Poster: SlideCNN: Deep Learning for Auditory Spatial Scenes with Limited Annotated Data
2022 IEEE/ACM 7th Symposium on Edge Computing (SEC)(2022)
摘要
Sound is an important modality to perceive and understand the spatial environment. With the development of digital technology, massive amounts of smart devices in use around the world can collect sound data. Auditory spatial scenes, a spatial environment to understand and distinguish sound, are important to be detected by analyzing sounds collected via those devices. Given limited annotated auditory spatial samples, the current best-performing model can predict an auditory scene with an accuracy of 73%. We propose a novel yet simple Sliding Window based Convolutional Neural Network, SlideCNN, without manually designing features. SlideCNN leverages windowing operation to increase samples for limited annotation problems and improves the prediction accuracy by over 12% compared to the current best-performing models. It can detect real-life indoor and outdoor scenes with a 85% accuracy. The results will enhance practical applications of ML to analyze auditory scenes with limited annotated samples. It will further improve the recognition of environments that may potentially influence the safety of people, especially people with hearing aids and cochlear implant processors.
更多查看译文
AI 理解论文
溯源树
样例
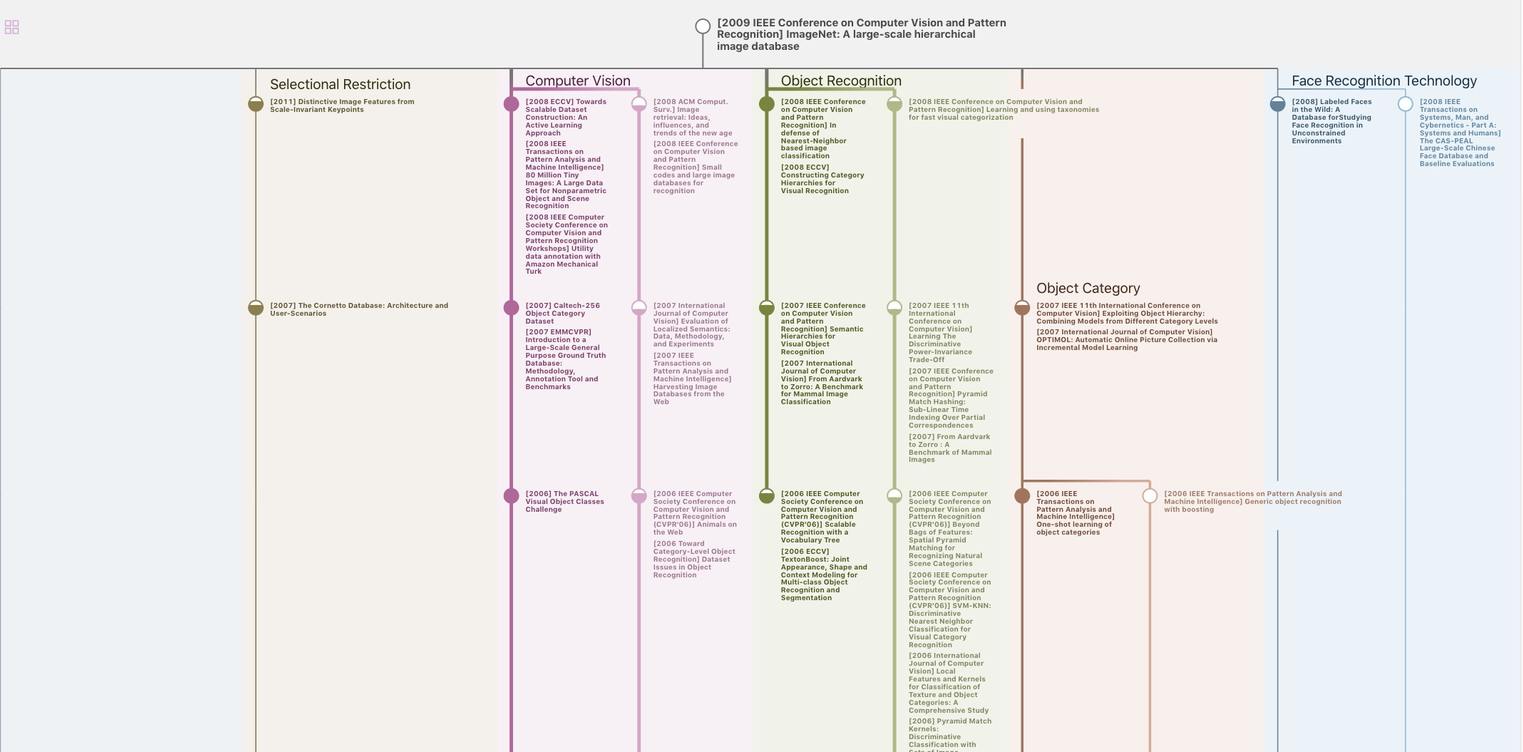
生成溯源树,研究论文发展脉络
Chat Paper
正在生成论文摘要