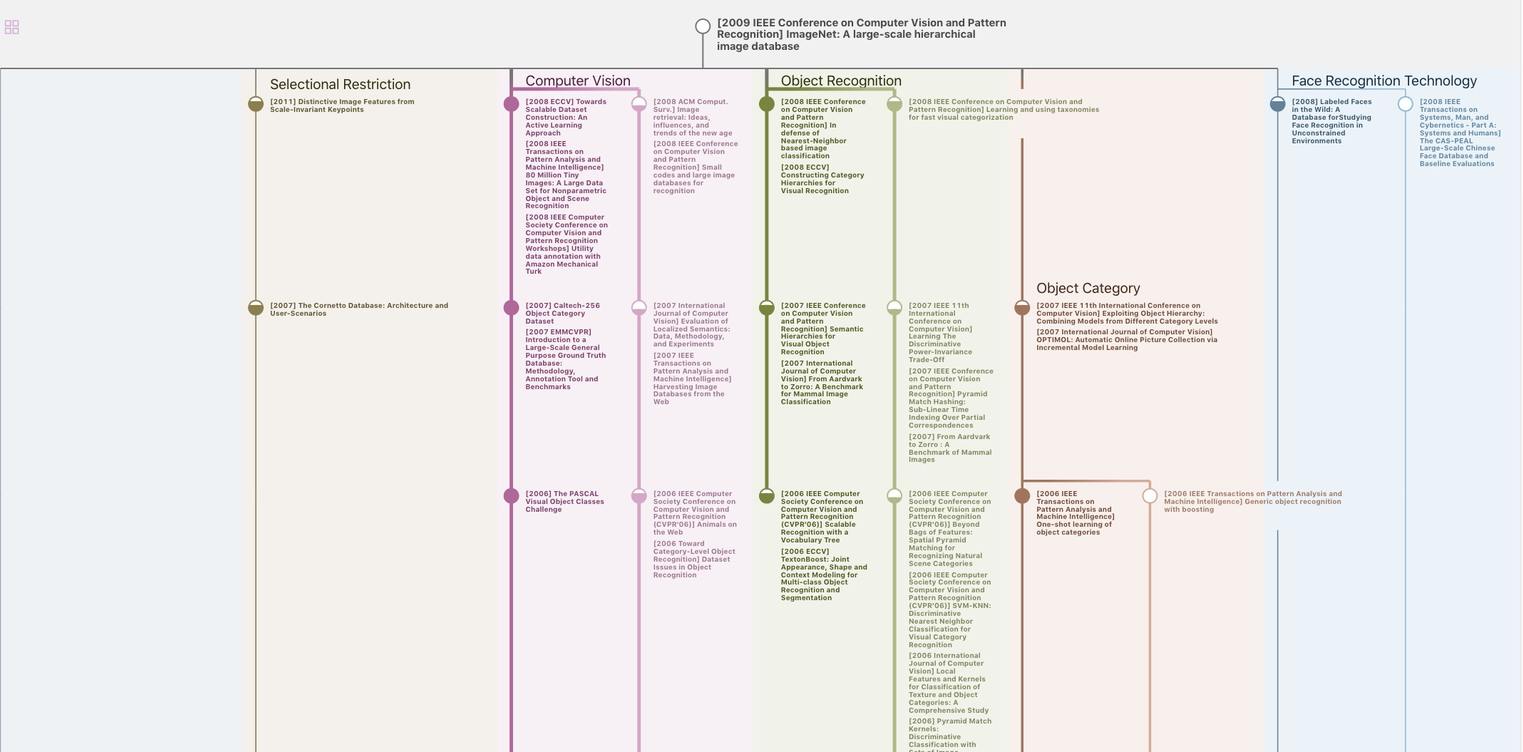
生成溯源树,研究论文发展脉络
Chat Paper
正在生成论文摘要