Distributed Deep Learning in An Edge Computing System
2022 IEEE 19th International Conference on Mobile Ad Hoc and Smart Systems (MASS)(2022)
摘要
In many scenarios (e.g., hurricanes, earthquake, rural areas), edge devices cannot access the cloud, which makes the cloud deep learning (DL) training approach inapplicable. However, an edge device may not be able to train a large-scale DL model due to its resource constraints. Though there are mobile-friendly DL models (e.g., mobilnet, shufflenet), it cannot meet the needs for different Deep Neural Networks (DNNs) and also model compression sacrifices accuracy. Distributed DL training among multiple edge devices is a solution. However, it poses challenges about how to partition a DNN model and assign the partitions among edge devices considering the DNN features and the resource availability, and how to handle edge overload to reduce the overall job time and accuracy loss. To handle the challenges, we propose both heuristic and Reinforcement Learning (RL) based DL job schedulers by leveraging DL job features. Our container-based emulation and real device experiments show that our job schedulers achieve up to 82% improvement on training time and 70% on consumed energy over comparison methods. We also open sourced our source code.
更多查看译文
关键词
n/a
AI 理解论文
溯源树
样例
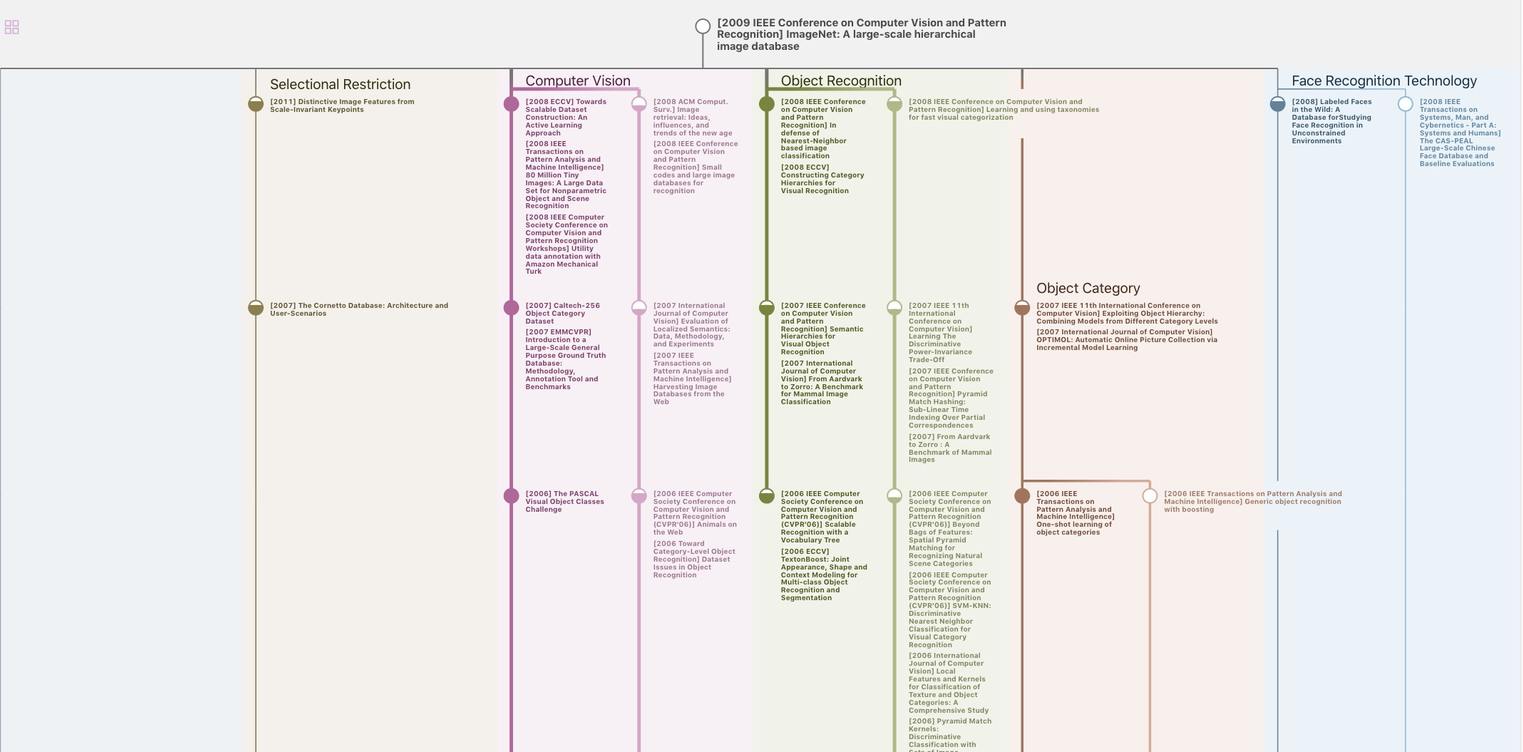
生成溯源树,研究论文发展脉络
Chat Paper
正在生成论文摘要