An NMF-based method for jointly handling mixture nonlinearity and intraclass variability in hyperspectral blind source separation
Digital Signal Processing(2023)
摘要
Considering a set of observed signals that result from mixing (i.e. combining) a set of unknown source signals by means of an unknown function, blind source separation (BSS) and blind mixture identification (BMI) methods aim at estimating these source signals and/or the mixing function. Such methods have been extensively used, e.g. to process hyperspectral data in the field of remote sensing (i.e. Earth observation). However, most of these methods are restricted to the simplest mixing model, namely linear mixtures without the "variability" defined below. Some investigations started to tackle the more complex case of linear-quadratic (LQ), i.e. second-order polynomial, and memoryless mixtures. Some other investigations deal with the so-called intraclass variability phenomenon, i.e. with the case when each source yields a component having a somewhat different "shape" in each observed signal. Such nonlinearity and variability may e.g. appear in remote sensing hyperspectral images, as detailed in this paper. To our knowledge, each BSS/BMI method from the literature related to the nonlinearity and variability issues only addresses one of them. In contrast, we here propose a more powerful, very generic, BSS/BMI approach, that extends standard Nonnegative Matrix Factorization so as to jointly handle LQ mixtures and arbitrary source intraclass variability. This opens the way to a much wider range of BSS/BMI applications than plain linear "variabilityless" mixtures, e.g. to handle the above-defined two issues of remote sensing. Considering the latter application, we built data sets so that they are both realistic and suited to a detailed quantitative performance evaluation. The tests reported in this paper show that our method significantly outperforms several approaches from the literature: the estimation errors are thus decreased by factors up to around 2 and 1.5, respectively for spectra and mixing coefficients.(c) 2022 Elsevier Inc. All rights reserved.
更多查看译文
关键词
Blind source separation,hyperspectral imaging,intraclass source variability,linear -quadratic mixture,nonnegative matrix factorization
AI 理解论文
溯源树
样例
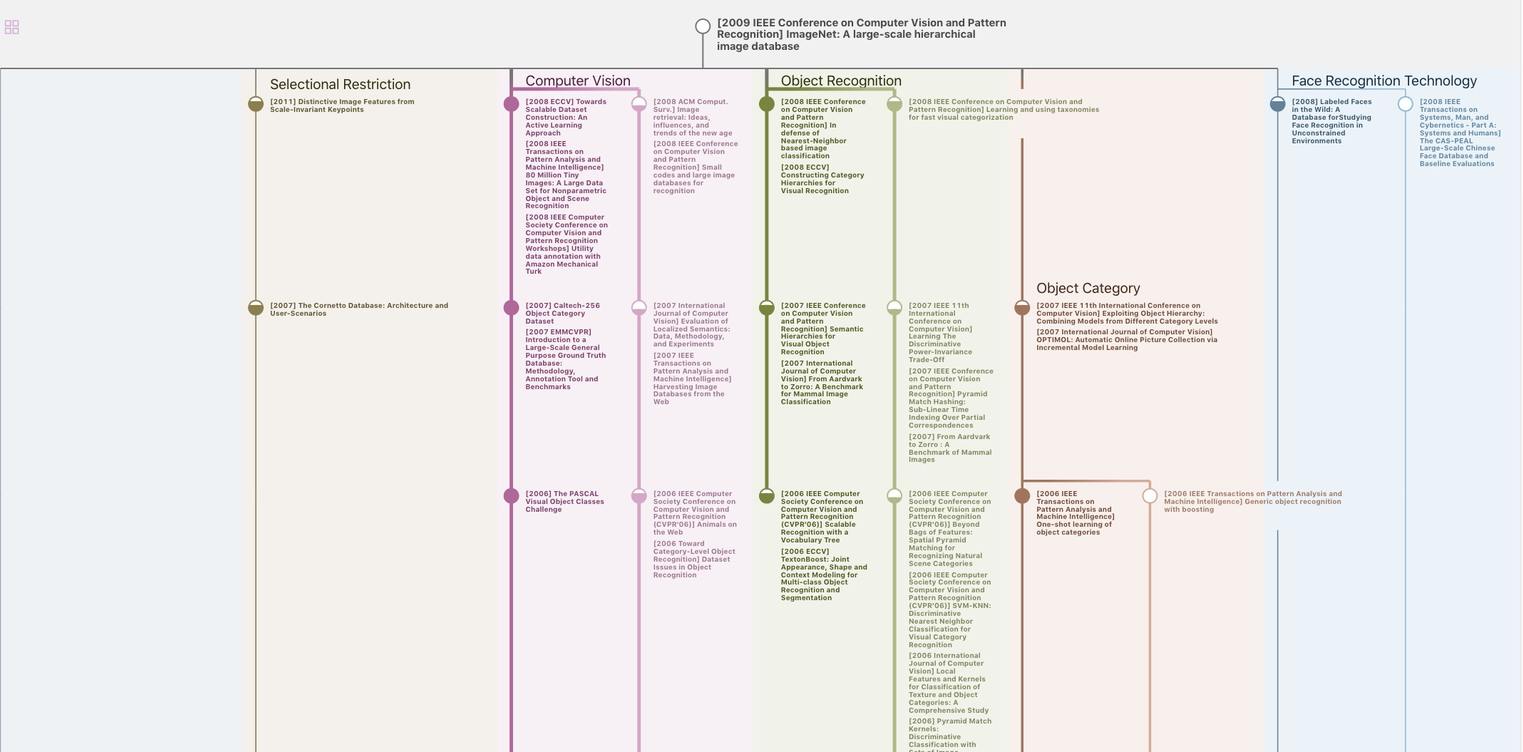
生成溯源树,研究论文发展脉络
Chat Paper
正在生成论文摘要